Improving Cancer Metastasis Detection via Effective Contrastive Learning
MATHEMATICS(2022)
摘要
The metastasis detection in lymph nodes via microscopic examination of H&E stained histopathological images is one of the most crucial diagnostic procedures for breast cancer staging. The manual analysis is extremely labor-intensive and time-consuming because of complexities and diversities of histopathological images. Deep learning has been utilized in automatic cancer metastasis detection in recent years. The success of supervised deep learning is credited to a large labeled dataset, which is hard to obtain in medical image analysis. Contrastive learning, a branch of self-supervised learning, can help in this aspect through introducing an advanced strategy to learn discriminative feature representations from unlabeled images. In this paper, we propose to improve breast cancer metastasis detection through self-supervised contrastive learning, which is used as an accessional task in the detection pipeline, allowing a feature extractor to learn more valuable representations, even if there are fewer annotation images. Furthermore, we extend the proposed approach to exploit unlabeled images in a semi-supervised manner, as self-supervision does not need labeled data at all. Extensive experiments on the benchmark Camelyon2016 Grand Challenge dataset demonstrate that self-supervision can improve cancer metastasis detection performance leading to state-of-the-art results.
更多查看译文
关键词
convolutional neural network, contrastive learning, self-supervision, deep learning, breast cancer detection
AI 理解论文
溯源树
样例
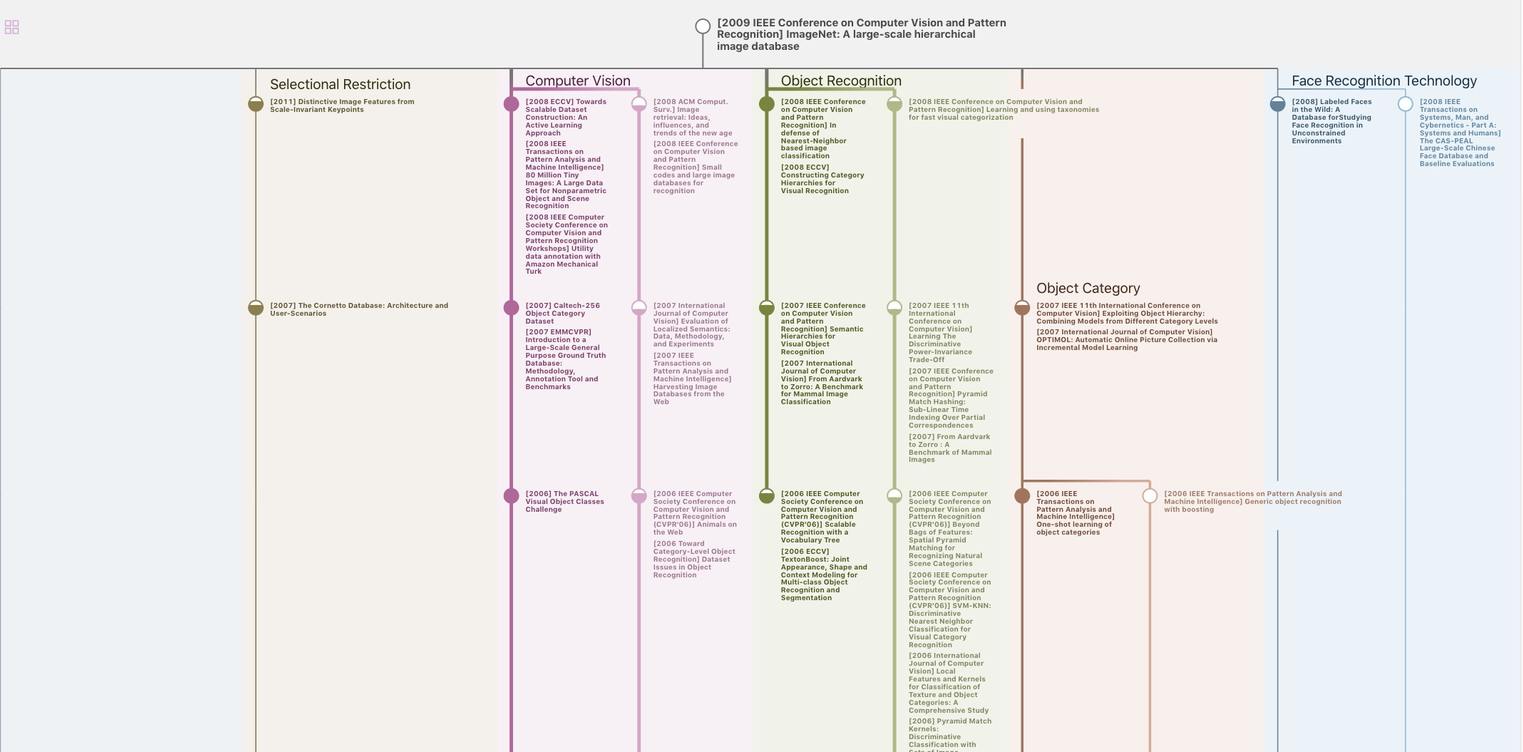
生成溯源树,研究论文发展脉络
Chat Paper
正在生成论文摘要