Efficiency and Fairness Oriented Dynamic Task Offloading in Internet of Vehicles
IEEE Transactions on Green Communications and Networking(2022)
摘要
The rapid development of the Internet of Vehicles (IoV) leads to various on-board applications including delay-sensitive and compute-intensive applications. However, vehicles with limited computing resources cannot meet the requirements of the delay for these applications. The application of edge computing on the IoV can deal with the above problems. However, most existing edge computing offloading work only considers static scenarios. These schemes are difficult to adapt to dynamic processes under continuous time. To solve this problem, in this article, we propose an end-edge-cloud architecture of vehicles for task computation offloading, where considers three task computing methods. For the dynamically changing environment in the IoV, we utilize an Asynchronous Advantage Actor-Critic (A3C) based computation offloading algorithm to solve the problem and seek optimal offloading decisions. While considering efficiency and fairness, our solution enables vehicle users to obtain computing services from edge servers in real-time and conveniently. The reward function in this paper contains a relative efficiency factor and a relative fairness factor, which are indicators to measure the efficiency of task completion as well as the relative fairness between vehicles. These indicators can be adjusted to obtain more targeted offloading decisions in different scenarios. The experimental results show that the scheme proposed in this paper can achieve good convergence performance and adapt to the dynamic offloading scene. Compared with the other scheme, our scheme can achieve better performance in terms of efficiency of task completion and fairness among tasks. The numerical results show that compared to the Deep Q Network-based scheme, the local computing scheme, the edge computing scheme, and the random scheme, our algorithm can save 7.2%, 18.5%, 41.8%, and 33.5% of the mean for average delay, respectively.
更多查看译文
关键词
Internet of Vehicles,mobile edge computing,reinforcement learning
AI 理解论文
溯源树
样例
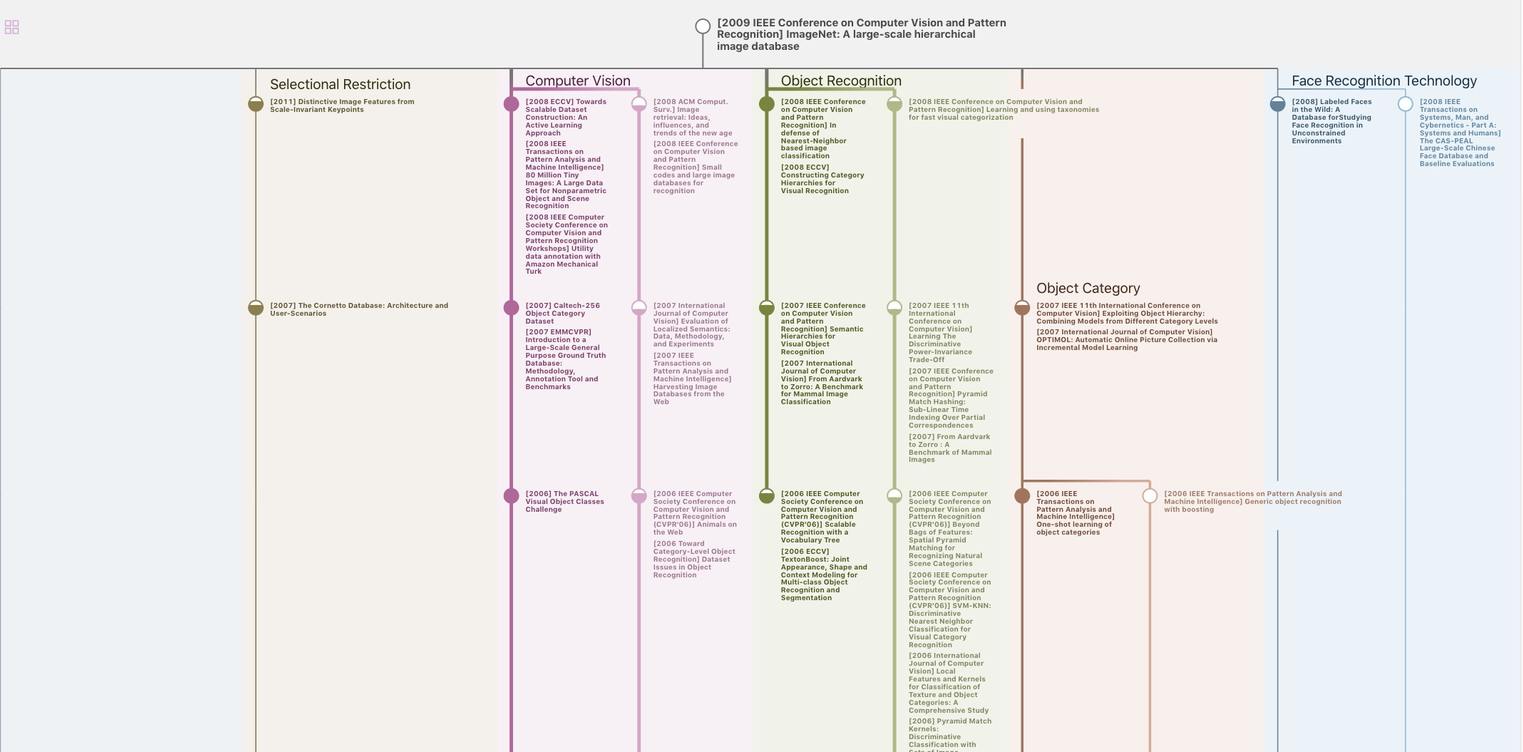
生成溯源树,研究论文发展脉络
Chat Paper
正在生成论文摘要