Machine learning of atomic forces from quantum mechanics: An approach based on pairwise interatomic forces
INTERNATIONAL JOURNAL OF QUANTUM CHEMISTRY(2022)
摘要
In this work, we explore a machine learning approach based on pairwise interatomic forces to directly predict atomic forces in a molecule to quantum chemical accuracy. The scheme, denoted pairF-Net, uses a neural network designed and trained to predict Cartesian forces as a linear combination of a set of force components in an interatomic basis; these pairwise forces exhibit chemically intuitive profiles and implicitly maintain rotational and translational invariance. Application of the approach to model small molecule systems indicates an accuracy in reconstructed Cartesian atomic forces of similar to 1 kcal mol(-1) angstrom(-1) from the reference force values obtained via density functional theory. The pairF-Net scheme predicts atomic forces at a quantum mechanical level but at a fraction of the cost, enabling molecular dynamics simulations via a chemically intuitive machine learning model.
更多查看译文
关键词
artificial neural network, interatomic forces, machine learning, molecular dynamics simulation, pairwise force decomposition, quantum mechanics
AI 理解论文
溯源树
样例
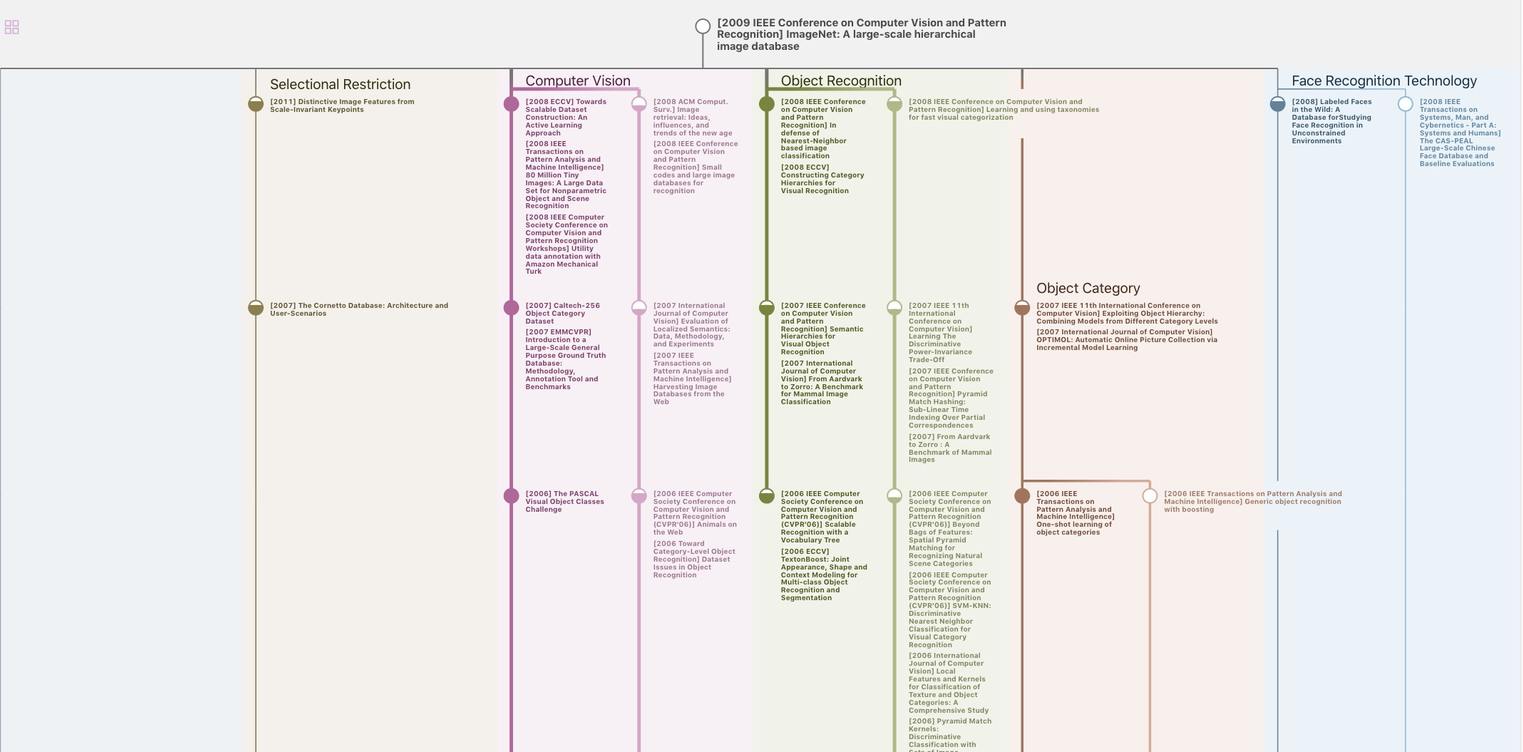
生成溯源树,研究论文发展脉络
Chat Paper
正在生成论文摘要