Vector Set Classification by Signal Subspace Matching
IEEE transactions on information theory(2023)CCF ASCI 3区
Technion Israel Inst Technol | Braude Coll Engn
Abstract
We present a powerful solution to the problem of vector set classification, based on a novel goodness-of-fit metric, referred to as signal subspace matching (SSM). Unlike the existing solutions based on principal component analysis (PCA), this solution is eigendecomposition-free and dimension-selection-free, i.e., it does not require PCA nor the election of the subspace dimension, which is done implicitly. More importantly, it copes effectively with the challenging cases wherein the subspaces characterizing the classes are partially or fully overlapping. The SSM metric matches the subspaces characterizing the vector sets of the test and the classes by minimizing the distance between respective soft-projection matrices constructed from the vector sets. We prove the consistency of the solution for the high signal-to-noise-ratio limit, and also for the large-sample limit, conditioned on the noise being white. Experimental results, demonstrating the superiority of the SSM solution over the existing PCA-based solutions, especially in the challenging cases of overlapping subspaces, are included.
MoreTranslated text
Key words
Measurement,Principal component analysis,Location awareness,Loading,Face recognition,Eigenvalues and eigenfunctions,Matrices,Subspace-based classification,subspace-based learning,signal subspace,latent subspace
求助PDF
上传PDF
View via Publisher
AI Read Science
AI Summary
AI Summary is the key point extracted automatically understanding the full text of the paper, including the background, methods, results, conclusions, icons and other key content, so that you can get the outline of the paper at a glance.
Example
Background
Key content
Introduction
Methods
Results
Related work
Fund
Key content
- Pretraining has recently greatly promoted the development of natural language processing (NLP)
- We show that M6 outperforms the baselines in multimodal downstream tasks, and the large M6 with 10 parameters can reach a better performance
- We propose a method called M6 that is able to process information of multiple modalities and perform both single-modal and cross-modal understanding and generation
- The model is scaled to large model with 10 billion parameters with sophisticated deployment, and the 10 -parameter M6-large is the largest pretrained model in Chinese
- Experimental results show that our proposed M6 outperforms the baseline in a number of downstream tasks concerning both single modality and multiple modalities We will continue the pretraining of extremely large models by increasing data to explore the limit of its performance
Upload PDF to Generate Summary
Must-Reading Tree
Example
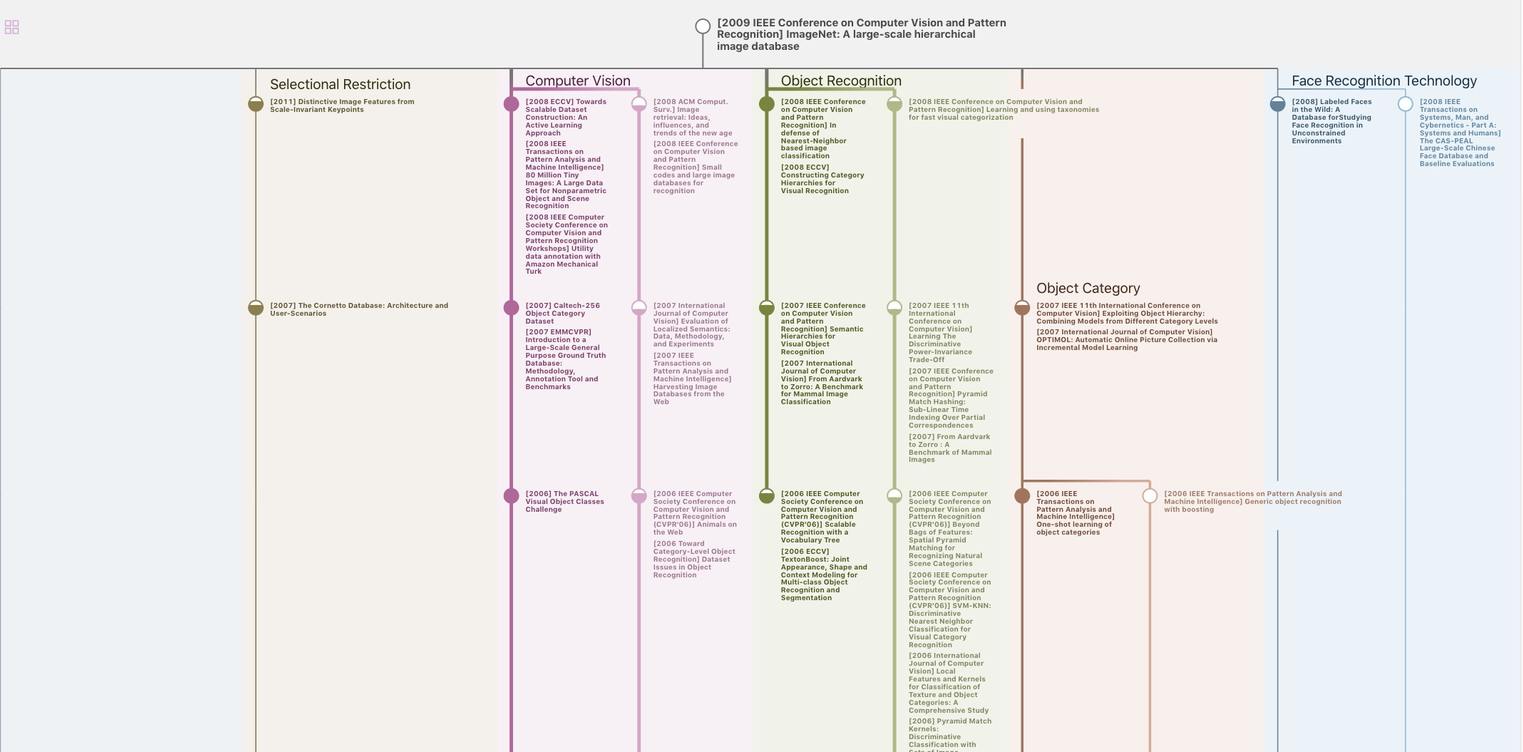
Generate MRT to find the research sequence of this paper
Related Papers
Detection of the Number of Exponentials by Invariant-Signal-Subspace Matching
IEEE Trans Signal Process 2024
被引用31
Robust Subspace Tracking by Soft-Projections and Signal Subspace Matching
IEEE TRANSACTIONS ON AEROSPACE AND ELECTRONIC SYSTEMS 2023
被引用0
Data Disclaimer
The page data are from open Internet sources, cooperative publishers and automatic analysis results through AI technology. We do not make any commitments and guarantees for the validity, accuracy, correctness, reliability, completeness and timeliness of the page data. If you have any questions, please contact us by email: report@aminer.cn
Chat Paper