FPL-UDA: FILTERED PSEUDO LABEL-BASED UNSUPERVISED CROSS-MODALITY ADAPTATION FOR VESTIBULAR SCHWANNOMA SEGMENTATION
2022 IEEE INTERNATIONAL SYMPOSIUM ON BIOMEDICAL IMAGING (IEEE ISBI 2022)(2022)
摘要
Automatic segmentation of Vestibular Schwannoma (VS) from Magnetic Resonance Imaging (MRI) will help patient management and improve clinical workflow. This paper aims to adapt a model trained with annotated ceT1 images to segment VS from hrT2 images, without annotations of the latter. The proposed method is named as Filtered Pseudo Labelbased Unsupervised Domain Adaptation (FPL-UDA) and consists of three components: 1) an image translator converting hrT2 images to pseudo ceT1 images, where a two-stage translation strategy is proposed to deal with images with VS in various sizes, 2) a pseudo label generator trained with ceT1 images to provide pseudo labels for the pseudo ceT1 images, where a GAN-based data augmentation method is proposed to deal with the domain gap between them, and 3) a final segmentor trained with hrT2 images and the corresponding pseudo labels, where an uncertainty-based filtering is used to select high-quality pseudo labels to improve the segmentor's robustness. Experimental results with a public VS dataset showed that our method achieved an average Dice of 81.52% for VS segmentation from hrT2 images, which outperformed existing unsupervised cross-modality adaptation methods.
更多查看译文
关键词
Domain adaption, image translation, uncertainty estimation, vestibular schwannoma, pseudo labels
AI 理解论文
溯源树
样例
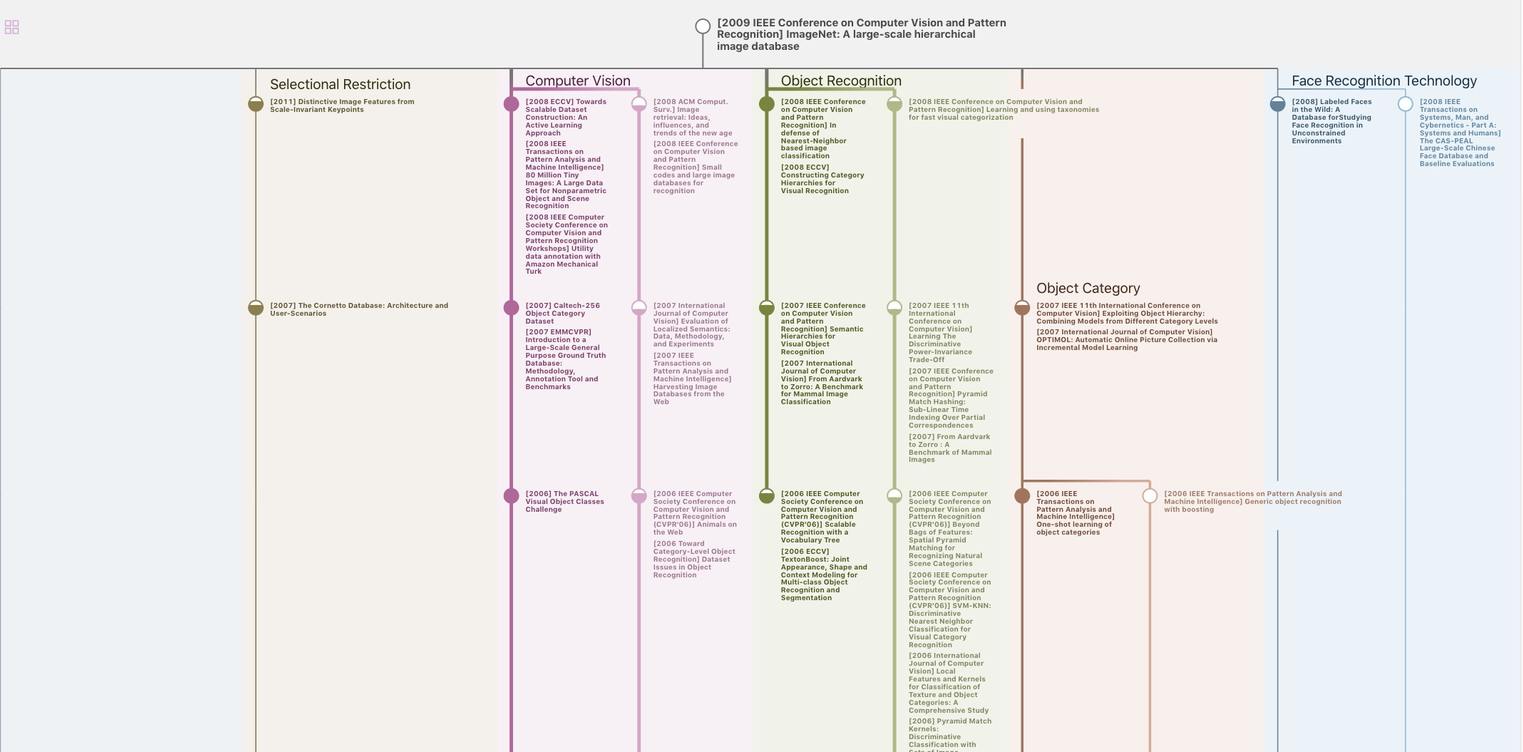
生成溯源树,研究论文发展脉络
Chat Paper
正在生成论文摘要