Unsupervised double weighted graphs via good neighbours for dimension reduction of hyperspectral image
INTERNATIONAL JOURNAL OF REMOTE SENSING(2022)
摘要
As the major research in pattern recognition, unsupervised dimension reduction is a challenging problem because of no label information. Most unsupervised dimension reduction methods usually construct similarity graph by k-nearest neighbour to preserve local structure in the low-dimensional subspace. However, k-nearest neighbour is calculated by Euclidean distance, which is sensitive to noise and outliers. And only considering local structure will reduce the classification accuracy. In this paper, a new unsupervised dimension reduction method called unsupervised double-weighted graphs via good neighbours (uDWG-GN) is proposed. First, uDWG-GN proposes a local structure Low-Rank Representation to learn similarity matrix and then uses the similarity matrix to find good neighbours of each sample. Second, according to good neighbours of the sample, uDWG-GN considers similar and dissimilar relationship between samples and constructs double-weighted graphs. Finally, based on L-2,L-1 norm, uDWG-GN finds the optimal projection matrix by maximizing the distance of dissimilar samples and minimizing the distance of similar samples. Experimental results on three hyperspectral images demonstrate the superiority and effectiveness of our method compared with other dimension reduction methods.
更多查看译文
关键词
Unsupervised learning, dimension reduction, good neighbour, feature extraction, hyperspectral data
AI 理解论文
溯源树
样例
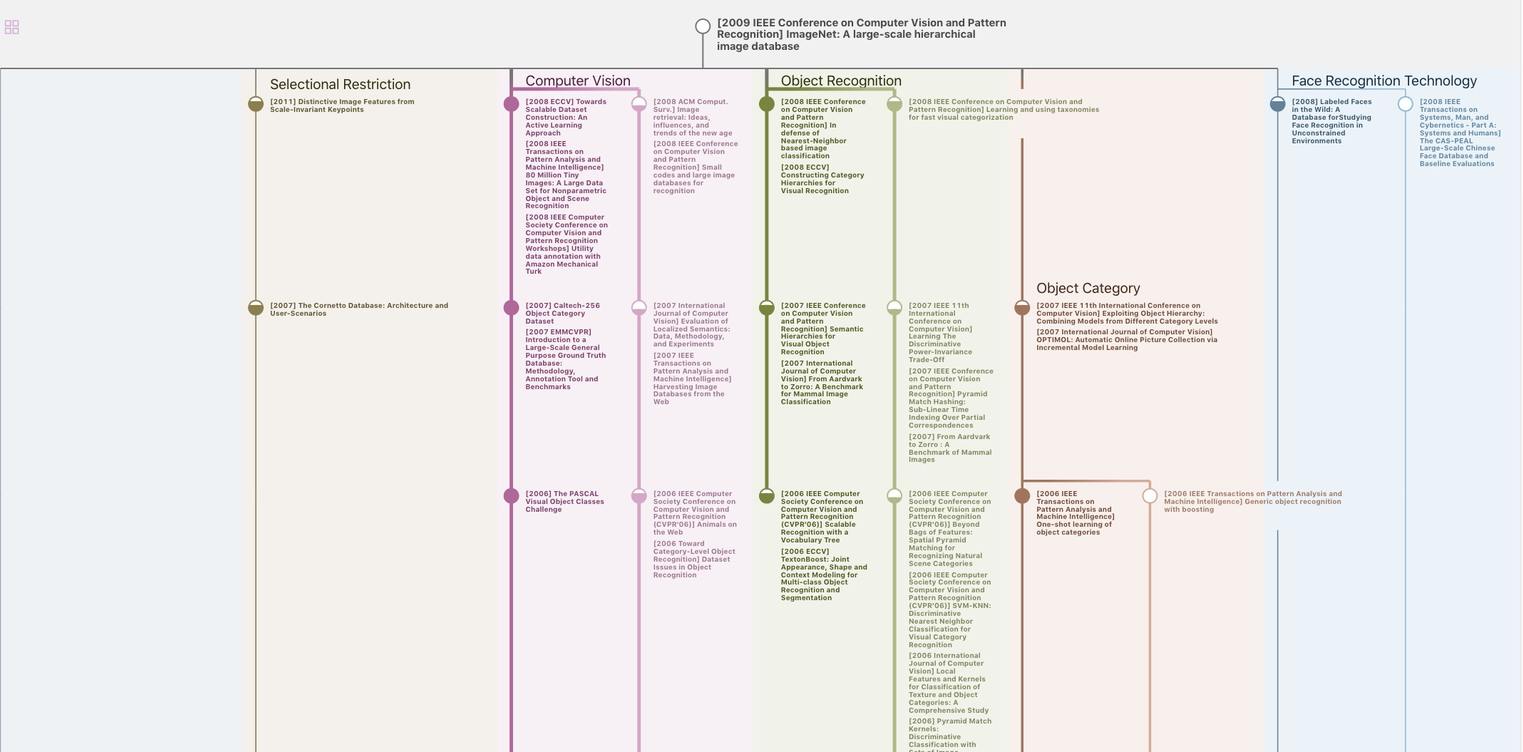
生成溯源树,研究论文发展脉络
Chat Paper
正在生成论文摘要