Progressive-encoding-based transmission for DNN-enabled edge intelligence in unreliable network
Theoretical Computer Science(2022)
摘要
Edge intelligence is getting in the spotlight to enable time-critical applications with the support of next-generation mobile networks. In edge intelligence, real-time image classification and object detection services powered by deep neural networks (DNN) are expected to play an important part. However, signal attenuation and device mobility inevitably lead to unreliability in mobile networks. Additionally, most services adopt transport protocols with fewer error correction mechanisms like user datagram protocol (UDP) to guarantee low latency. These two factors result in data perturbation in mobile networks, which further causes degradation in service reliability. In this paper, we aim to retain high reliability in such scenarios by minimizing the impact of distortion. When researching into image compression, our experiment in the frequency domain reflects that low-frequency coefficients in images are more significant in DNN inference. Progressive encoding provides higher priority to the low-frequency components therefore it is likely to help realize more reliable edge intelligence. We propose a novel transmission process based on progressive encoding. To test its effectiveness compared to other processes, we conduct experiment on state-of-the-art inference models in object detection and image classification. The proposed process reaches a considerable result in normalized reliability in unreliable networks.
更多查看译文
关键词
Edge intelligence,DNN,Reliability,Progressive encoding
AI 理解论文
溯源树
样例
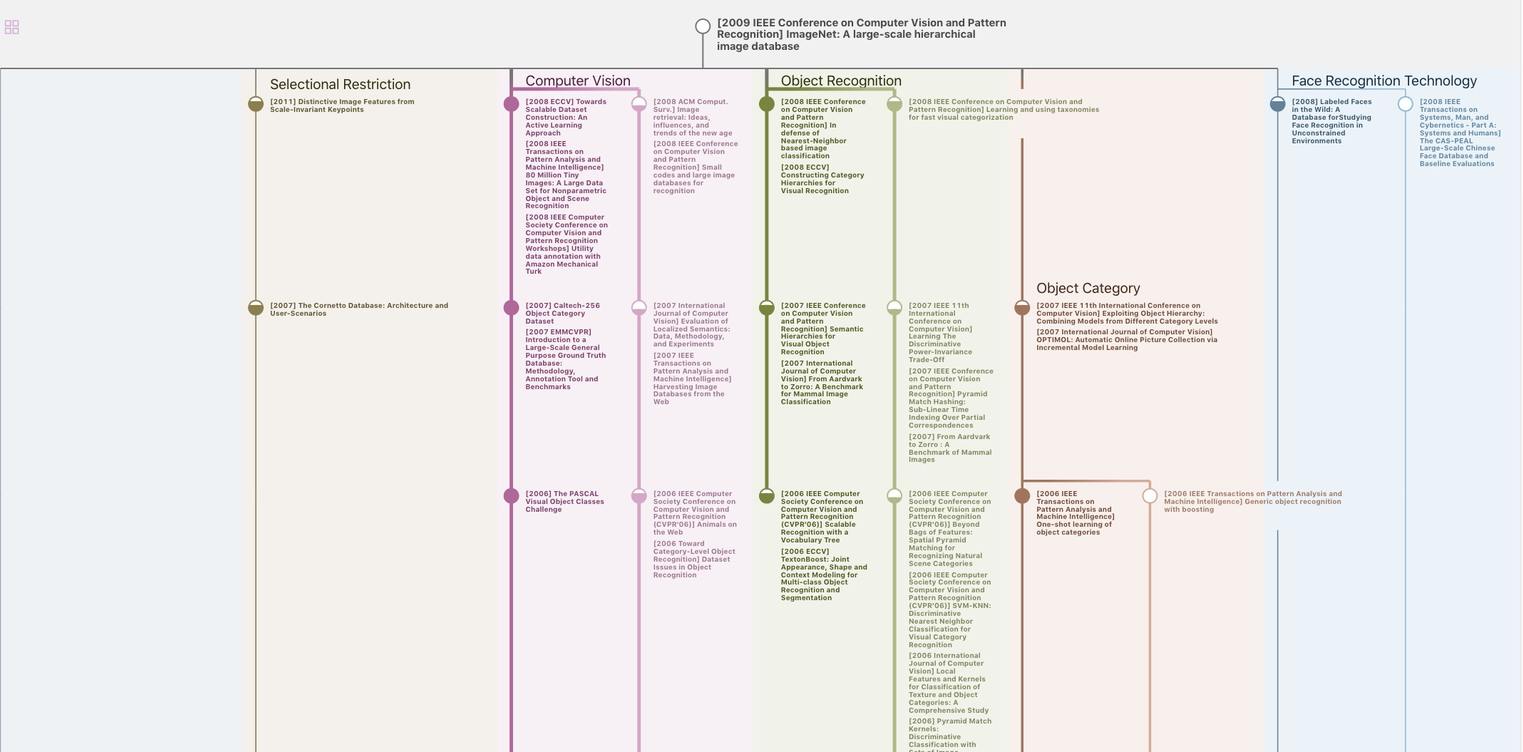
生成溯源树,研究论文发展脉络
Chat Paper
正在生成论文摘要