Investigation of Multi-Cohort Brain MRI Segmentation in Infants
MEDICAL IMAGING 2022: IMAGE PROCESSING(2022)
摘要
Accurate segmentation of infant brain magnetic resonance (MR) images into different tissue types is crucial for studying brain developmental patterns and morphological changes in early life. However, due to the fast maturation speed, MR images scanned at different ages even within a narrow range (such as newborn and around 6-month-old) show drastic differences in size and contrast, which make it challenging for accurate segmentation of infant brain images and generalizing to multi-cohorts likely including infants with different ages and acquisition protocols. Deep learning-based methods haven been shown to achieve state-of-the-art performance among infants aged 6-9 months old, with little contrast between gray and white matter. However, little effort has been devoted to investigate whether these methods are generalizable to successfully segment brain data in infants with other ages, such as newborn babies. Here, we investigated the accuracy and generalizability of deep-learning based methods evaluated in iSeg-2017 and iSeg-2019. We utilized iSeg-2017 dataset and 15 UNC newborn infant brain dataset (UNC_15) to train and test three deep-learning methods: SemiDenseNet, HyperDenseNet, 3D-DenseSeg. Experimental results demonstrate that HyperDenseNet and 3D-DenseSeg have better segmentation performance and HyperDenseNet shows higher generalizability, i.e., producing more accurate results when the testing and training data were from different cohorts. Moreover, we built a joint segmentation-registration method based on DeepAtlas by applying HyperDenseNet for segmentation and VoxelMorph for registration, and used UNC_15 to train the joint network. Results show that the joint segmentation-registration produced better or comparable performance compared with using only registration or segmentation separately.
更多查看译文
关键词
Infant brain,Segmentation,Isointense stage,Deep-learning,Registration
AI 理解论文
溯源树
样例
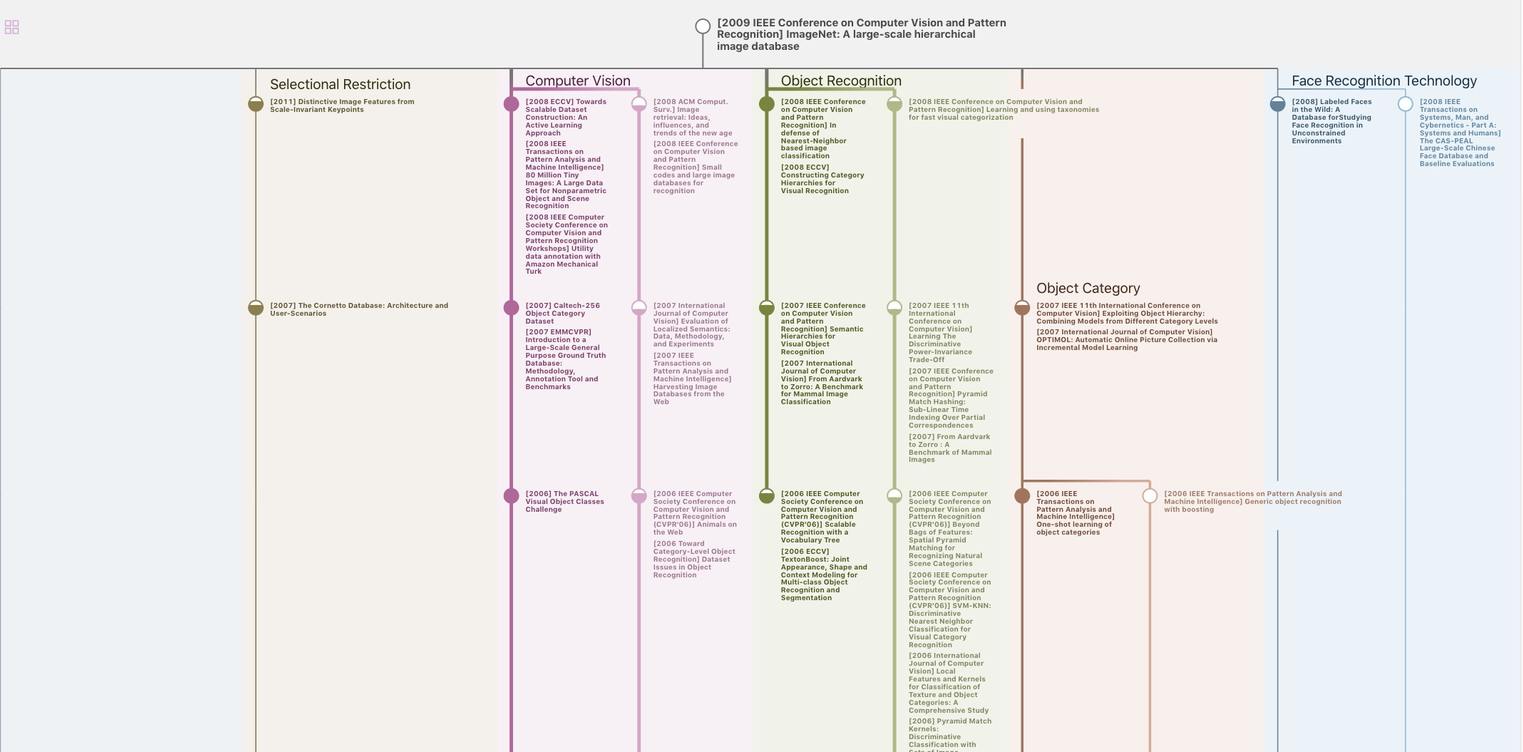
生成溯源树,研究论文发展脉络
Chat Paper
正在生成论文摘要