Self-supervised Human Pose Recovery for Through-wall Radar Based on Convolutional Neural Networks
2022 Photonics & Electromagnetics Research Symposium (PIERS)(2022)
摘要
Through-wall radar (TWR) can penetrate through non-metallic occlusions and detect hidden human targets. Nonetheless, because of its low imaging spatial resolution, most of the current methods can only obtain the low-level human detecting information from the TWR signals, for example, the human trunk positions. More complex human information, such as complete pose outline, has remained intractable. In this paper, a novel self-supervised human pose recovery method for TWR based on convolutional neural networks (CNNs) is proposed. We adopt a self-supervised teacher-student learning pipeline for the method. During training, we attach a camera to the radar to simultaneously collect pairs of RGB images and TWR signals. A vision-based pretrained teacher network extracts human pose information from RGB images and generates the human outline masks as pseudo labels. A student network learns to extract the patterns in corresponding TWR signals and predicts the masks that are close to the labels above. There is no external supervision in the training process, so it is unnecessary to label the dataset manually. After training, the method can recover accurate human pose just from TWR signals. The experiments are conducted in two different scenarios. In a scenario without wall occlusion, we collected synchronized radar signals and images for method training and accuracy evaluation. The quantitative results show comparable predictions with the state-of-the-art methods in non-wallocclusive scenarios. In a wall-occlusive scenario, we solely collect radar signals for generalization evaluation. The accurate qualitative predictions show complete human pose recovery with wall occlusions.
更多查看译文
关键词
convolutional neural networks,RGB imaging,vision-based pretrained teacher network,human outline masks,TWR signals,training process,radar signals,nonwallocclusive scenarios,wall-occlusive scenario,complete human pose recovery,self-supervised human pose recovery,through-wall radar,nonmetallic occlusions,low imaging spatial resolution,low-level human detecting information,human trunk positions,CNN,self-supervised teacher-student learning pipeline
AI 理解论文
溯源树
样例
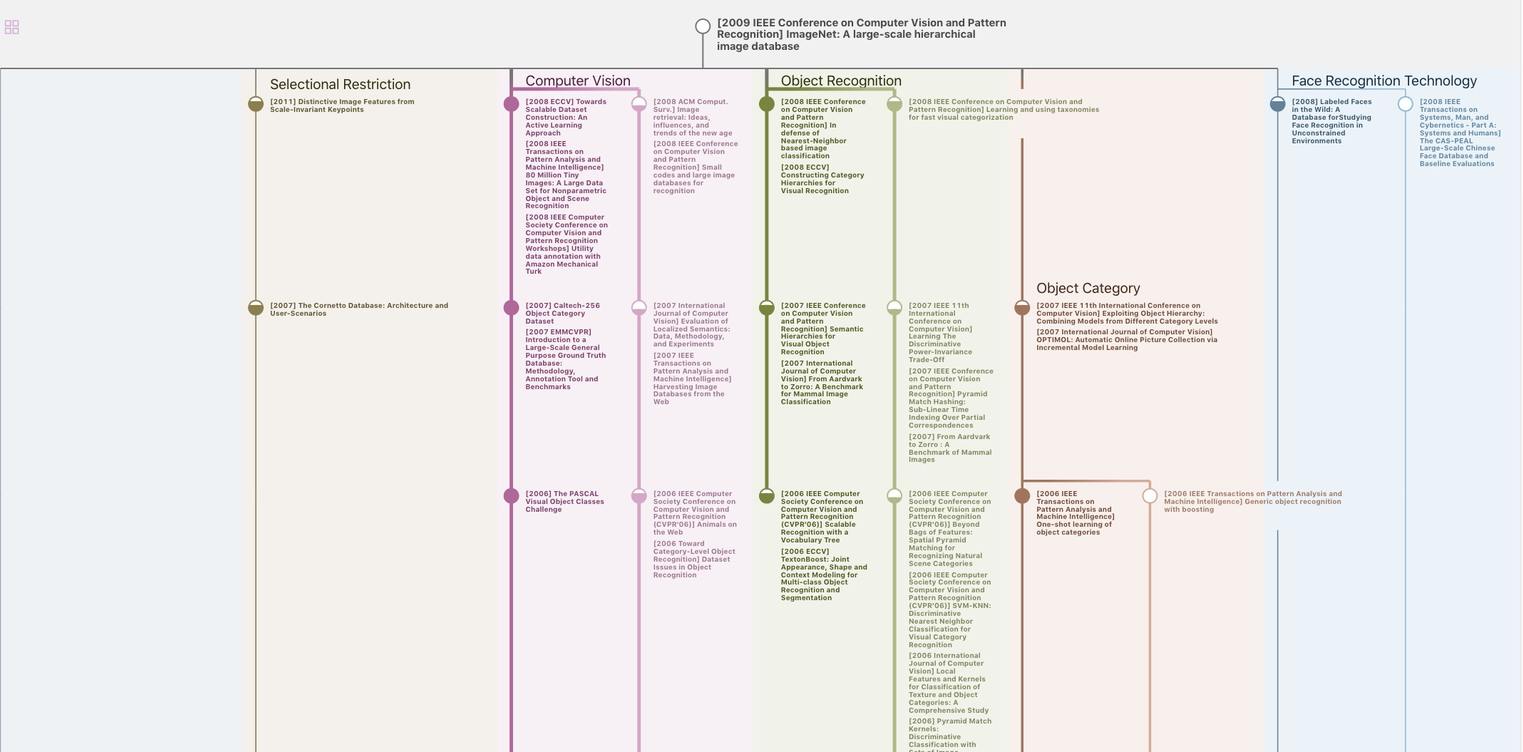
生成溯源树,研究论文发展脉络
Chat Paper
正在生成论文摘要