Lightweight Yolov4 Target Detection Algorithm Fused with ECA Mechanism
Processes(2022)
摘要
For the task of garbage classification, to overcome the main disadvantages of the Yolov4 target detection algorithm, such as the large network model and lower detection accuracy for small objects, a lightweight Yolov4 target detection network based on the EfficientNet-B0 fusion ECA mechanism is presented. The lightweight EfficientNet was used to replace the original backbone network, which reduces the parameters of the network model and improves the detection accuracy. Moreover, a deep detachable convolution block replaced the common convolution block in the original network, which further reduced the number of parameters in the model. In the feature pyramid model PANet, a lightweight ECA attention mechanism was introduced to realize the weight analysis of the importance of different channel feature maps through cross-channel interaction, allowing the network to extract more obvious features with which to distinguish categories. Finally, a Soft-NMS algorithm was introduced in the post-processing stage of the detection frame to reduce the missed target detection rate in dense areas, which can improve the detection accuracy of the network and detection efficiency. As shown in the results, the size of the model was only 48 MB, and the mAP was 91.09%. Compared with the original Yolov4 network, the mAP was increased by 5.77% based on the 80% reduction in the model size. The recognition of small targets was also improved, which proved the effectiveness and robustness of the improved algorithm.
更多查看译文
关键词
Yolov4,EfficientNet,depth-wise separable convolution,ECA,Soft-NMS
AI 理解论文
溯源树
样例
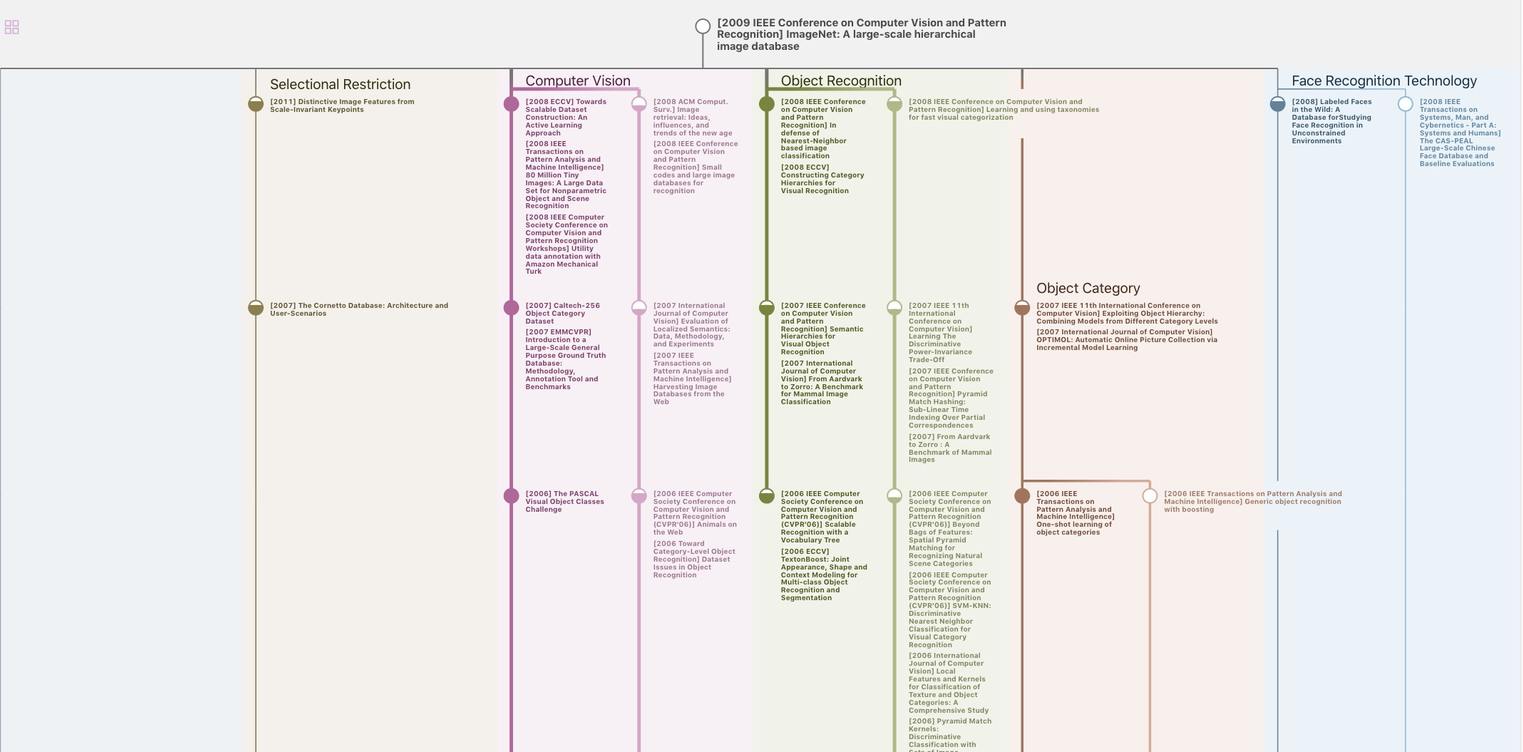
生成溯源树,研究论文发展脉络
Chat Paper
正在生成论文摘要