A Novel CNN plus LSTM Approach to Classify Frontal Chest X-Rays for Spine Fractures
MEDICAL IMAGING 2022: COMPUTER-AIDED DIAGNOSIS(2022)
摘要
Spine fractures are serious injuries that are often missed on standard chest x-rays, especially the frontal view. Automatic detection using artificial intelligence could help reduce these missed findings. To detect fractures, radiologists compare the morphological characteristics within the ordered vertebrae sequence. To this end, we designed a time-distributed CNN+LSTM model with Res-Net-inspired backbone to classify a sequence of vertebral image patches extracted from a frontal chest x-ray. While the CNN component encapsulates spatial characteristics of vertebrae, the time-distributed aspect ensures the sequential information of each image is explicitly preserved and better exploited by the LSTM. A Dense UNet was trained to automatically detect vertebrae locations for patch extraction. For this retrospective research- only study, a comparative analysis was performed using six random partitions of 1250 images, and the AUCs for each partition were averaged. Using manual vertebrae detection to extract vertebral images patches from the x-rays, the proposed method achieved an average AUC of 83.17 +/- 3.8%. Using the Dense UNet for automatic vertebrae detection in the pipeline, the proposed method achieved an average AUC of 79.3 +/- 1.8% in differentiating x-rays with and without vertebral fractures. This is an average improvement of 6.0% relative to the same CNN+LSTM architecture that is not time distributed. Using a model ensemble, an AUC of 82.7 +/- 3.7% was achieved. These findings suggest the importance of exploiting both sequential and spatial information for fracture detection.
更多查看译文
关键词
spine fracture,frontal chest x-rays,CNN,LSTM,classification,deep learning
AI 理解论文
溯源树
样例
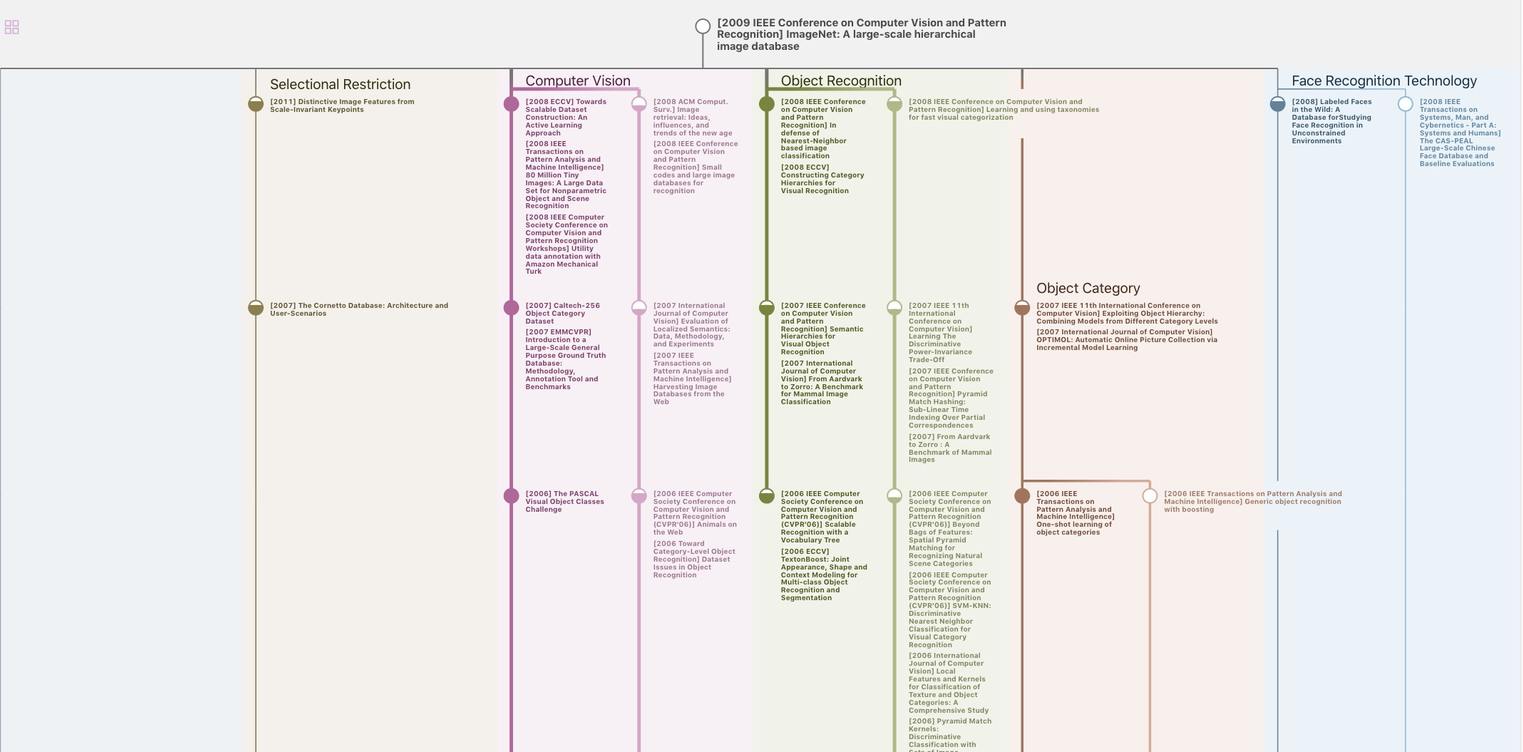
生成溯源树,研究论文发展脉络
Chat Paper
正在生成论文摘要