Evaluating Neural Network Robustness for Melanoma Classification using Mutual Information
MEDICAL IMAGING 2022: IMAGE PROCESSING(2022)
摘要
Deep Neural Networks (DNN) have made rapid progress in medical image analysis, e.g., recent literature has proposed melanoma classifiers that meet or exceed the performance of dermatologists. However, DNNs are typically black box systems and their deployment for safety-critical tasks, such as medical applications, is challenging without methods to characterize and identify the robustness of DNNs in a generalized setting. DNNs are known to be sensitive to small changes in input data, and may rely on spurious correlations seen in the training samples which precludes them from generalizing to data with a different distribution. We previously proposed an attribute-ranking algorithm that computes how informative discrete data attributes are about the DNN performance using the Mutual Information. In this study we leverage this algorithm in a novel way to determine if the data attributes that impact the predictions made by the DNN are clinically relevant and thereby whether the DNN predictions are robust. We demonstrate the applicability of this method on melanoma classification with a DNN trained on the publicly available HAM 10,000 dataset and achieve 0.852 AUC on the held-out HAM test set. Our analysis identifies that image saturation, not a clinically relevant feature, is highly indicative of both whether an image is melanomic in the HAM training data and whether the DNN prediction is correct. Further testing reveals that when the classifier is tested on the SIIM-ISIC Melanoma dataset, where the correlation between image saturation and melanoma is not present, the classifier achieves only an AUC of 0.599, confirming non-robustness of the DNN.
更多查看译文
关键词
Robust Neural Networks,Melanoma Classification,Deep Learning,Dataset Shift
AI 理解论文
溯源树
样例
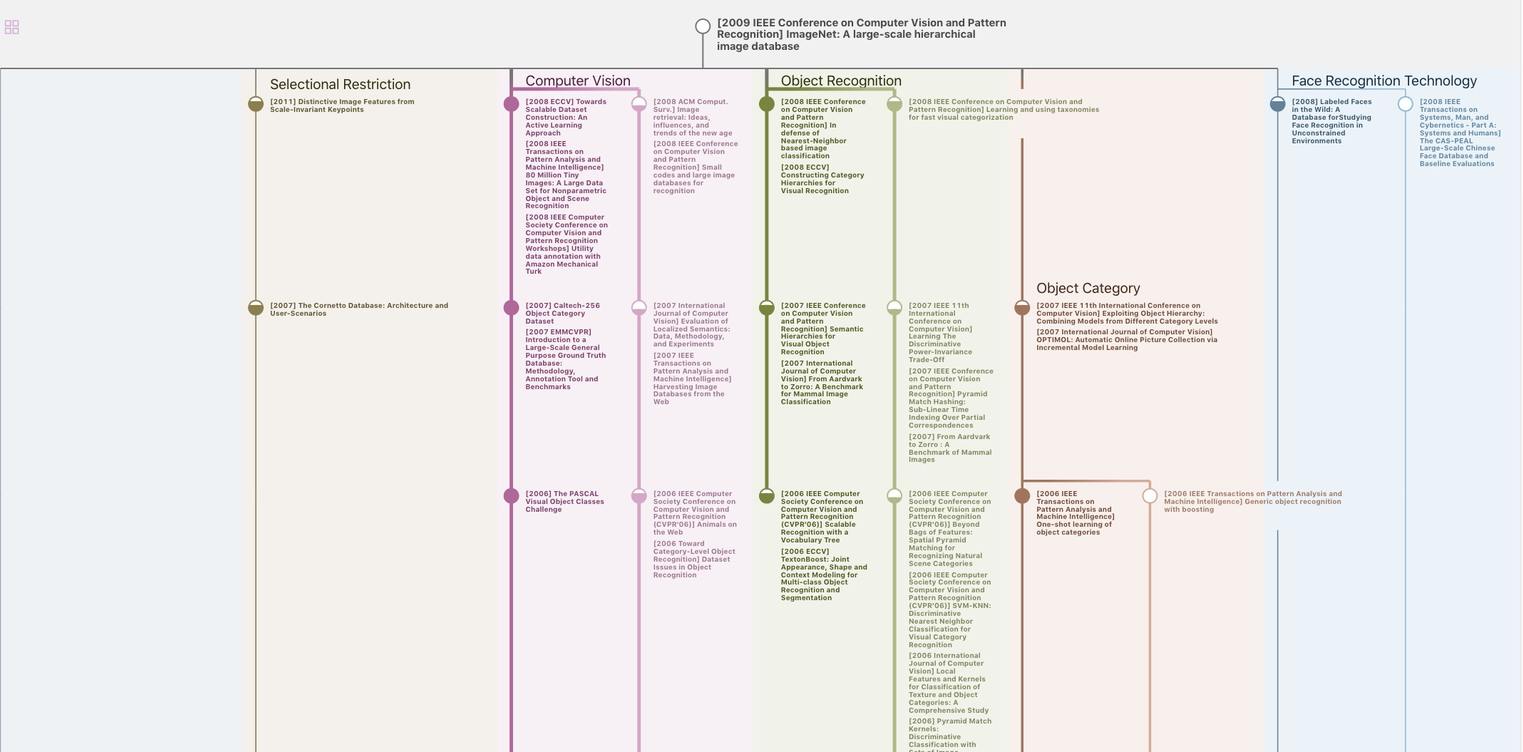
生成溯源树,研究论文发展脉络
Chat Paper
正在生成论文摘要