Low Tucker rank tensor completion using a symmetric block coordinate descent method
NUMERICAL LINEAR ALGEBRA WITH APPLICATIONS(2023)
摘要
Low Tucker rank tensor completion has wide applications in science and engineering. Many existing approaches dealt with the Tucker rank by unfolding matrix rank. However, unfolding a tensor to a matrix would destroy the data's original multi-way structure, resulting in vital information loss and degraded performance. In this article, we establish a relationship between the Tucker ranks and the ranks of the factor matrices in Tucker decomposition. Then, we reformulate the low Tucker rank tensor completion problem as a multilinear low rank matrix completion problem. For the reformulated problem, a symmetric block coordinate descent method is customized. For each matrix rank minimization subproblem, the classical truncated nuclear norm minimization is adopted. Furthermore, temporal characteristics in image and video data are introduced to such a model, which benefits the performance of the method. Numerical simulations illustrate the efficiency of our proposed models and methods.
更多查看译文
关键词
block coordinate descent, sparsity, tensor completion, truncated nuclear norm, Tucker rank
AI 理解论文
溯源树
样例
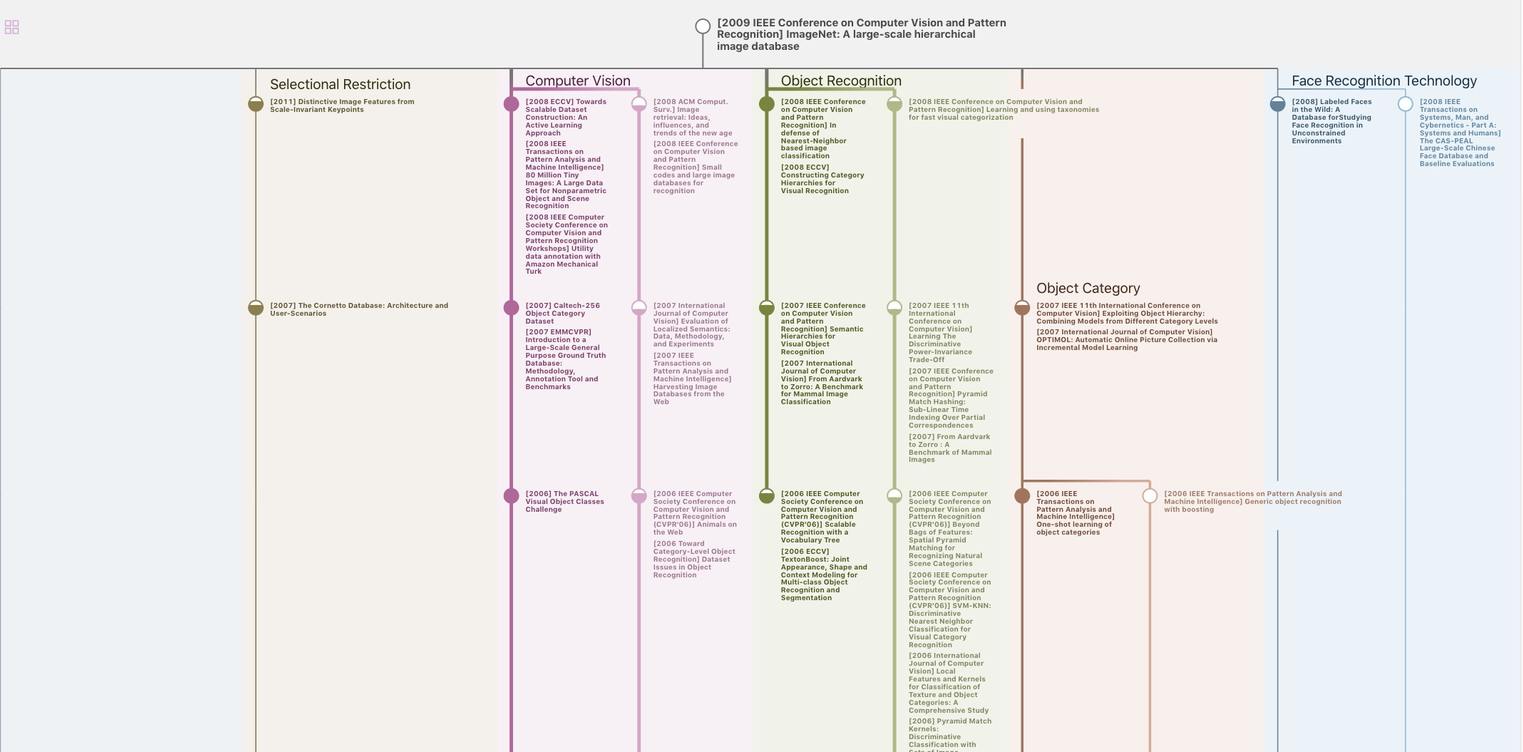
生成溯源树,研究论文发展脉络
Chat Paper
正在生成论文摘要