Wavelet ELM-AE Based Data Augmentation and Deep Learning for Efficient Emotion Recognition Using EEG Recordings
IEEE ACCESS(2022)
摘要
Emotion perception is critical for behavior prediction. There are many ways to capture emotional states by observing the body and copying actions. Physiological markers such as electroencephalography (EEG) have gained popularity, as facial emotions may not always adequately convey true emotion. This study has two main aims. The first is to measure four emotion categories using deep learning architectures and EEG data. The second purpose is to increase the number of samples in the dataset. To this end, a novel data augmentation approach namely the Extreme Learning Machine Wavelet Auto Encoder (ELM-W-AE) is proposed for data augmentation. The proposed data augmentation approach is both simple and faster than the other synthetic data augmentation approaches. For deep architectures, large datasets are important for performance. For this reason, data multiplexing approaches with classical and synthetic methods have become popular recently. The proposed synthetic data augmentation is the ELM-W-AE because of its efficiency and detail reproduction. The ELM-AE structure uses wavelet activation functions such as Gaussian, groove gap waveguide (GGW), Mexican, Meyer, Morlet, and Shannon. Deep convolutional architectures classify EEG signals as images. EEG waves are scalograms using Continuous Wavelet Transform (CWT). The ResNet18 architecture recognizes emotions. The proposed technique uses GAMEEMO data collected during gameplay. Each of these states is represented in the GAMEEMO data collection. The visual data set created from the signal was divided into two groups 70% training and 30% testing. ResNet18 has been fine-tuned with augmented photos, training images only. It achieved 99.6% classification accuracy in tests. The proposed method is compared with the other approaches on the same dataset, and an approximately 22% performance improvement is achieved.
更多查看译文
关键词
Electroencephalography, Brain modeling, Emotion recognition, Feature extraction, Support vector machines, Deep learning, Data models, Auto-encoder, data augmentation, deep learning, emotion recognition, emotion measurement
AI 理解论文
溯源树
样例
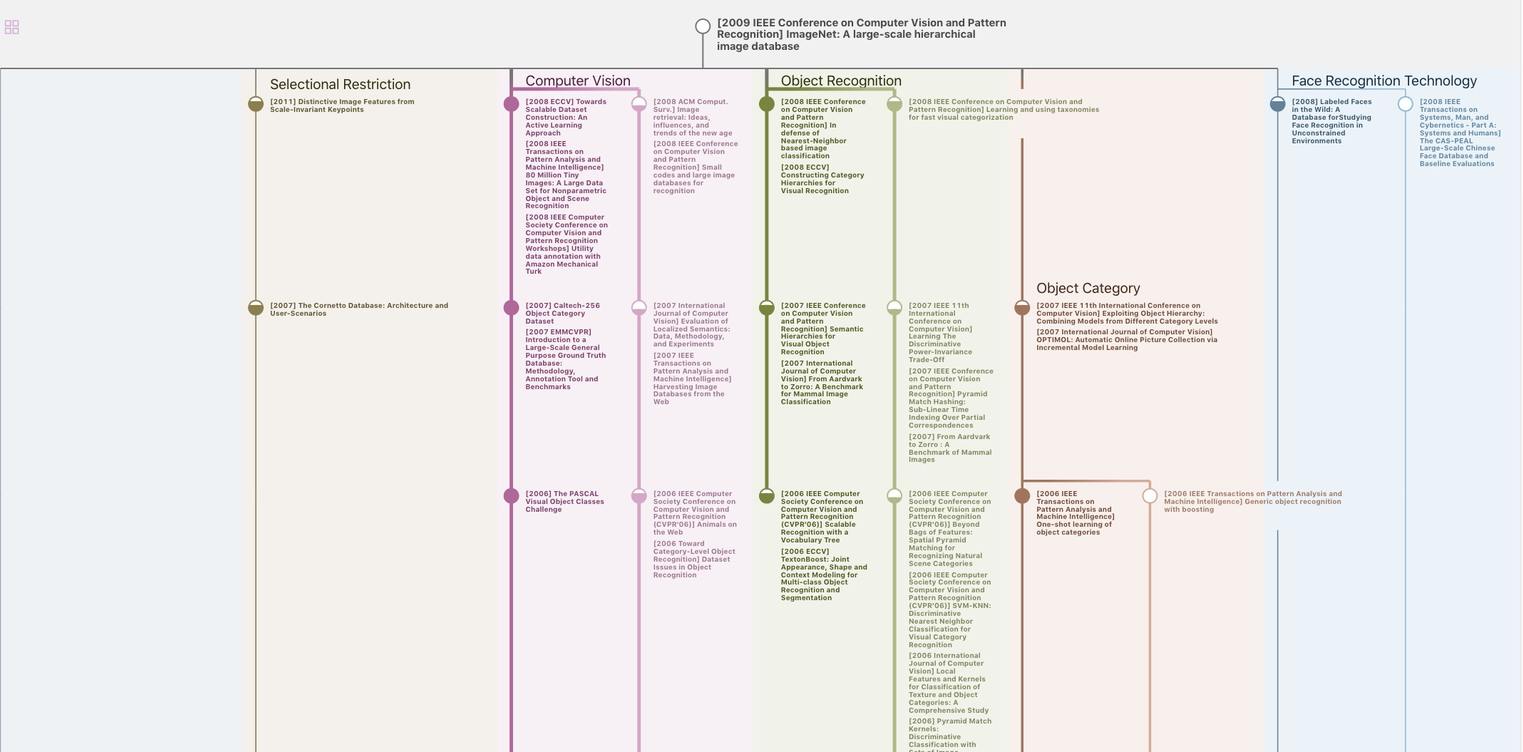
生成溯源树,研究论文发展脉络
Chat Paper
正在生成论文摘要