An Improved Model for Kernel Density Estimation Based on Quadtree and Quasi-Interpolation
Mathematics(2022)
Abstract
There are three main problems for classical kernel density estimation in its application: boundary problem, over-smoothing problem of high (low)-density region and low-efficiency problem of large samples. A new improved model of multivariate adaptive binned quasi-interpolation density estimation based on a quadtree algorithm and quasi-interpolation is proposed, which can avoid the deficiency in the classical kernel density estimation model and improve the precision of the model. The model is constructed in three steps. Firstly, the binned threshold is set from the three dimensions of sample number, width of bins and kurtosis, and the bounded domain is adaptively partitioned into several non-intersecting bins (intervals) by using the iteration idea from the quadtree algorithm. Then, based on the good properties of the quasi-interpolation, the kernel functions of the density estimation model are constructed by introducing the theory of quasi-interpolation. Finally, the binned coefficients of the density estimation model are constructed by using the idea of frequency replacing probability. Simulation of the Monte Carlo method shows that the proposed non-parametric model can effectively solve the three shortcomings of the classical kernel density estimation model and significantly improve the prediction accuracy and calculation efficiency of the density function for large samples.
MoreTranslated text
Key words
large samples,kernel density estimation,quasi-interpolation,quadtree algorithm,adaptive
AI Read Science
Must-Reading Tree
Example
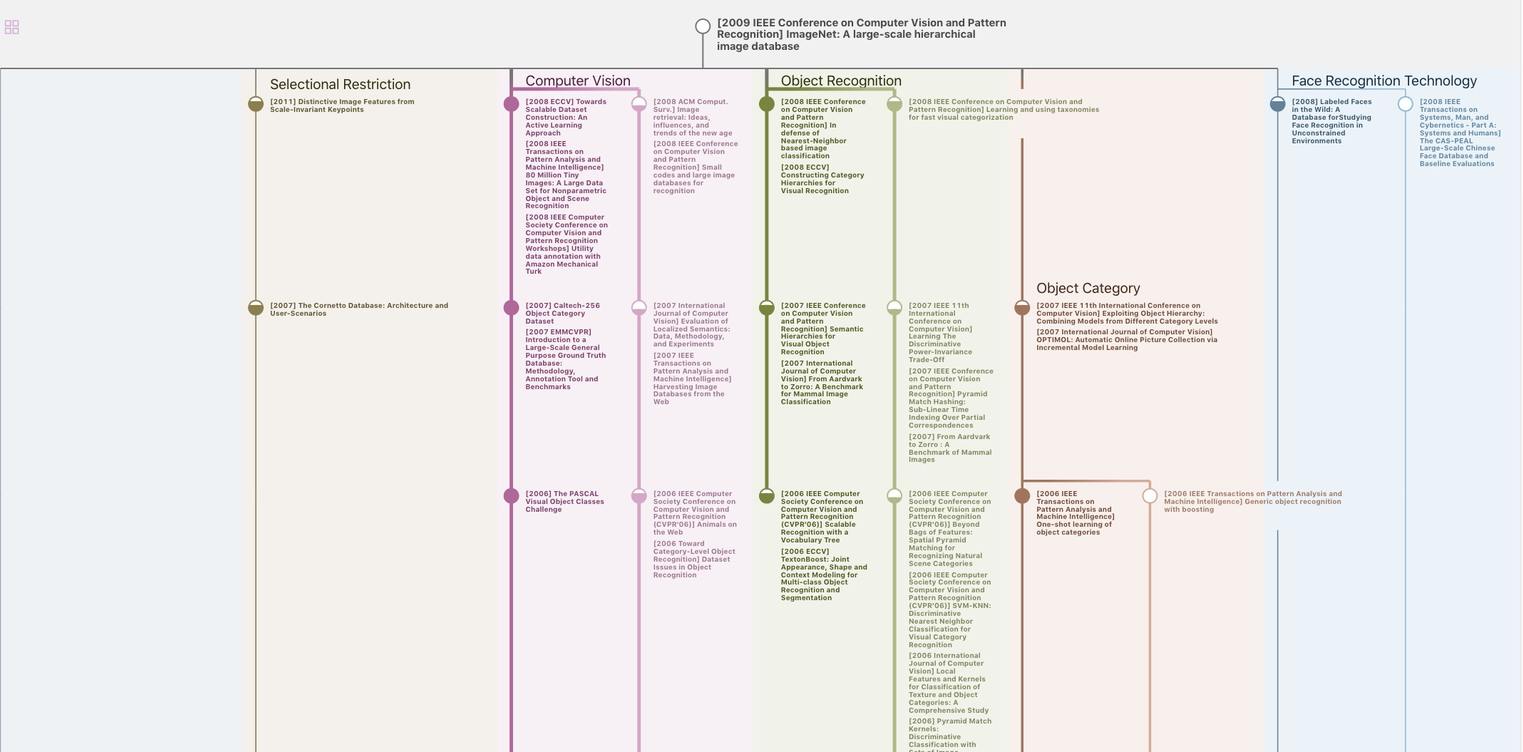
Generate MRT to find the research sequence of this paper
Chat Paper
Summary is being generated by the instructions you defined