Evaluating the sensitivity of deep learning to inter-reader variations in lesion delineations on bi-parametric MRI in identifying clinically significant prostate cancer
MEDICAL IMAGING 2022: COMPUTER-AIDED DIAGNOSIS(2022)
摘要
Deep learning based convolutional neural networks (CNNs) for prostate cancer (PCa) risk stratification employ radiologist delineated regions of interest (ROIs) on MRI. These ROIs contain the reader's interpretation of the region of PCa. Variations in reader annotations change the features that are extracted from the ROIs, which may in turn affect classification performance of CNNs. In this study, we sought to analyze the effect of variations in inter-reader delineations of PCa ROIs on training of CNNs with regards to distinguishing clinically significant (csPCa) and insignificant PCa (ciPCa). We employed 180 patient studies (n=274 lesions) from 3 cohorts who underwent 3T multi-parametric MRI followed by MRI-targeted biopsy and/or radical prostatectomy. ISUP Gleason grade groups (GGG) obtained from pathology were used to determine csPCa (GGG >= 2) and ciPCa (GGG=1). 5 experienced radiologists, with over 5 years of experience in prostate imaging, delineated PCa ROIs on bi-parametric MRI (bpMRI including T2 weighted (T2W) and diffusion weighted (DWI) sequences) within the training set (n(1)=160 lesions) using targeted biopsy locations. Patches were extracted using the ROIs which were then used to train individual CNNs (N-1-N-5) using the SqueezeNet architecture. The average volume for reader-delineated ROIs used for training varied greatly, ranging between 1106 and 2107 mm across all readers. The resulting networks showed no significant difference in classification performance (AUC= 0.82 +/- 0.02) indicating that they were relatively robust to inter-reader variations in ROI. These models were evaluated on independent test sets (n(2)=85 lesions, n(3)=29 lesions) where ROIs were obtained by co-registration of MRI with post-surgical pathology, unaffected by inter-reader variations in ROIs. Network performance across D-2 and D-3 was 0.80 +/- 0.02 and 0.62 +/- 0.03, respectively. The CNN predictions were moderately consistent, with ICC(2,1) scores across D-2 and D-3 being 0.74 and 0.54, respectively. Higher agreement in ROI overlap produced higher correlation in predictions on external test sets (R = 0.89, p < 0.05). Furthermore, higher average ROI volume produced greater AUC scores on D-3, indicating that comprehensive ROIs may provide more features for DL networks to use in classification. Inter-reader variations in ROIs on MRI may influence the reliability and generalizability of CNNs trained for PCa risk stratification.
更多查看译文
关键词
Convolutional neural networks,Deep learning,prostate cancer,Magnetic Resonance Imaging
AI 理解论文
溯源树
样例
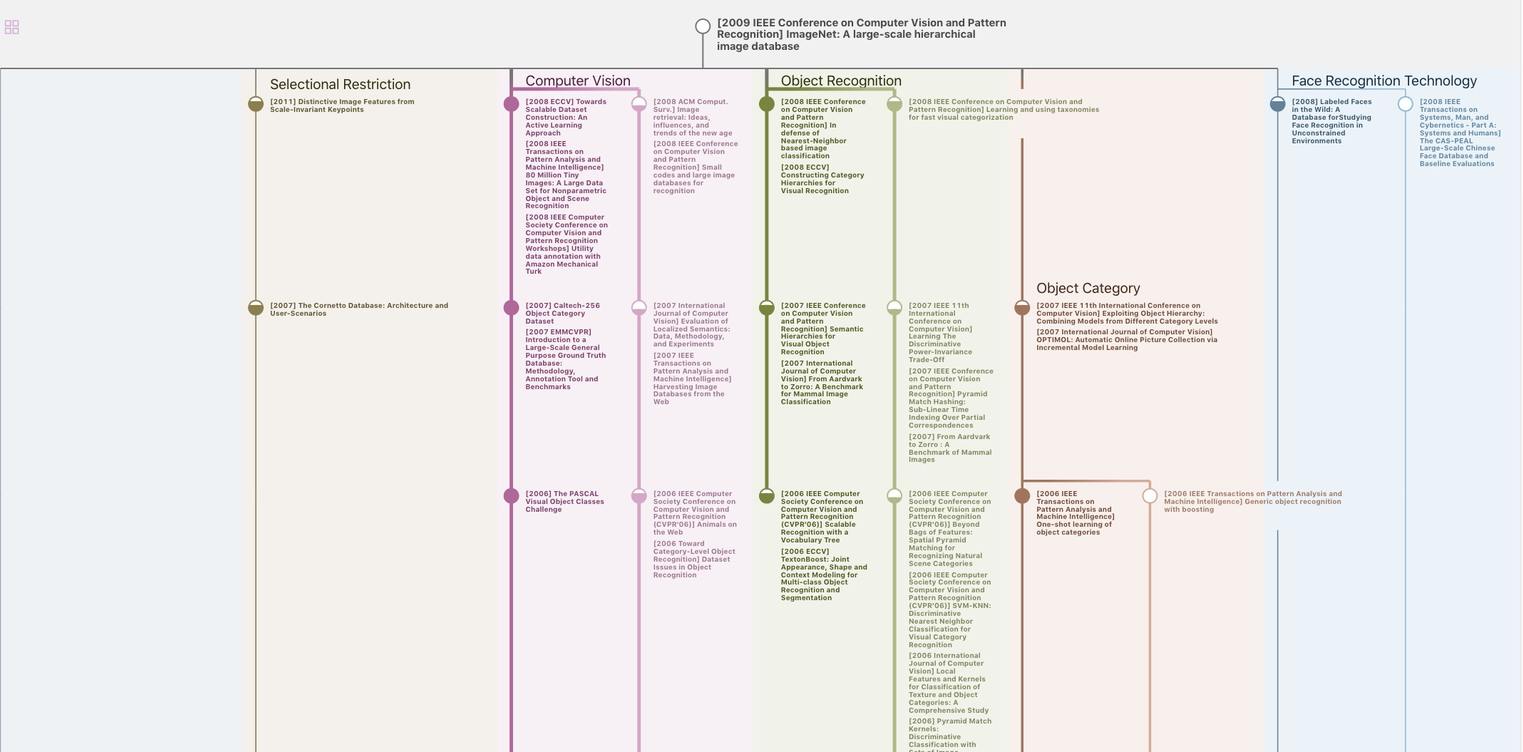
生成溯源树,研究论文发展脉络
Chat Paper
正在生成论文摘要