Improving prostate cancer triage with GAN-based synthetically generated prostate ADC MRI
MEDICAL IMAGING 2022: COMPUTER-AIDED DIAGNOSIS(2022)
摘要
Tumor classification in clinically significant (cS, Gleason score >= 7) or non-clinically significant (ncS, Gleason score < 7) plays a crucial role in patient management of prostate cancer (PCa), allowing to triage those patients that might benefit from an active surveillance approach from those that require an immediate action in the form of further testing or treatment. In spite of it, the current diagnostic pathway of PCa is substantially hampered by over-diagnosis of ncS lesions and under-detection of cS ones. Magnetic Resonance Imaging (MRI) has proven to be helpful in the stratification of tumors, but it relies on specialized training and experience. Despite the promise shown by deep learning (DL) methods, they are data-hungry approaches and rely on the availability of large amounts of annotated data. Standard augmentation techniques such as image translation have become the by default option to increase variability and data availability. However, the correlation between transformed data and original one limits the amount of information provided by them. Generative Adversarial Networks (GAN) present an alternative to classic augmentation techniques by creating synthetic samples. In this paper, we explore a conditional GAN (cGAN) architecture and a deep convolutional one (DCGAN) to generate synthetic apparent diffusion coefficient (ADC) prostate MRI. Following, we compare classic augmentation techniques with our GAN-based approach in a prostate cancer triage (classification of tumors) setting. We show that by adding synthetic ADC prostate MRI we are able to improve the final classification AUC of cS vs ncS tumors when compared to classic augmentation.
更多查看译文
关键词
Prostate Cancer,MRI,GAN,Classification,ADC
AI 理解论文
溯源树
样例
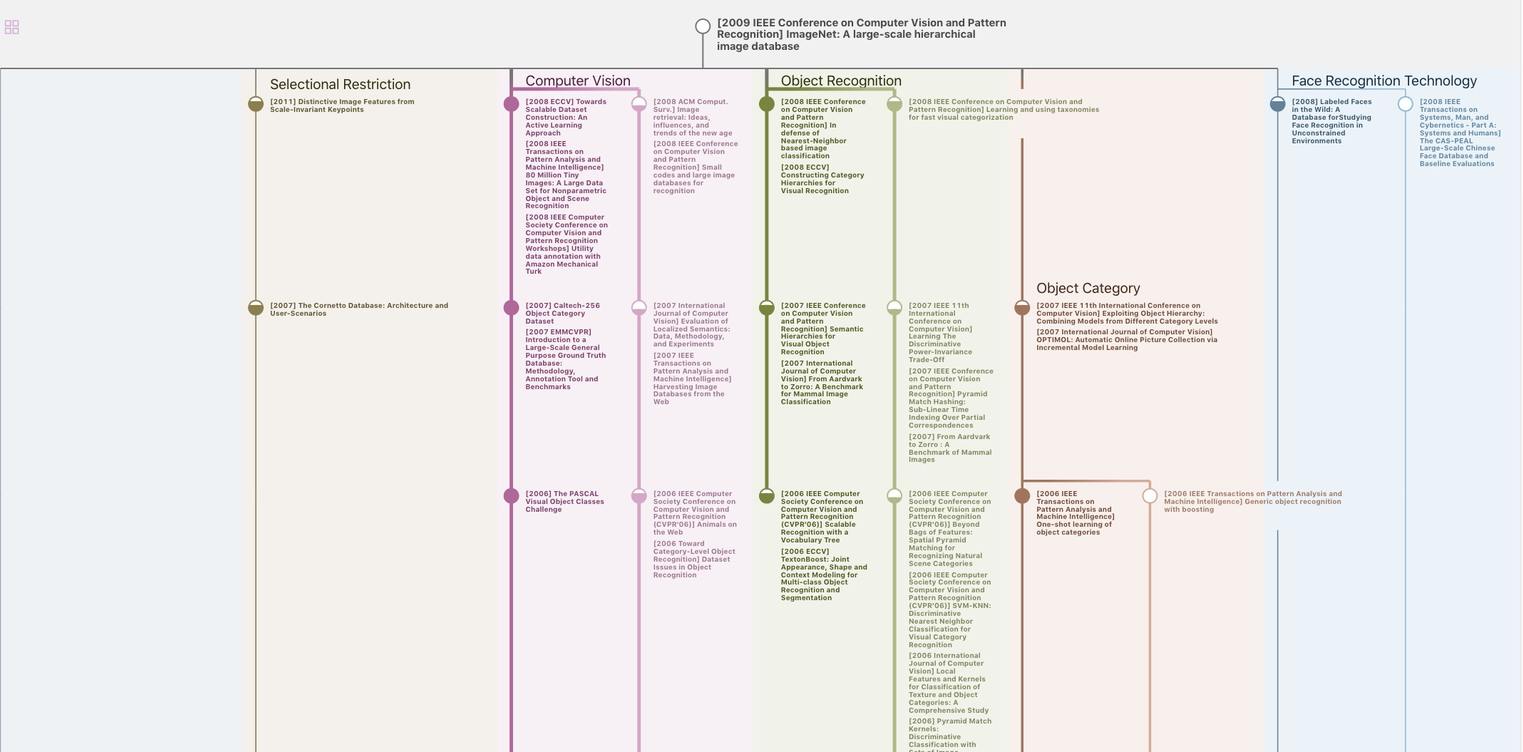
生成溯源树,研究论文发展脉络
Chat Paper
正在生成论文摘要