A Lyapunov Approach for Stable Reinforcement Learning.
Computational & Applied Mathematics(2022)
摘要
Our strategy is based on a novel reinforcement-learning (RL) Lyapunov methodology. We propose a method for constructing Lyapunov-like functions using a feed-forward Markov decision process. These functions are important for assuring the stability of a behavior policy throughout the learning process. We show that the cost sequence, which corresponds to the best approach, is frequently non-monotonic, implying that convergence cannot be guaranteed. For any Markov-ergodic process, our technique generates a Lyapunov-like function, implying an one-to-one correspondence between the present cost-function and the suggested function, resulting in a monotonically non-increase behavior on the trajectories under optimum strategy realization. We show that the system’s dynamics and trajectory converge. We show how to employ the Lyapunov technique to solve RL problems. We explain how to employ the Lyapunov method to RL. We test the proposed approach to demonstrate its efficacy.
更多查看译文
关键词
Reinforcement learning,Lyapunov,Architecture,Average cost,Markov chains,Optimization
AI 理解论文
溯源树
样例
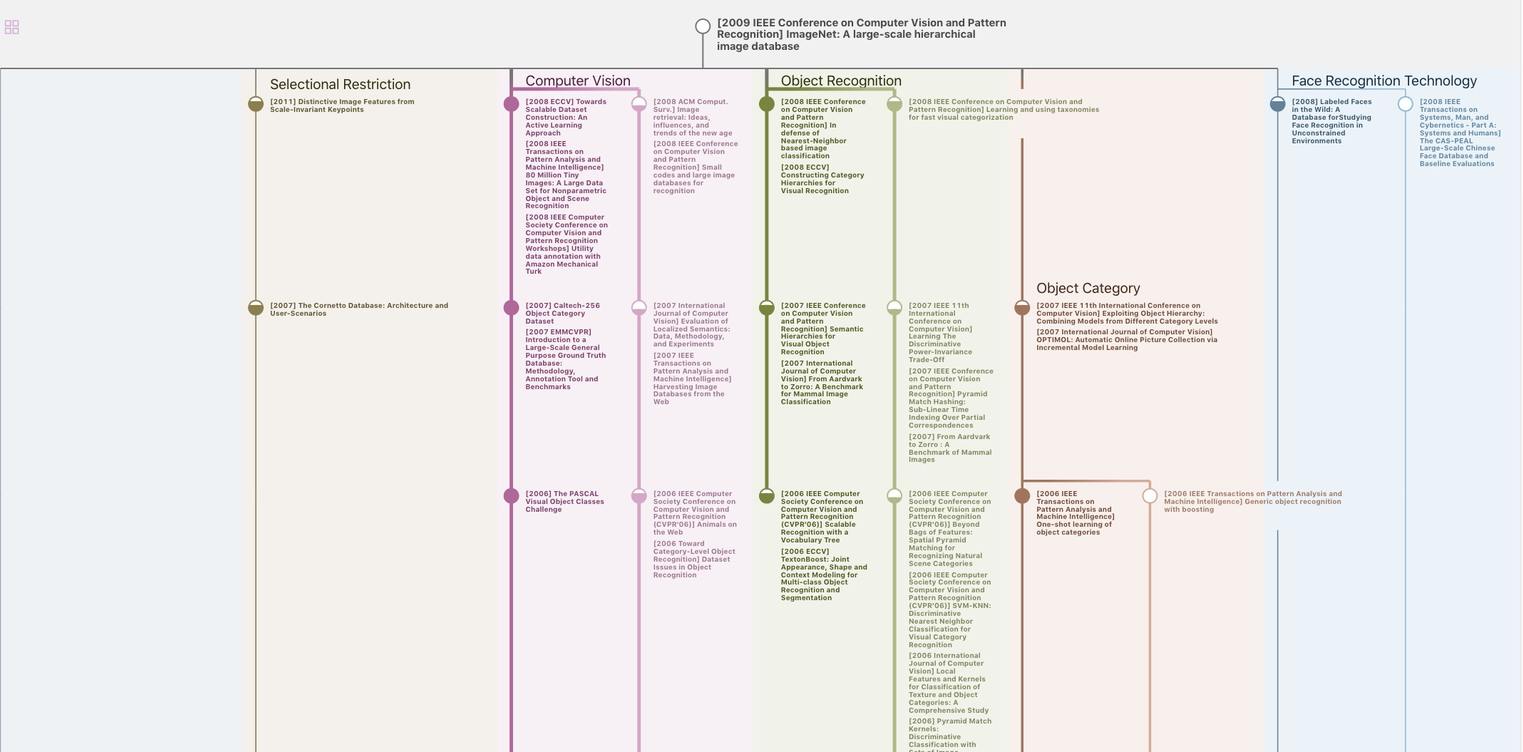
生成溯源树,研究论文发展脉络
Chat Paper
正在生成论文摘要