Few shot learning-based fast adaptation for human activity recognition
Pattern Recognition Letters(2022)
摘要
Sensor-based human activity recognition (HAR) is a common application in the fields of mobile computing and pattern recognition. Existing approaches and models of HAR can present ideal recognition performance only in well-designed, specific, and deterministic scenarios. However, in real scenes, new types of activities, new human bodies that performs activities, and other new situations are encountered. When new situations arise, it is difficult to collect sufficient and high-quality data in time. Thus, the existing approaches and models suffer from a lack of interoperability and scalability. To address these challenges, this study proposes a Model-agnostic Meta Learnings(MAML)-Coarse-Fine Convolutional Neural Networks(CFCNN) mixed task strategy to achieve fast adaptation to the human activity recognition under new situations. This is a novel method that incorporates shot learning to recognize tasks in situations where several kinds of new scenarios exist. First, some modifications were carried out on traditional MAML for multi-scale feature extraction, and a mixed task strategy was adopted during training. The proposed method improves the generalization ability of the model, and is capable of learning quickly when dealing with diffirent new tasks. Finally, the model was compared with other models when facing two new scenes of a new activity category and a new human body. The results showed that the accuracy of the proposed MAML-CFCNN based on the mixed task strategy is higher than that of the state-of-the-art methods, including MAML, First-Order MAML (FOMAML), REPTILE, and so on.
更多查看译文
AI 理解论文
溯源树
样例
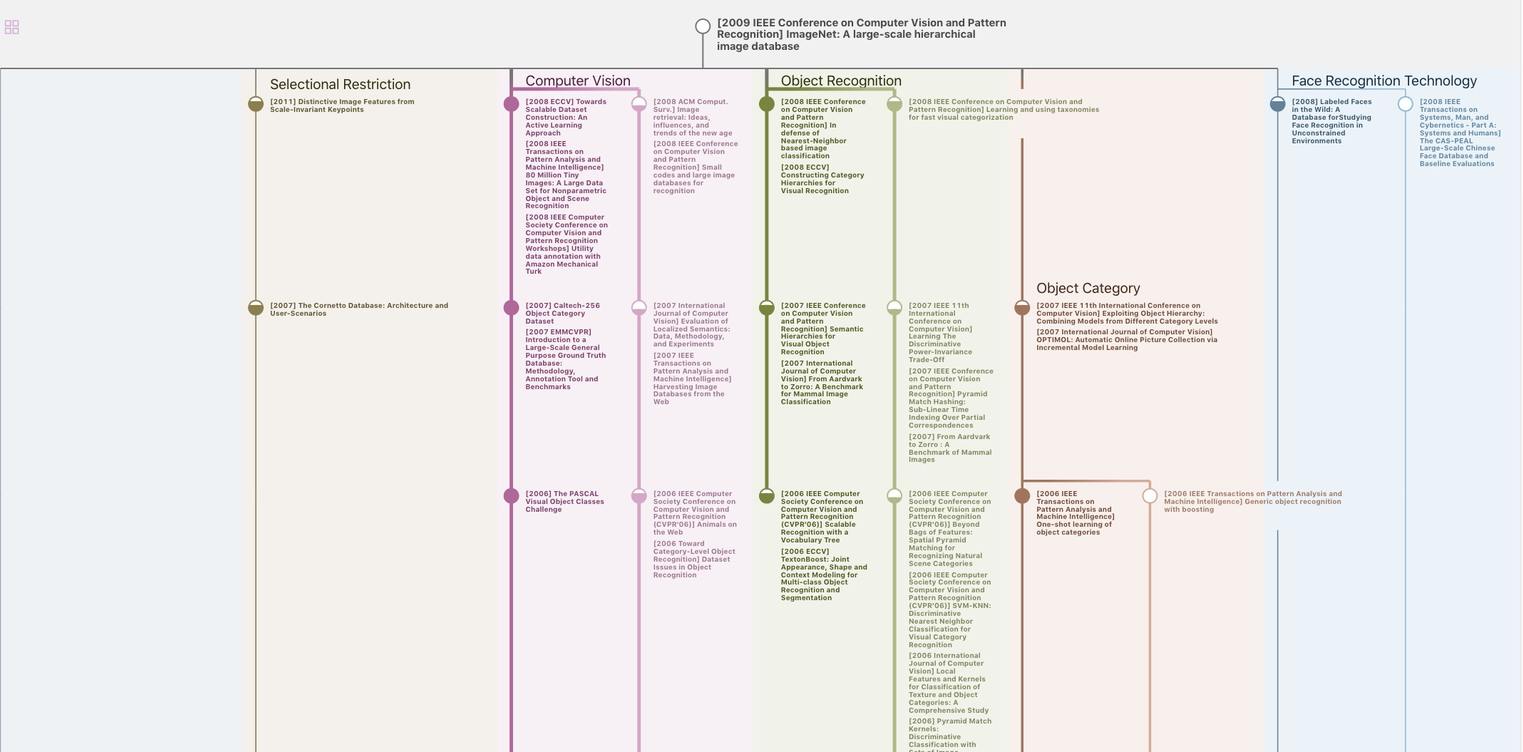
生成溯源树,研究论文发展脉络
Chat Paper
正在生成论文摘要