Source Term Estimation Using Deep Reinforcement Learning With Gaussian Mixture Model Feature Extraction for Mobile Sensors
IEEE ROBOTICS AND AUTOMATION LETTERS(2022)
摘要
This paper proposes a deep reinforcement learning method for mobile sensors to estimate the properties of the source of the hazardous gas release. The problem of estimating the properties of the released gas is generally termed as the source term estimation (STE) problem. Since the sensor measurements from atmospheric gas dispersion are sparse, intermittent, and time-varying due to the turbulence and the sensor noise, STE is considered to be a challenging problem. The particle filter is adopted to estimate the source term under such stochastic noise conditions. The deep deterministic policy gradient (DDPG) is also employed to find the best source search policy in terms of successful estimation and traveled distance. Through ablation studies, we demonstrate that the use of the Gaussian mixture model, which clusters the potential source positions from the particle filter, as an input to the DDPG and the gated recurrent unit functioning as a memory in DDPG help to improve the STE performance. Besides, simulation results in randomized source term conditions and previously-unseen environments show the superior STE performance of the proposed algorithm compared with the existing information-theoretic STE algorithm.
更多查看译文
关键词
Autonomous agents, machine learning for robot control, motion and path planning, reinforcement learning, robotics in hazardous fields
AI 理解论文
溯源树
样例
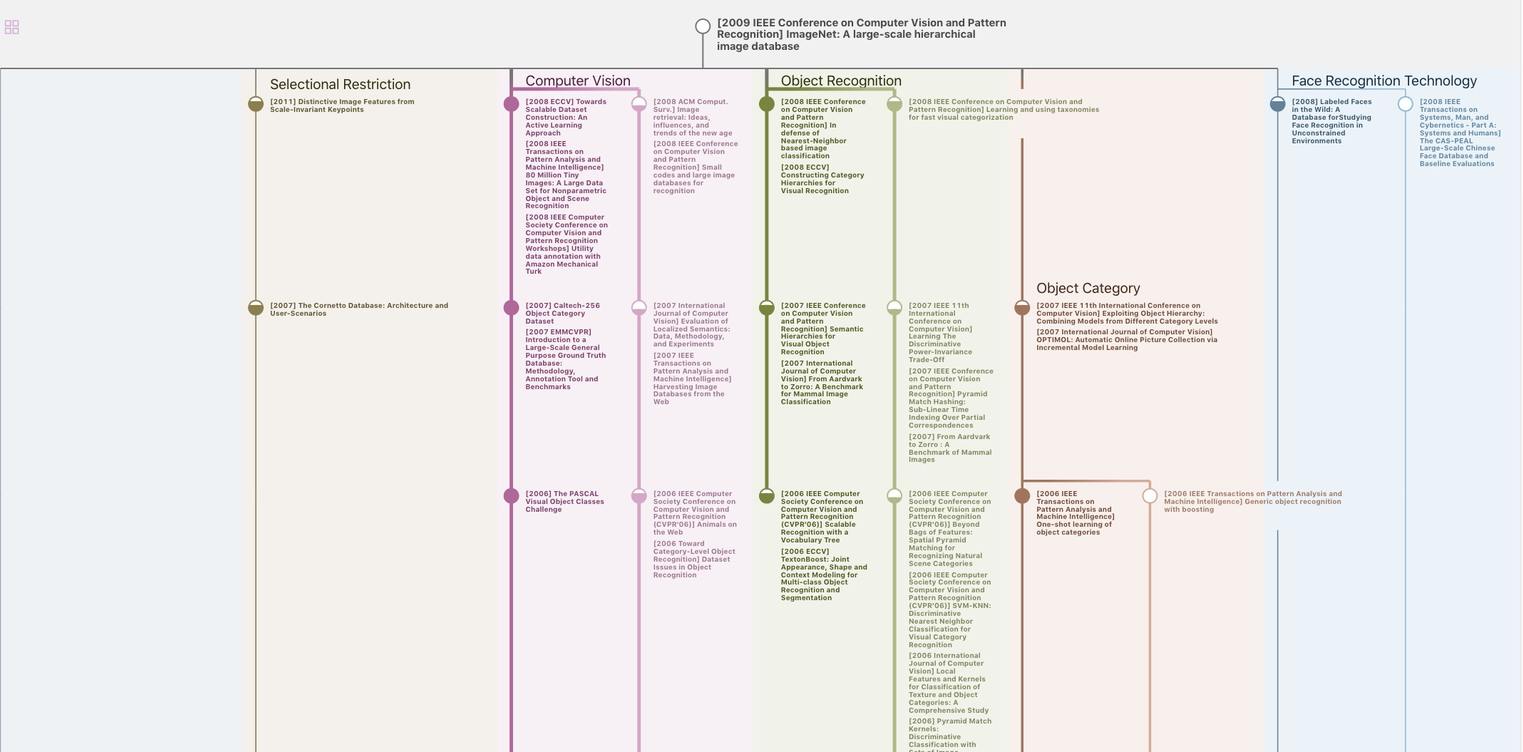
生成溯源树,研究论文发展脉络
Chat Paper
正在生成论文摘要