Ensembling Validation Indices to Estimate the Optimal Number of Clusters
Applied intelligence(2022)
摘要
In unsupervised learning tasks, one of the most significant and challenging aspects is how to estimate the optimal number of clusters (NC) for a particular set of data. Identifying NC in a given dataset is an essential criterion of cluster validity in clustering analysis. The purpose of cluster analysis is to group data points of similar characteristics, which helps determine distributions and correlations of patterns in large datasets. Recently, the availability and diversity of vast data have inspired researchers to identify an optimal NC in such data. In this paper, an ensemble approach is proposed called Ensemble Cluster Validity Index ECVI, to determine the optimal NC based on integrating and optimising several clustering validity indices, namely the Silhouette (Sil) index, the Davies–Bouldin (DB) index, the Calinski-Harabasz (CH) index, and the Gap statistic. The proposed ECVI aims to enhance the selection of the proper NC, which can be used as a measure of a dataset’s partitioning correctness to represent the actual structure of the dataset. The clustering solution (outcome) of the proposed ECVI is used as an input parameter for the k-means clustering algorithm. In other words, the proposed ECVI is concentrated to develop and validate an internal validity method in order to identify a suitable NC. The experimental comparison with the ground-truth labels for given datasets collected from the UCI repository demonstrates that the proposed ECVI outperforms and produces promising outcomes when finding the optimal ECVI in such datasets. The ECVI evaluates the clustering results obtained using a specific algorithm (e.g., k-means or affinity propagation) and identifies the optimal NC for twenty-two UCI datasets. The effectiveness of the proposed ECVI is illustrated by the theoretical analysis and then demonstrated by extensive experiments. ECVI was compared to fifteen recently published and state-of-the-art validity indices, including DB, SIL, CH, Gap, STR, EM with STR, K-means with STR, KL, Hart, Wint, IGP, Dunn, BWC, PBM, and SC indices. The experimental results show that ECVI surpasses all the compared indices in terms of the optimal NC and accuracy rate.
更多查看译文
关键词
Cluster validity index,k-means algorithm,Unsupervised learning,Number of clusters
AI 理解论文
溯源树
样例
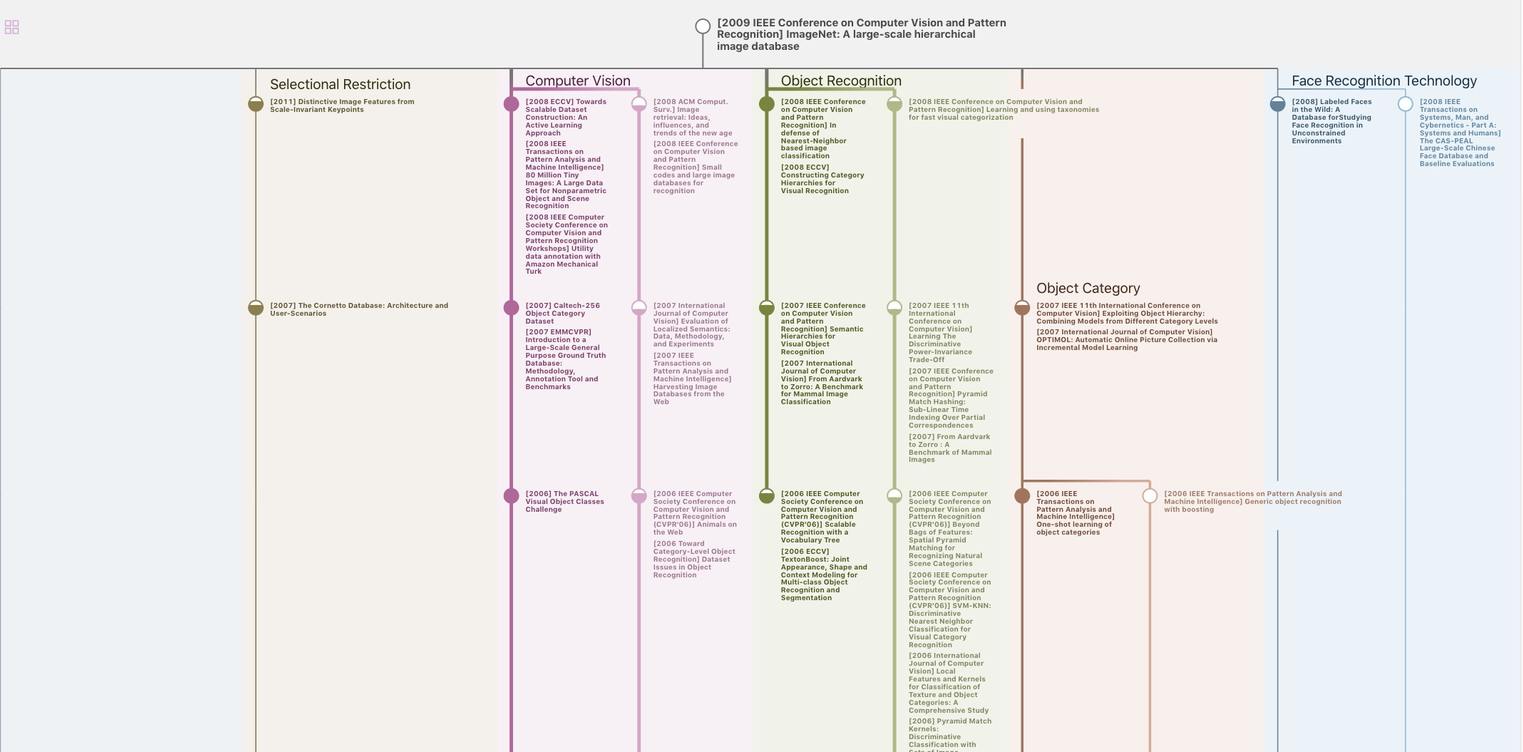
生成溯源树,研究论文发展脉络
Chat Paper
正在生成论文摘要