Hierarchical latent variables structure for topic aware multi-turn conversation
JOURNAL OF INTELLIGENT & FUZZY SYSTEMS(2022)
摘要
Hierarchical structures have emerged as a powerful framework for response generation, which can generate fluent responses in multi-turn conversation. However, the generated responses are often generic and bland. Some researchers have adopted latent variables to improve the diversity of responses, but they can not make full use of the information from multi-turn background, leading to meaningless replies with irrelevant topics. In order to fully utilize the background information for generating diverse and informative responses, we propose a Variational Hierarchical Conversation RNNs model with Topic aware latent variables (VHCR-T). The model contains three levels of latent variables: the global level latent variable to represent background information, the topic level latent variable to capture topic-related information, and the sentence level latent variable to increase the response diversity. When modeling the topic information, we design two different topic level latent variables to maintain the dialog coherence and role preference, and to enhance the context sensitiveness, respectively. Experimental results on Cornell Movie Dialog and Ubuntu Dialog Corpus show that our model outperforms the state-of-the-art models for multi-turn conversation generation in terms of diversity and informativeness, verifying the effectiveness of our VHCR-T model.
更多查看译文
关键词
Multi-turn conversation, response generation, hierarchical structure, topic, latent variable
AI 理解论文
溯源树
样例
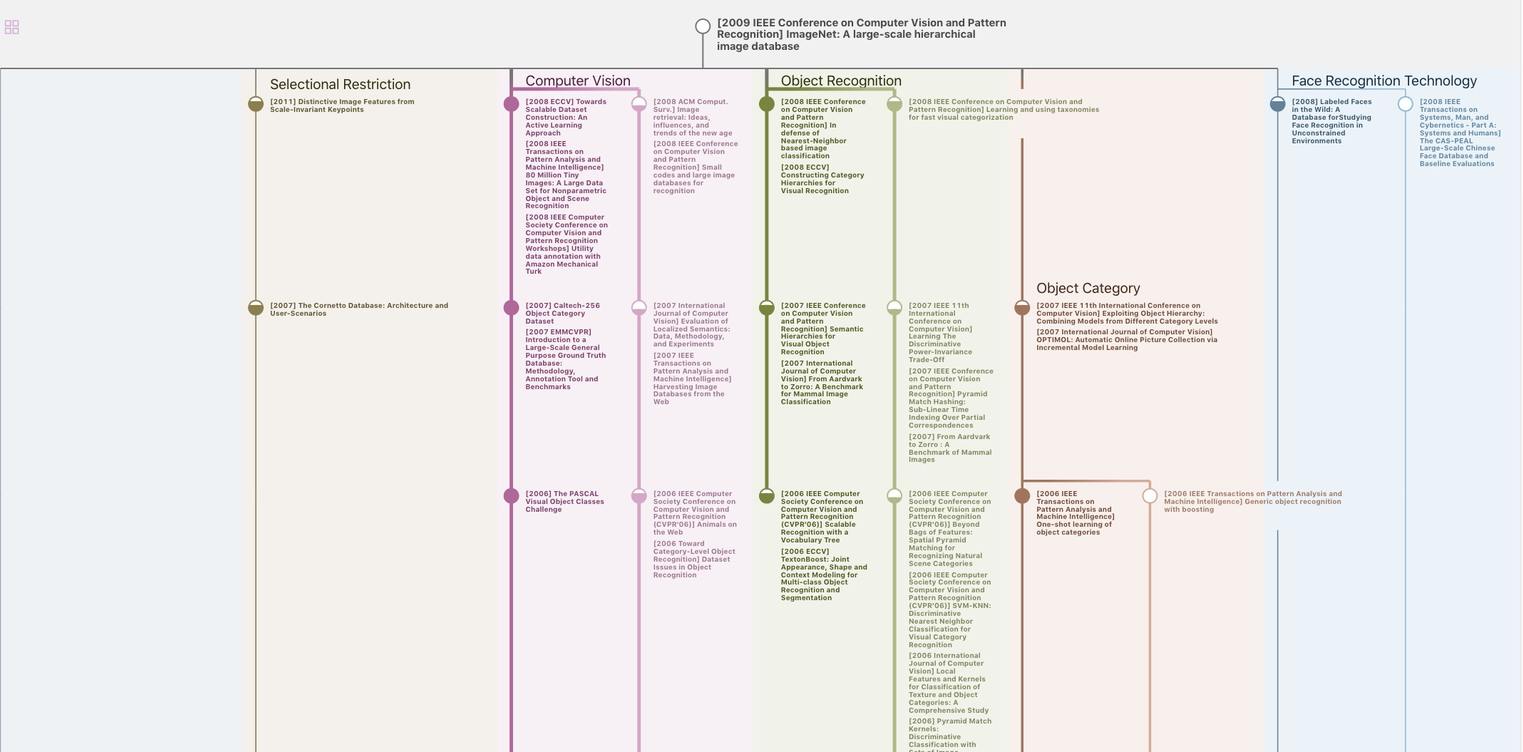
生成溯源树,研究论文发展脉络
Chat Paper
正在生成论文摘要