Adversarially Robust Learning: A Generic Minimax Optimal Learner and Characterization
NeurIPS 2022(2022)
摘要
We present a minimax optimal learner for the problem of learning predictors robust to adversarial examples at test-time. Interestingly, we find that this requires new algorithmic ideas and approaches to adversarially robust learning. In particular, we show, in a strong negative sense, the suboptimality of the robust learner proposed by Montasser, Hanneke, and Srebro (2019) and a broader family of learners we identify as local learners. Our results are enabled by adopting a global perspective, specifically, through a key technical contribution: the global one-inclusion graph, which may be of independent interest, that generalizes the classical one-inclusion graph due to Haussler, Littlestone, and Warmuth (1994). Finally, as a byproduct, we identify a dimension characterizing qualitatively and quantitatively what classes of predictors $\mathcal{H}$ are robustly learnable. This resolves an open problem due to Montasser et al. (2019), and closes a (potentially) infinite gap between the established upper and lower bounds on the sample complexity of adversarially robust learning.
更多查看译文
关键词
adversarially robust PAC learning,sample complexity
AI 理解论文
溯源树
样例
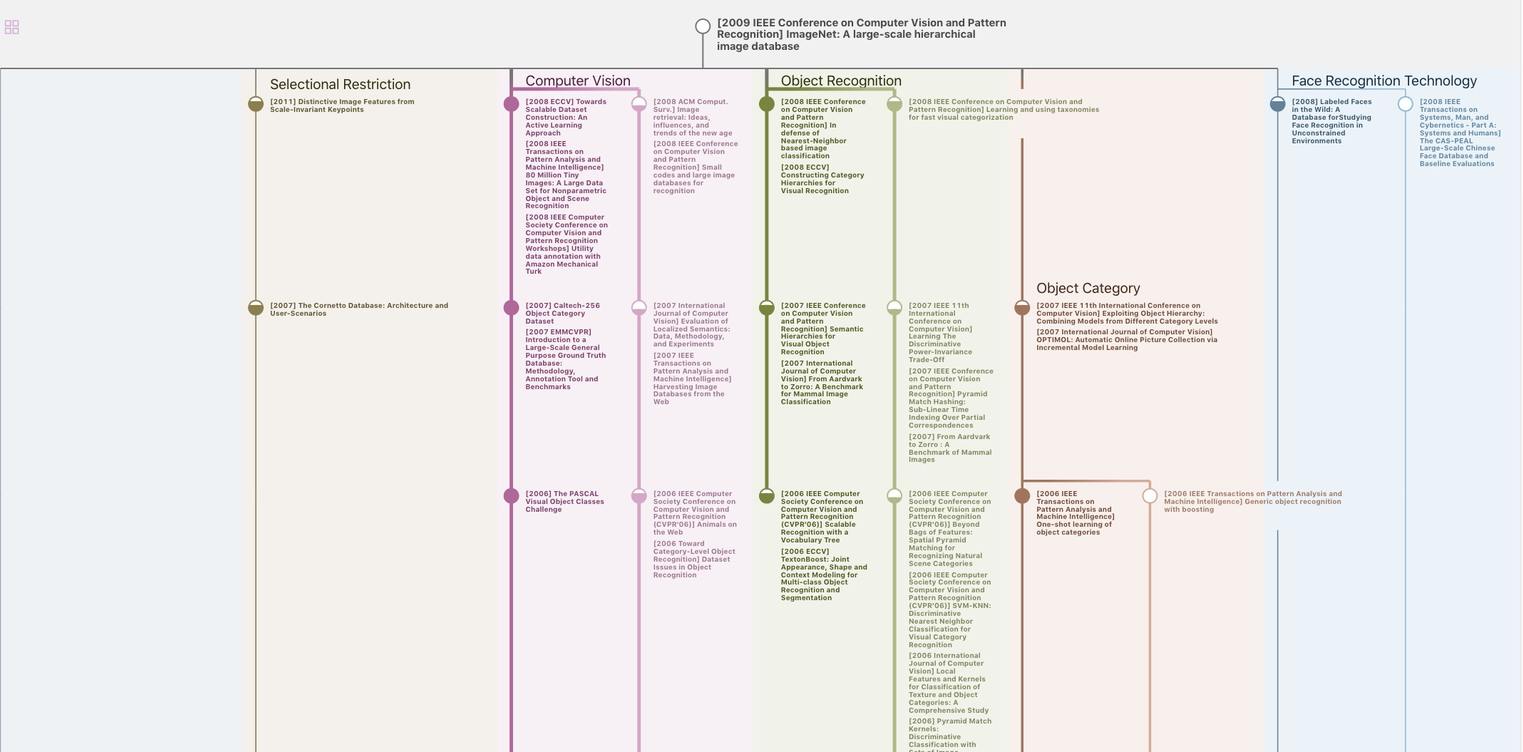
生成溯源树,研究论文发展脉络
Chat Paper
正在生成论文摘要