Risk-Adapted Optimal Experimental Design
SIAM-ASA JOURNAL ON UNCERTAINTY QUANTIFICATION(2022)
摘要
Constructing accurate statistical models of critical system responses typically requires an enormous amount of experimental data. Unfortunately, physical experimentation is often expensive and time consuming. Optimal experimental design determines the "best" allocation of experiments with respect to a criterion that measures the ability to estimate some important aspect of an assumed statistical model. While optimal design has a vast literature, few researchers have developed design paradigms targeting tail statistics, such as quantiles. In this paper, we introduce a new optimality criterion, R-optimality, that attempts to minimize the risk associated with large prediction variances. The R-optimality criterion generalizes the classical I-and G-optimality criteria and can be tailored to the risk preferences of stakeholders. We discuss numerical methods for the case when the design is supported on a finite number of points. This happens if there are only finitely many experimental configurations or if the design space is discretized. In the latter case, we prove consistency of the approximation as the number of design points increases. We demonstrate the R-optimality criterion on various numerical examples, including the calibration of a polynomial model using least-squares and quantile regression, the calibration of the nonlinear Michaelis-Menten model, and microphone placement for direct field acoustic testing-a technique used to test engineered structures in vibration environments by subjecting them to intense acoustic pressure.
更多查看译文
关键词
experimental design, optimal design, average value-at-risk, conditional value-at-risk, risk measures, distributionally robust optimization, R-optimality, statistical estimation
AI 理解论文
溯源树
样例
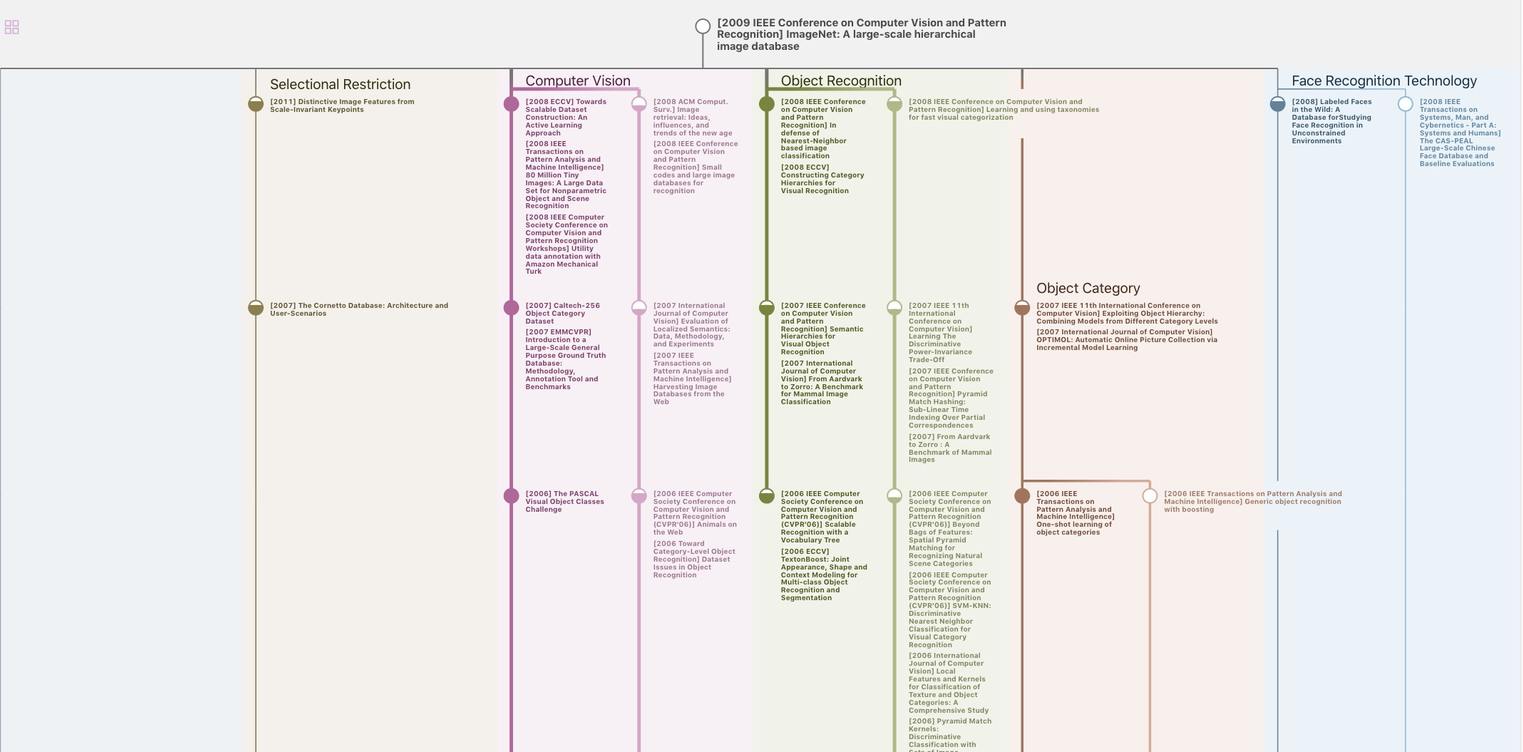
生成溯源树,研究论文发展脉络
Chat Paper
正在生成论文摘要