CATEGORY SEPARATION FOR WEAKLY SUPERVISED MULTI-CLASS CELL COUNTING
2022 IEEE INTERNATIONAL SYMPOSIUM ON BIOMEDICAL IMAGING (IEEE ISBI 2022)(2022)
摘要
Cell quantification in immunohistochemically stained images assists the prognostic evaluation of tumor progression. The development of lighter artificial intelligence-assisted diagnosis models can further improve the efficiency of whole slide image analysis and control training costs. However, recent count-level regression models are limited in the multicategory cell counting task. Therefore, we propose a cellular quantification framework under the count-number supervision by introducing a category-separation learning method, which instructs the model to discriminate differences between various cells. Moreover, a novel loss function is presented to control the representation learning of multi-category cells. In addition, we also validate the performance of Transformerbased architecture. By comparing with learning methods under dots-labeled supervision, the proposed method realize the simultaneous counting of multi-category cells and exhibit competitive results on the public dataset.
更多查看译文
关键词
cell counting, immunohistochemically stained image, weakly supervised learning, count-level regression, Ki-67
AI 理解论文
溯源树
样例
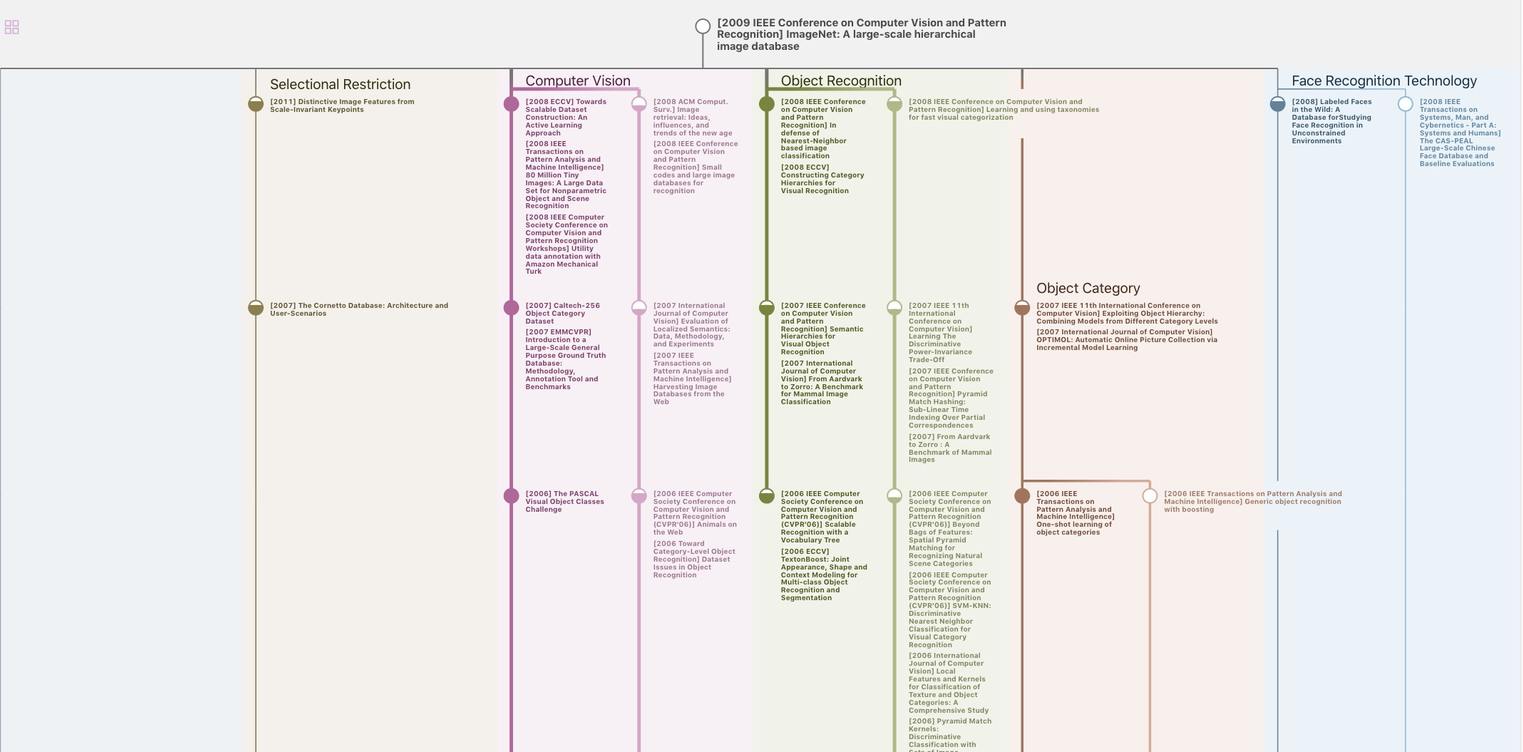
生成溯源树,研究论文发展脉络
Chat Paper
正在生成论文摘要