A Modeling Framework for Assessing the Value of Learning in Dynamic Adaptive Planning: Application to Green Infrastructure Investment Evaluation
WATER RESOURCES RESEARCH(2022)
摘要
To cope with the uncertainty of green infrastructure planning, many cities take an adaptive approach and use learning-by-doing to improve estimates of the cost and efficacy of stormwater management practices (SMPs) and use that information to improve stormwater plans. However, deciding whether that learning is worth its expense has been a challenge for practitioners. We propose a modeling framework to assess the economic value of learning. Methodologically, we present a generalized adaptive planning method that includes learning from direct and indirect investments and multiple degrees of learning. The formulation enables users to specify possible knowledge gains from near-term actions and quantify its value by assessing its impacts on subsequent decisions and their performance. Further, we quantify the values of both learning and adaptability by calculating differences in expected system performance between three types of decision making: non-adaptive (no learning between decisions), passive adaptive (adaptive planning that passively accepts incidental learning), and active adaptive (adaptive planning that considers potential learning opportunities when choosing investments). For illustration, we apply the framework to an example inspired by a real stormwater management setting in Philadelphia, PA. In the example, the ability of SMPs to reduce runoff is evaluated by hydrological simulation. The literature and expert opinions inform estimates of costs, SMP performance deterioration over time, and predictions of possible knowledge gains. The results show that active adaptive planning supported by stochastic optimization can achieve substantial cost savings. Plain Language Summary How much can stormwater plans be improved by investing in learning? Is that investment worthwhile? This paper presents a modeling approach building on our previous work to answer these questions. Specifically, the new method accounts for multiple degrees of learning from local investments and experience gained from other locations. Using this method, we can calculate the cost difference between plans that use trial-and-error (passive adaptive) and active experimentation (active adaptive) management solutions, called the "value of learning," while those savings achieved only from incorporating lessons learned passively are called the "value of adaptivity." To illustrate the use of the method, we apply it to an example of green infrastructure planning based on the settings of a real stormwater management program in Philadelphia, PA. We evaluate technology efficacy by hydrological simulations and rely on expert opinions and literature for the costs of the technologies, performance deterioration over time, and the prediction of possible knowledge gains. The results show that taking the active adaptive approach can potentially save hundreds of millions USD by investments to enhance our understanding of stormwater management practices and stimulate technology improvement.
更多查看译文
关键词
BMP, SWMM, uncertainty, urban, Bayesian Inference, maintenance
AI 理解论文
溯源树
样例
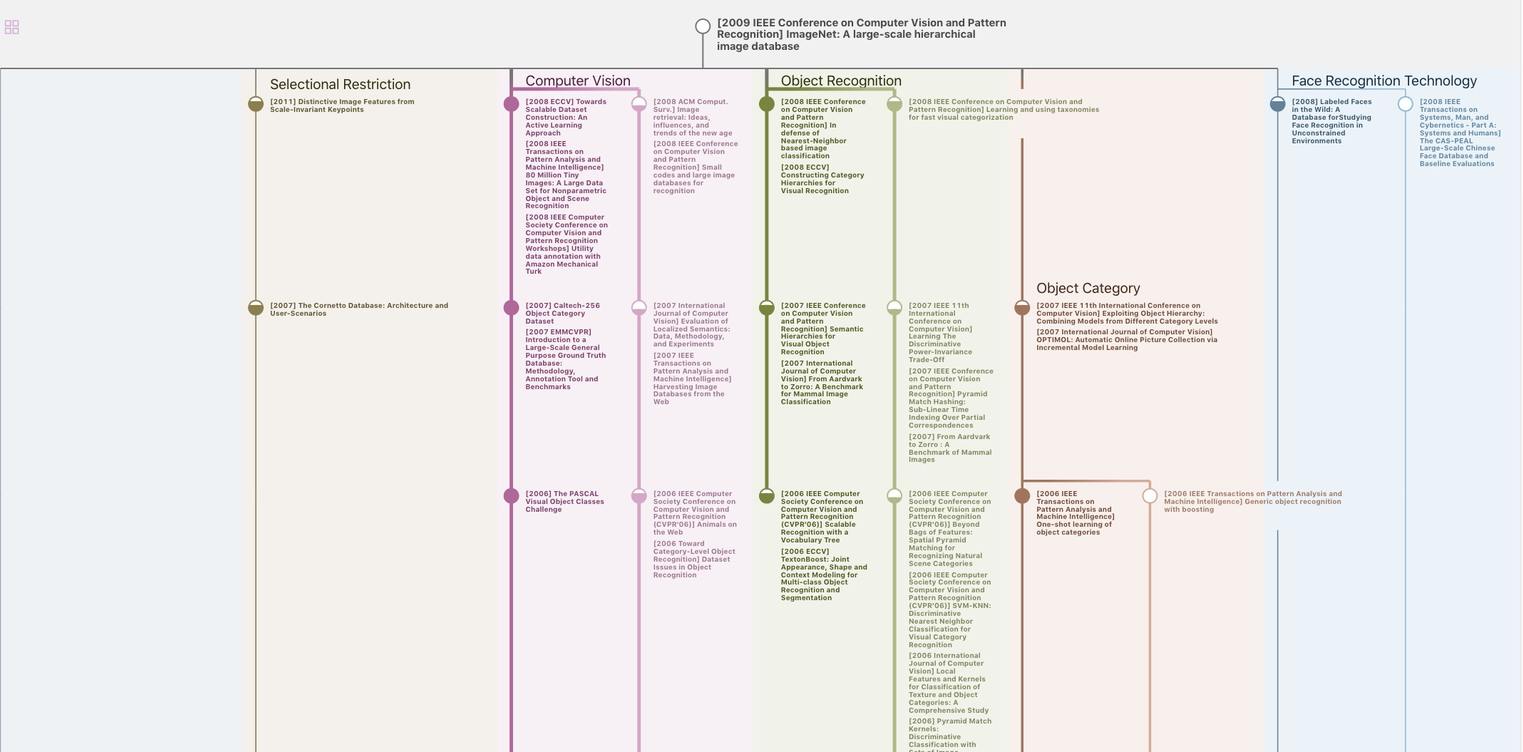
生成溯源树,研究论文发展脉络
Chat Paper
正在生成论文摘要