Target Tracking Algorithm Based on Siamese Network of Feature Optimization Model
LASER & OPTOELECTRONICS PROGRESS(2022)
摘要
In the target tracking sequences, it is difficult to identify the target because of the complex background and large-scale changes of the target. To solve this problem, a target tracking algorithm based on feature optimization model in the Siamese network is proposed. First, the deep network is constructed to extract the deep semantic information effectively. Then, the hourglass network is used to encode the global features of the multi-scale feature map, and the encoded features are normalized to obtain the effective target features. Finally, a feature optimization model is constructed, and the features obtained by decoding are used as selectors to identify and enhance the effective features of the original feature map. In order to further improve the generalization ability of the model, the attention mechanism is introduced to adaptively weigh the target features to adapt to the scene changes. The proposed algorithm is tested on two standard tracking data sets including OTB100 and VOT2018. The success rate in the OTB100 is 0.648, the prediction accuracy is 0.853, and the real-time performance is 59.5 frame/s; the test accuracy in the VOT2018 is 0.536, the expected average coverage rate is 0.192, and the real-time performance is 44.3 frame/s. The test results prove the effectiveness of the proposed algorithm.
更多查看译文
关键词
machine vision, deep learning, target tracking, Siamese network, feature optimization, feature fusion
AI 理解论文
溯源树
样例
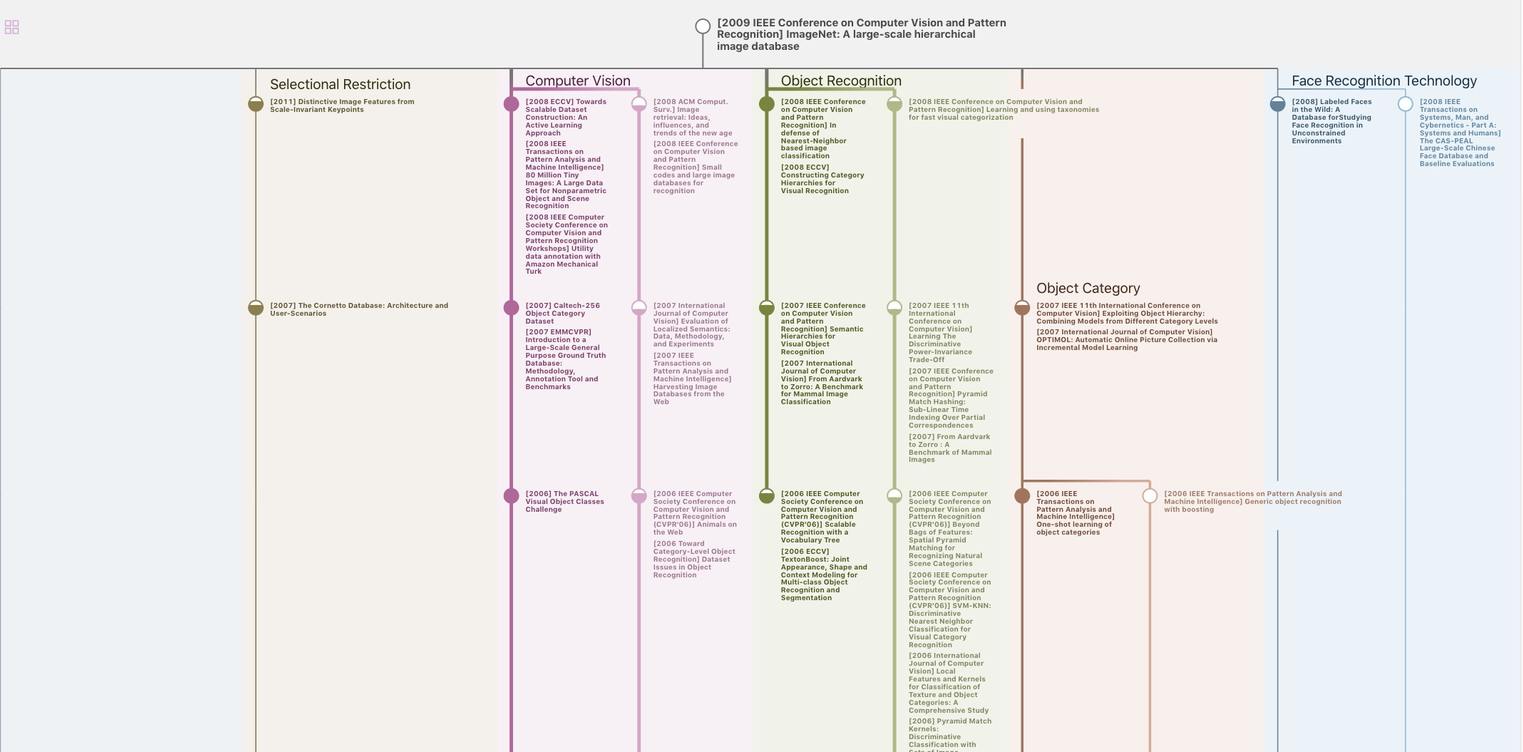
生成溯源树,研究论文发展脉络
Chat Paper
正在生成论文摘要