Pairwise classification using quantum support vector machine with Kronecker kernel
Quantum Machine Intelligence(2022)
摘要
We investigated the potential application of quantum computing using the Kronecker kernel to pairwise classification and have devised a way to apply the Harrow-Hassidim-Lloyd (HHL)-based quantum support vector machine algorithm. Pairwise classification can be used to predict relationships among data and is used for problems such as link prediction and chemical interaction prediction. However, in pairwise classification using a Kronecker kernel, it is very costly to calculate the Kronecker product of the kernel matrices when there is a large amount of data. We found that the Kronecker product of kernel matrices can be represented more efficiently in time and space in quantum computing than that in classical computing. We also found that a pairwise classifier can be effectively trained by applying the HHL-based quantum support vector machine algorithm to the Kronecker kernel matrix. In an experiment comparing a classical algorithm with a quantum support vector machine with a Kronecker kernel run on a quantum computing simulator, the misclassification rate of the latter was almost the same as that of the former for the same pairwise classification problem in some cases. This indicates that a quantum support vector machine with a Kronecker kernel algorithm can achieve accuracy equivalent to that of the classical algorithm more efficiently and scalably. This finding paves the way for applying quantum machine learning to predicting relationships in large-scale data.
更多查看译文
关键词
Quantum support vector machine,Kernel method,Pairwise classification,Quantum machine learning
AI 理解论文
溯源树
样例
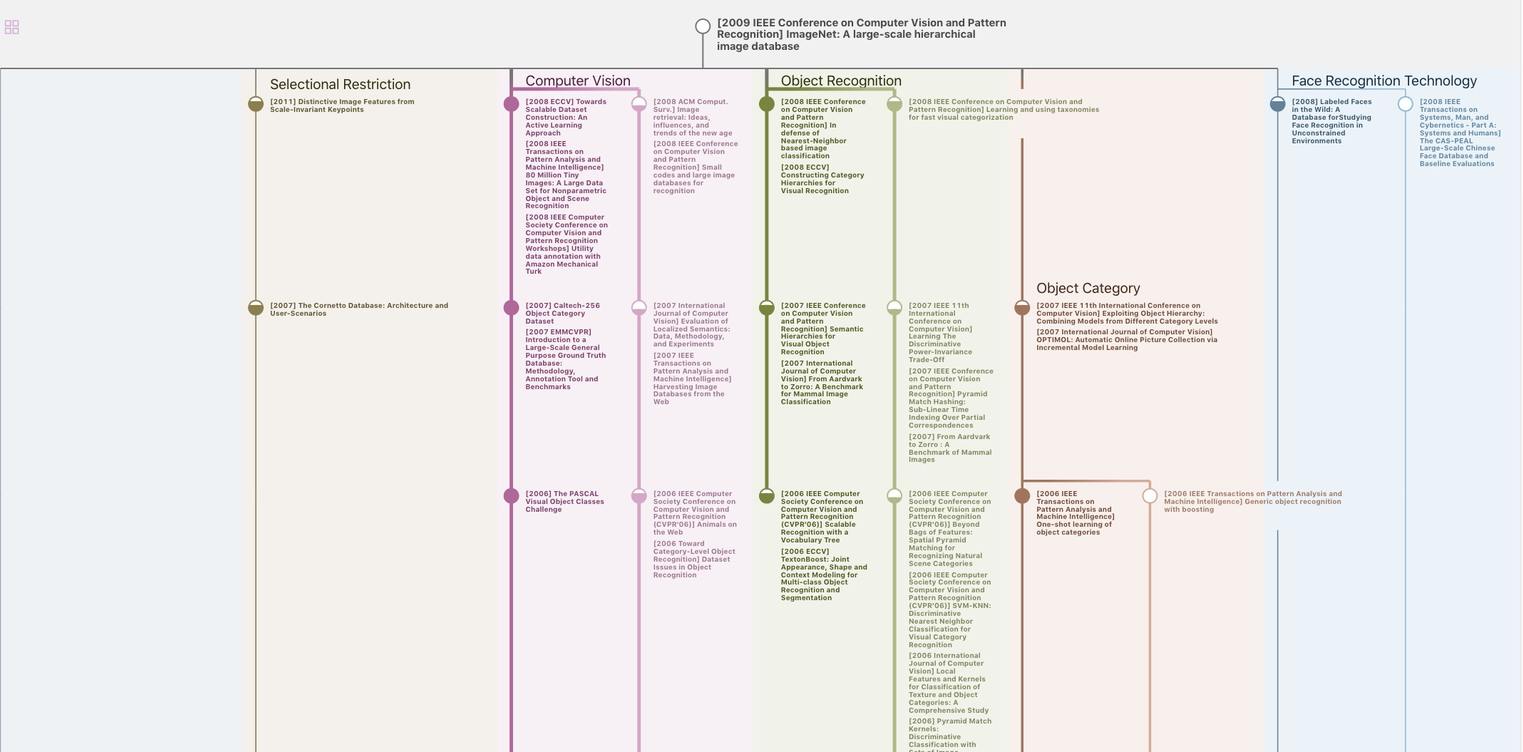
生成溯源树,研究论文发展脉络
Chat Paper
正在生成论文摘要