Domain adaptive multi-task transformer for low-resource machine reading comprehension
Neurocomputing(2022)
摘要
In recent years, low-resource Machine Reading Comprehension (MRC) attracts increasing attention. Due to the difficulty in data collecting, current low-resource MRC approaches often suffer from poor generalizing capability: the model only learns limited task-aware and domain-aware knowledge from a small-scale training dataset. Previous works generally address such deficiency by learning the required knowledge from out-of-domain MRC datasets and in-domain self-supervised datasets. However, such approaches also introduce domain noise and task noise. This paper proposes a Domain Adaptive Multi-Task Transformer (DAMT2) to tackle these noises. For task noise, DAMT2 utilizes a well-designed Multi-Task Transformer (MT2) as the backbone to model the high-level features separately from different tasks. For domain noise, two kinds of domain adaptation approaches are incorporated into MT2 to learn domain-invariant representations. The experimental results show that our method outperforms several baselines on multiple datasets, and especially achieves a new SOTA on the RRC dataset. Moreover, using only 40%-60% training data, our work achieves comparable performance with the classic BERT model.
更多查看译文
关键词
Low-resource machine reading comprehension,Multi-task transformer,Domain adaptation
AI 理解论文
溯源树
样例
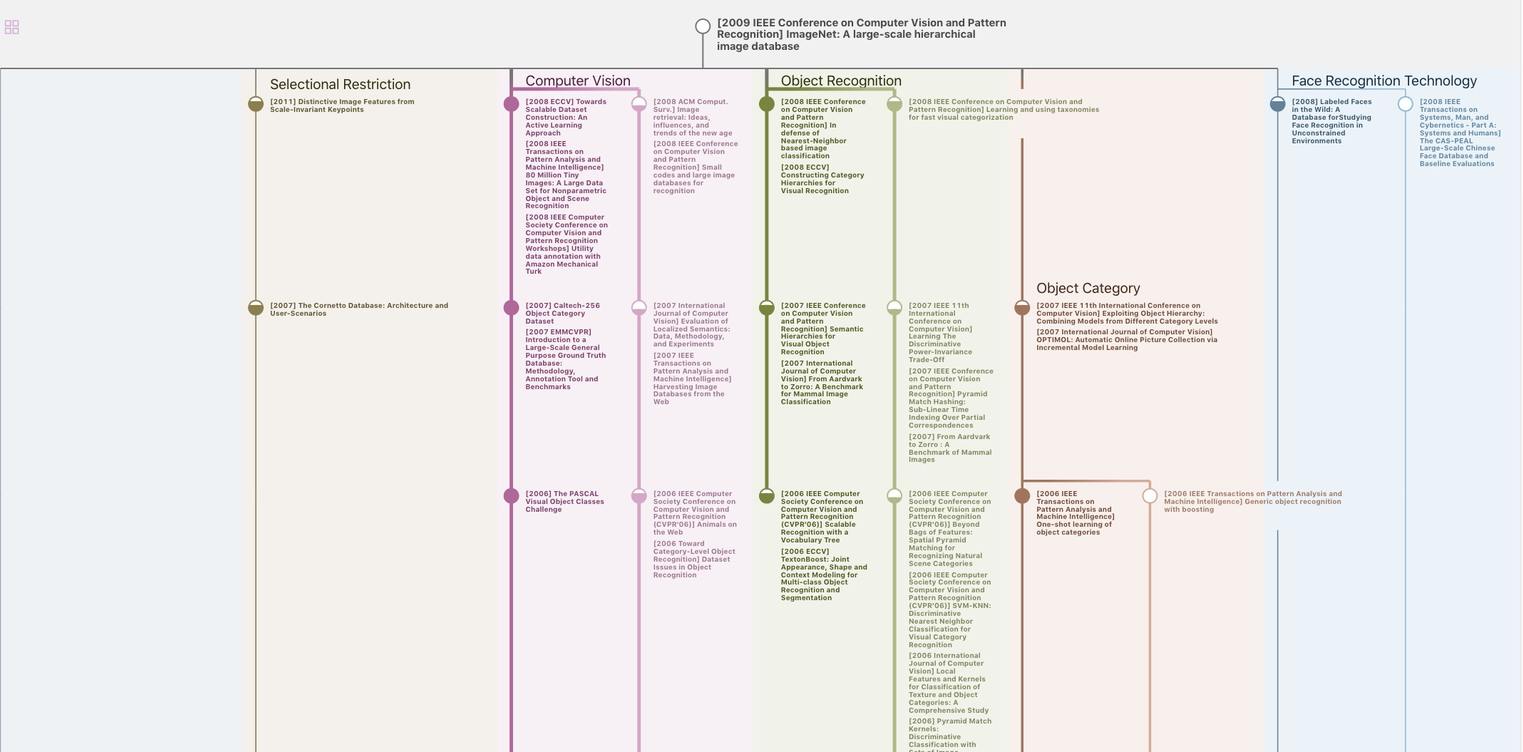
生成溯源树,研究论文发展脉络
Chat Paper
正在生成论文摘要