Enhanced image super-resolution using hierarchical generative adversarial network
INTERNATIONAL JOURNAL OF COMPUTING SCIENCE AND MATHEMATICS(2022)
摘要
Recently, generative adversarial networks (GAN) have been introduced in single-image super-resolution (SISR) to reconstruct more realistic high-resolution (HR) images. In this paper, we propose an effective SISR method, named super-resolution using hierarchical generative adversarial network (SRHGAN), based on the idea of GAN and the prior knowledge. Different from the existing GANs that focus on the depth of networks, our proposed method considers the prior knowledge in addition. That is, we introduce an edge extraction branch and an edge enhancement branch into GAN for considering the edge information. By means of the added edge loss in the loss function, the edge extraction branch and the edge enhancement branch will be trained to reconstruct the sharp edge well. Experimental results on several datasets illustrate that our reconstructed visual effect images are clearer and sharper than some related SISR methods.
更多查看译文
关键词
single image super-resolution, deep learning, generative adversarial network, hierarchical network, edge prior
AI 理解论文
溯源树
样例
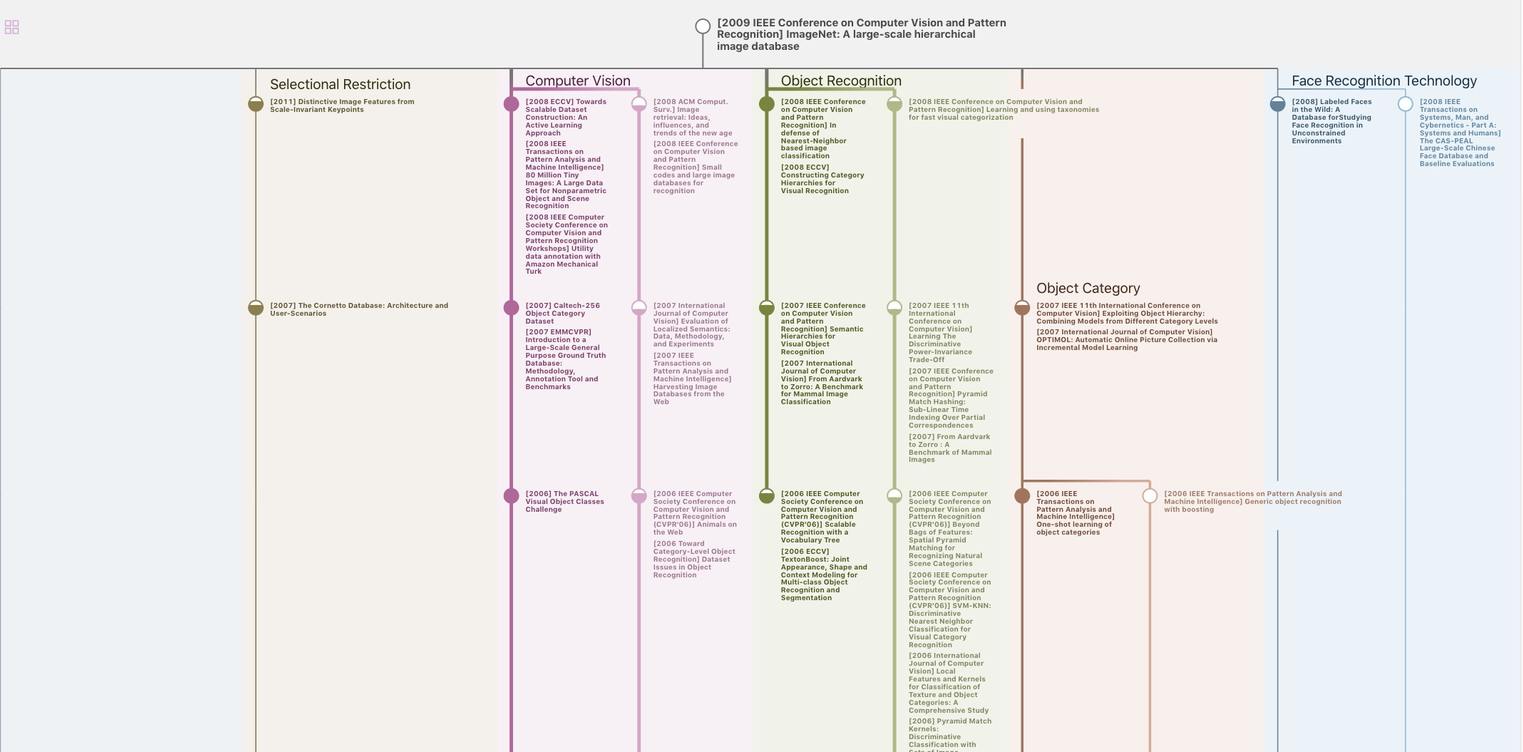
生成溯源树,研究论文发展脉络
Chat Paper
正在生成论文摘要