Gathering Strength, Gathering Storms: Knowledge Transfer via Selection for VRPTW
MATHEMATICS(2022)
摘要
Recently, due to the growth in machine learning and data mining, for scheduling applications in China's industrial intelligence, we are quite fortunate to witness a paradigm of evolutionary scheduling via learning, which includes a new tool of evolutionary transfer optimization (ETO). As a new subset in ETO, single-objective to multi-objective/many-objective optimization (SMO) acts as a powerful, abstract and general framework with wide industrial applications like shop scheduling and vehicle routing. In this paper, we focus on the general mechanism of selection that selects or gathers elite and high potential solutions towards gathering/transferring strength from single-objective problems, or gathering/transferring storms of knowledge from solved tasks. Extensive studies in vehicle routing problems with time windows (VRPTW) on well-studied benchmarks validate the great universality of the SMO framework. Our investigations (1) contribute to a deep understanding of SMO, (2) enrich the classical and fundamental theory of building blocks for genetic algorithms and memetic algorithms, and (3) provide a completive and potential solution for VRPTW.
更多查看译文
关键词
evolutionary transfer optimization, green scheduling, transfer learning, data analytics, system optimization, carbon neutrality
AI 理解论文
溯源树
样例
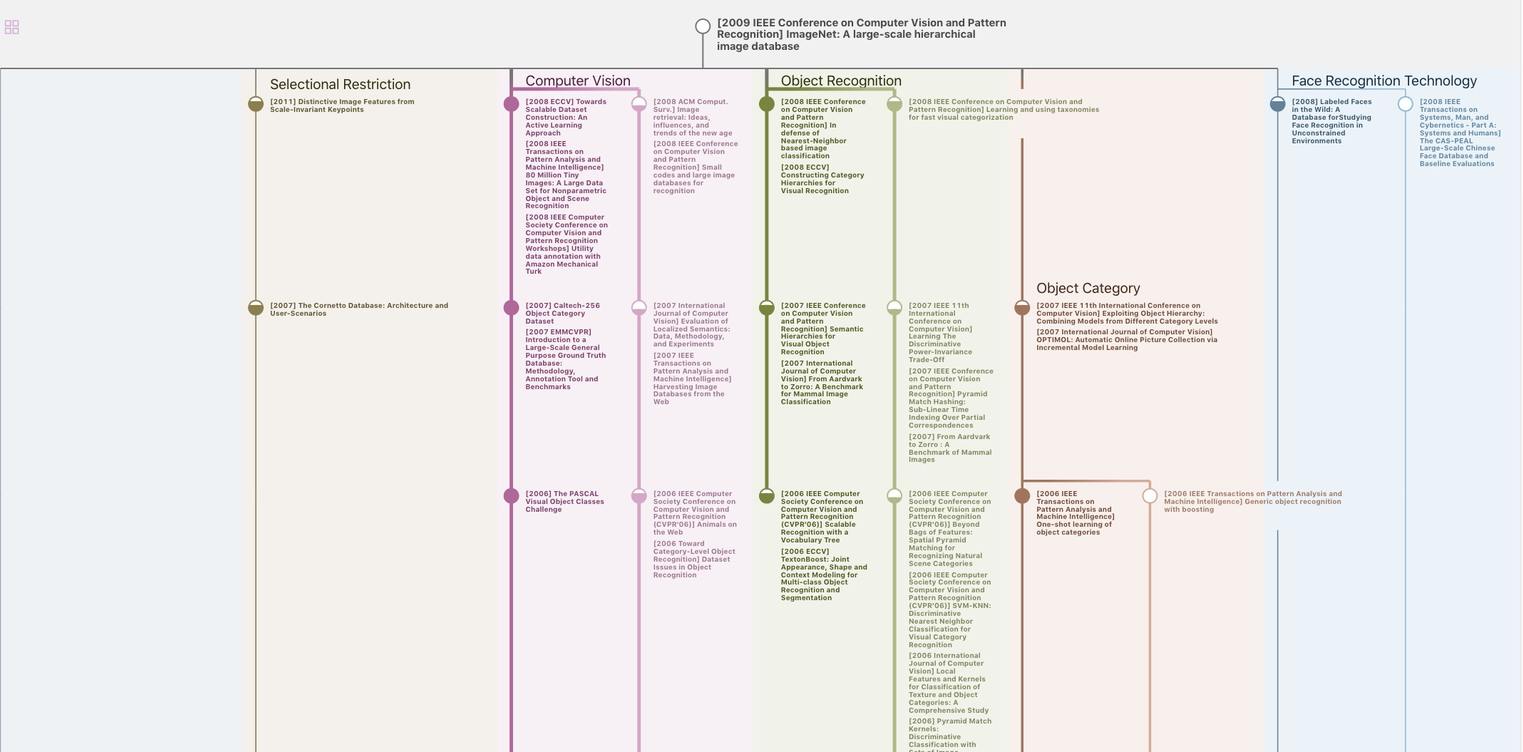
生成溯源树,研究论文发展脉络
Chat Paper
正在生成论文摘要