A neighborhood weighted-based method for the detection of outliers
Applied Intelligence(2022)
摘要
Outlierdetection is an important research direction in data mining, including fraud detection, activity monitoring, medical research, network intrusion detection, etc. Many outlier detection methods have been proposed; however, most of them are not suitable for complex patterns because they do not extract appropriate neighbor information and cannot estimate the density accurately. Additionally, their performance is not stable and depends heavily on the number of nearest neighbors ( k ) selected. To overcome the above defects, we proposed a neighborhood weighted-based outlier detection(NWOD) algorithm that can obtain correct detection result in a variety of situations. In our algorithm, the local density of an object is measured by constructing a weighted nearest neighbor graph and quantifying how difficult it is for the object and its nearest neighbors to reach each other. Furthermore, the proposed neighborhood weighted local outlier factor (NWLOF) compares the differences of the neighborhood weighted local density between a given object and the objects in its neighborhood, and then the degree of being an outlier of an object can be judged. The larger the NWLOF of an object is, the more likely it is to be an outlier. In addition, due to our proposed algorithm being based on the concept of a natural stable structure, its performance does not rely on the value of k . Experiments conducted on both synthetic and real-world datasets show the superiority of our algorithm.
更多查看译文
关键词
Data mining,Outlier detection,Weighted local density,Natural stable structure
AI 理解论文
溯源树
样例
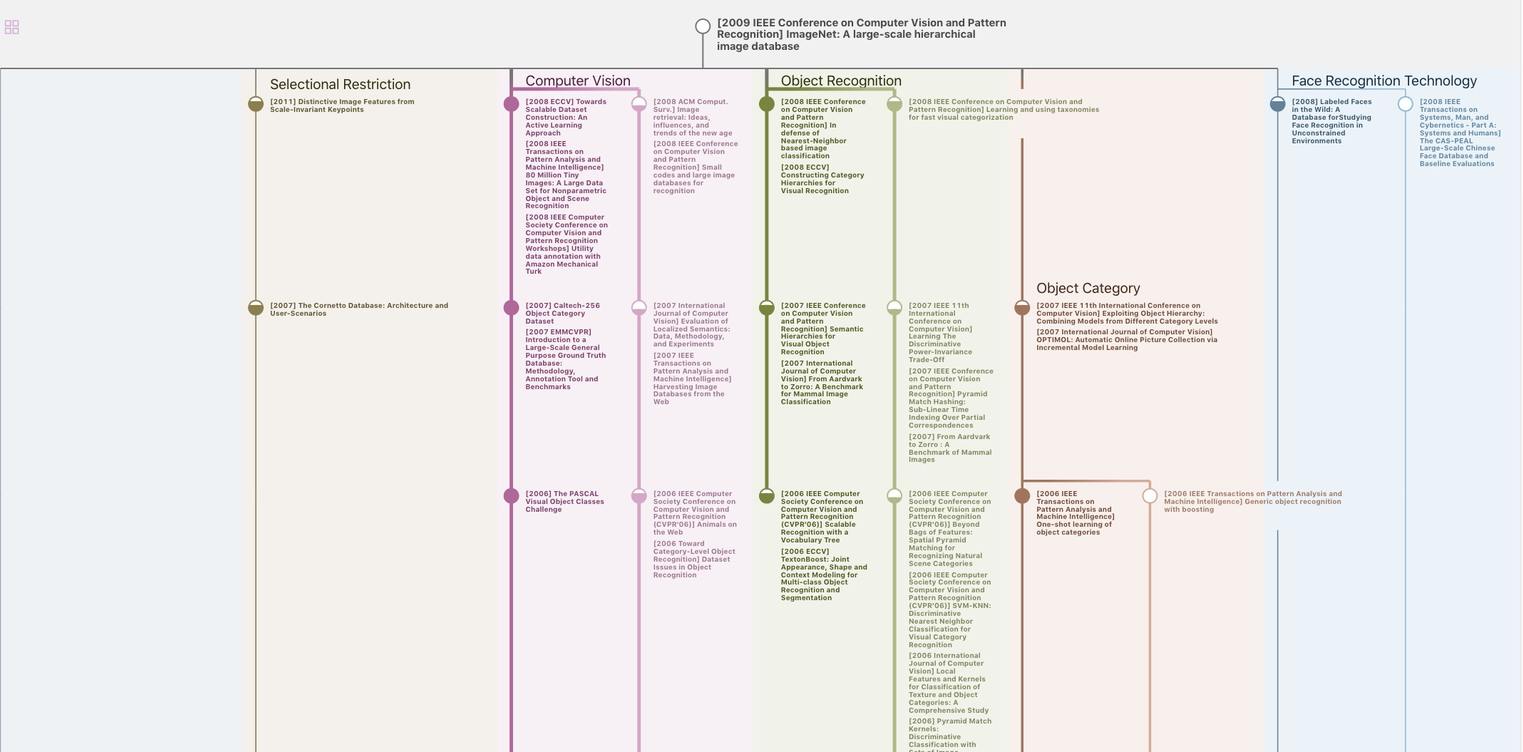
生成溯源树,研究论文发展脉络
Chat Paper
正在生成论文摘要