ATTENTION-BASED NOISE PRIOR NETWORK FOR MAGNETIC RESONANCE IMAGE DENOISING
2022 IEEE INTERNATIONAL SYMPOSIUM ON BIOMEDICAL IMAGING (IEEE ISBI 2022)(2022)
摘要
Data obtained from magnitude magnetic resonance images (MRI) are corrupted by signal-dependent and spatially variant Rician noise. The contribution of this work can be discussed at three different levels. Firstly, Rician noise-level estimation map is fed as prior concatenated with the noisy input data; to handle spatially variant noise and achieve an optimal compromise between noise removal and detail preservation. Secondly, modified U-Net architecture is used to accommodate non-local multi-level and multi-scale features. Thirdly, to preserve the long-range dependencies lost in farther symmetric layers, features obtained from symmetric-group attention block is fed as input to the deconvolution layers for nonlocal high- and low-level feature mapping. Experimental results over synthetically corrupted MR images and real data obtained from MR scanners suggest the potential utility of our proposed technique over a wide-range of noise levels.
更多查看译文
关键词
Attention network, magnetic resonance imaging, noise level estimation, Rician distribution
AI 理解论文
溯源树
样例
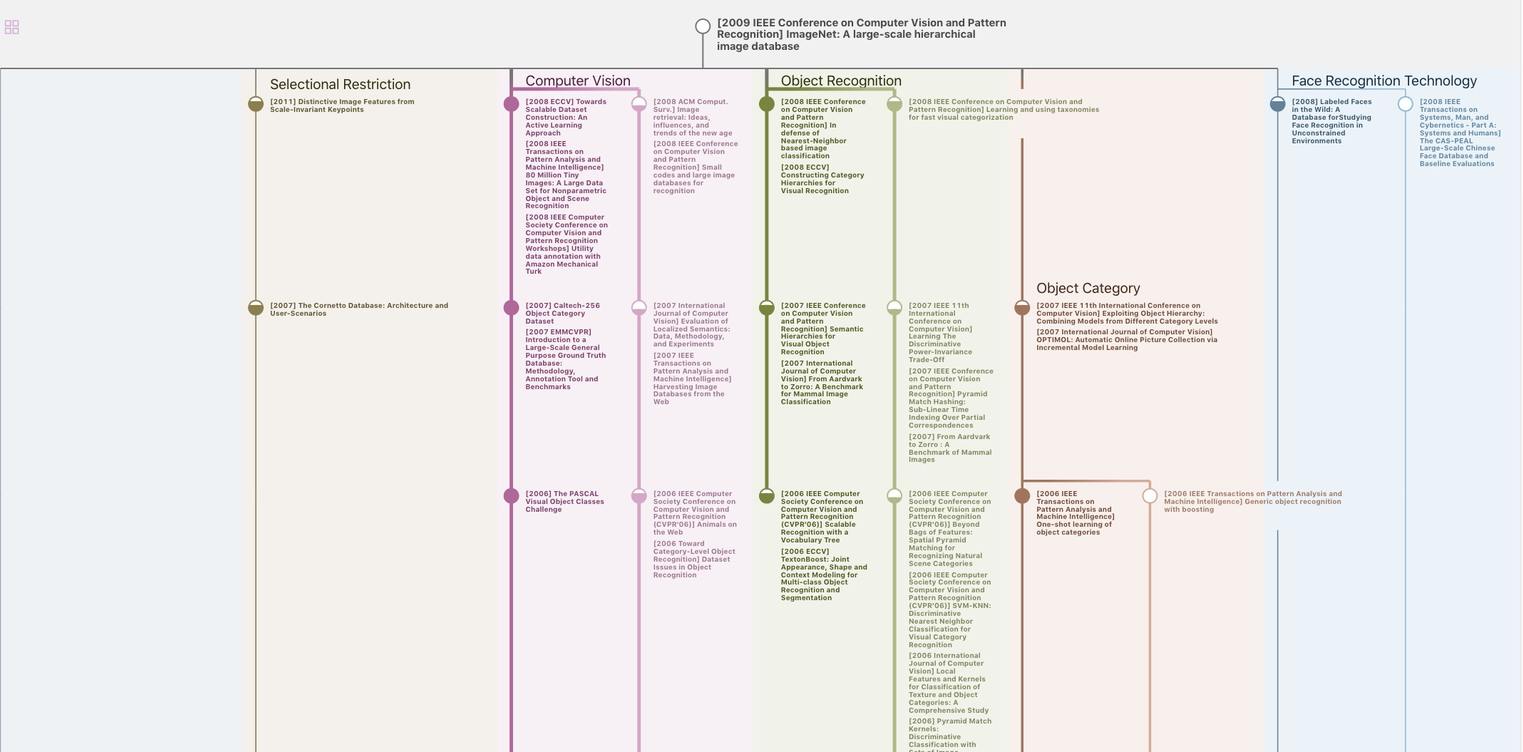
生成溯源树,研究论文发展脉络
Chat Paper
正在生成论文摘要