Importance of Pre-Storm Morphological Factors in Determination of Coastal Highway Vulnerability
JOURNAL OF MARINE SCIENCE AND ENGINEERING(2022)
摘要
This work considers a database of pre-storm morphological factors and documented impacts along a coastal roadway. Impacts from seven storms, including sand overwash and pavement damage, were documented via aerial photography. Pre-storm topography was examined to parameterize the pre-storm morphological factors likely to control whether stormwater levels and waves impact the road. Two machine learning techniques, K-nearest neighbors (KNN) and ensemble of decision trees (EDT), were employed to identify the most critical pre-storm morphological factors in determining the road vulnerability, expressed as a binary variable to impact storms. Pre-processing analysis was conducted with a correlation analysis of the predictors' data set and feature selection subroutine for the KNN classifier. The EDTs were built directly from the data set, and feature importance estimates were reported for all storm events. Both classifiers report the distances from roadway edge-of-pavement to the dune toe and ocean as the most important predictors of most storms. For storms approaching from the bayside, the width of the barrier island was the second most important factor. Other factors of importance included elevation of the dune toe, distance from the edge of pavement to the ocean shoreline, shoreline orientation (relative to predominant wave angle), and beach slope. Compared to previously reported optimization techniques, both machine learning methods improved using pre-storm morphological data to classify highway vulnerability based on storm impacts.
更多查看译文
关键词
coastal roadways, vulnerability, morphological indicators, machine learning, management, water resources
AI 理解论文
溯源树
样例
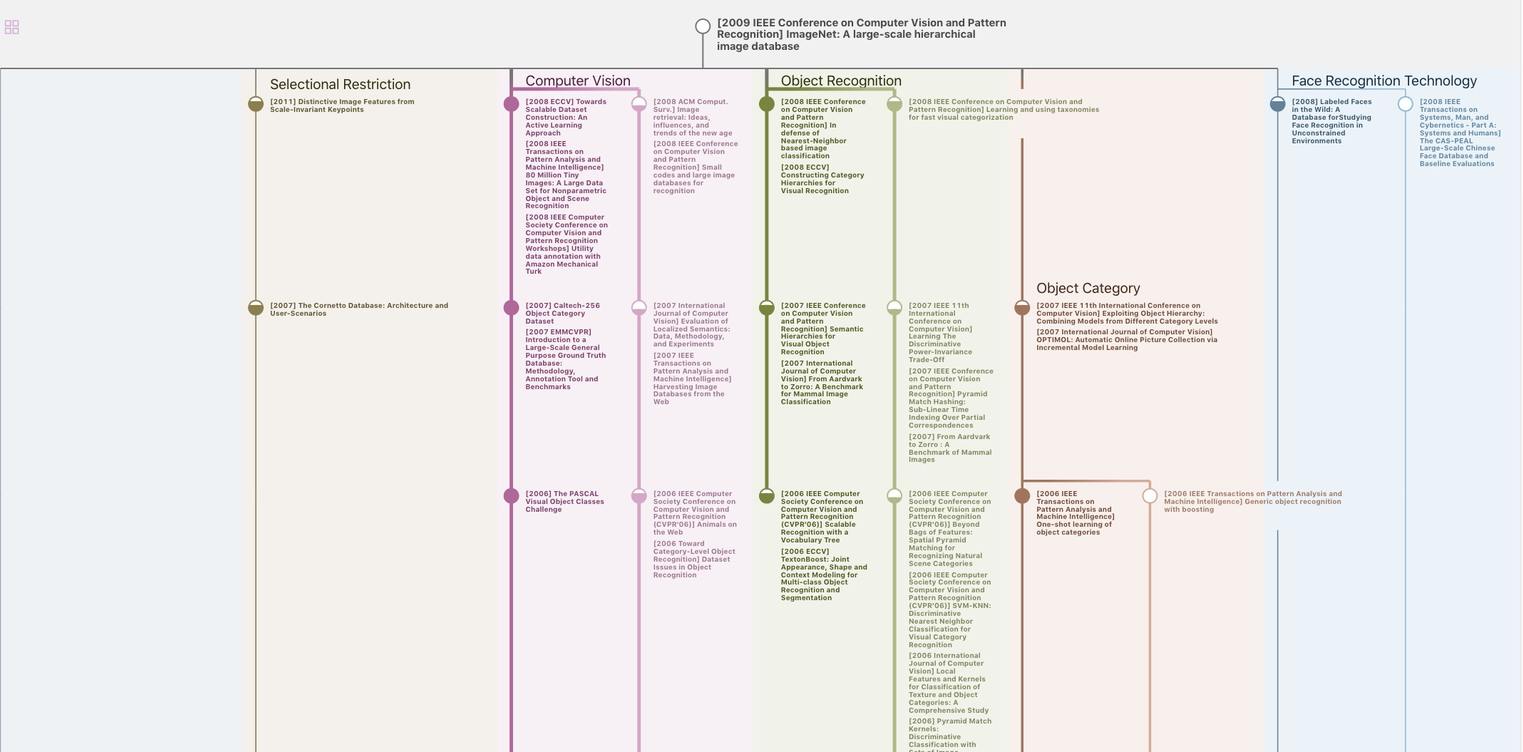
生成溯源树,研究论文发展脉络
Chat Paper
正在生成论文摘要