FIBS-Unet: Feature Integration and Block Smoothing Network for Single Image Dehazing
IEEE ACCESS(2022)
摘要
The dehazing algorithms are based on the hazy simulation equation to remove haze and restore the input image feature maps by estimating the intensity coefficient of the atmospheric light source and the scattering coefficient of the atmosphere. However, the coefficient prediction isn't good, resulting in artifact noise in the dehazed output image. The increasing expansion of deep learning algorithms in computer vision applications to combat noise and interference in the hazy picture is growing. This paper proposed an efficient framework for Feature Integration and Block Smoothing (FIBS-Unet) Unet architecture using encoder-decoder processing with intensity attention block. We modified the Res2Net residual block with customized convolution and added instance normalization to improve the encoder feature extraction efficiency. Besides, we designed the Intensity Attention Block (IAB) using Sub-Pixel Layer and convolution (1 x1 ) to amplify input feature and fusion feature maps. We developed an efficient decoder employing sub-pixel convolutions, concatenations, contrive convolutions, and multipliers to recover smooth and high-quality feature maps at the framework. The proposed FIBS-Unet has minimized the Mean Absolute Error (MAE) at perceptual loss function with the RESIDE dataset. We calculated the Peak Signal-to-Noise Ratio (PSNR), the Similarity Index Measure (SSIM), and a subjective visual color difference to evaluate the model's effectiveness. The proposed FIBS-Unet achieved better quality dehazing image results of PSNR:34.122 and SSIM:0.9890 in the outdoor scenarios at dense haze and backlight image for the Synthetic Objective Testing Set (SOTS). Our extensive experimental results specify that proposed FIBS-Unet is extendable to real-time applications.
更多查看译文
关键词
Image dehazing,feature extraction,feature fusion,intensity attention block,instance normalization,residual connection,sub-pixel convolution
AI 理解论文
溯源树
样例
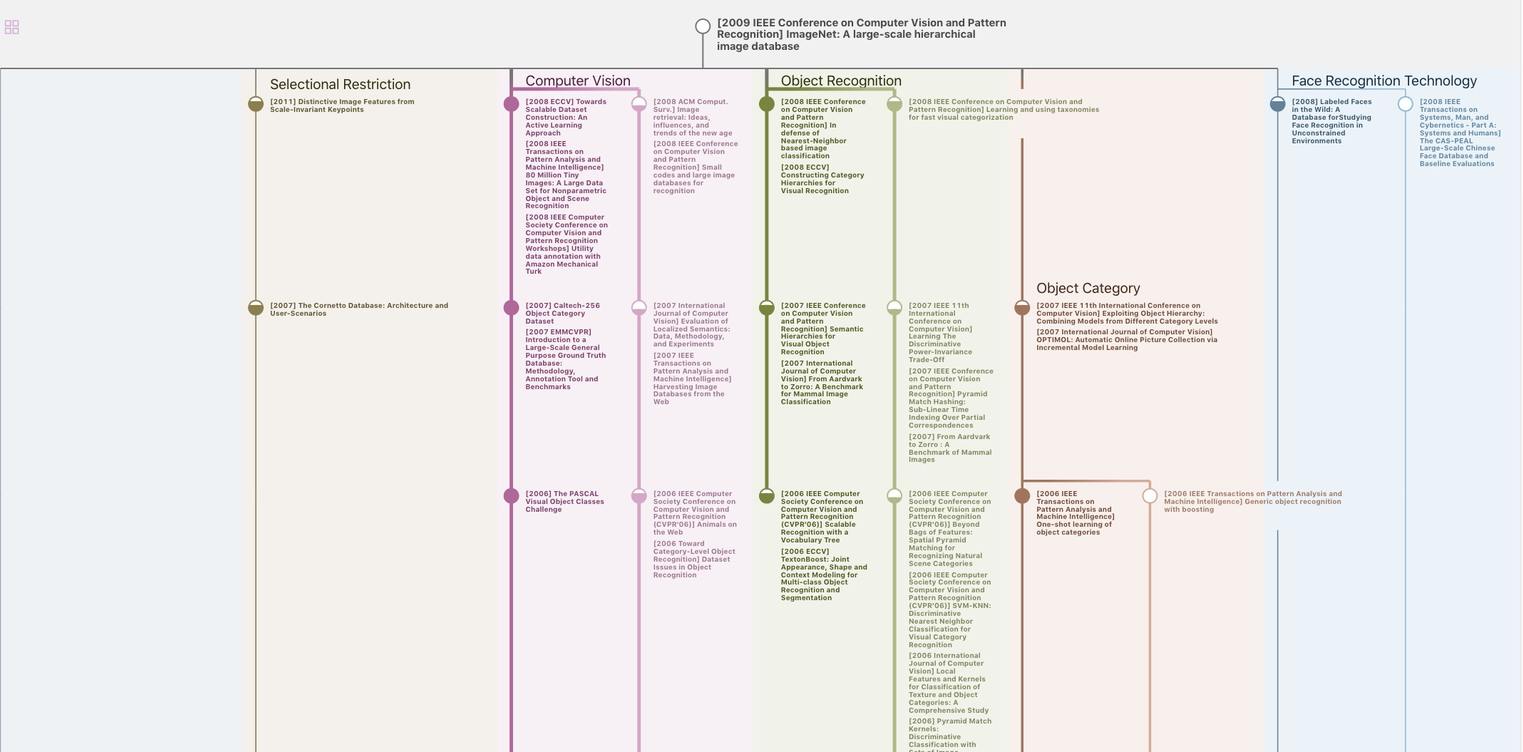
生成溯源树,研究论文发展脉络
Chat Paper
正在生成论文摘要