Introducing the Software CASE (Cluster and Analyze Sound Events) by Comparing Different Clustering Methods and Audio Transformation Techniques Using Animal Vocalizations
ANIMALS(2022)
摘要
Simple Summary Unsupervised clustering algorithms are widely used in ecology and conservation to classify animal vocalizations, but also offer various advantages in basic research, contributing to the understanding of acoustic communication. Nevertheless, there are still some challenges to overcome. For instance, the quality of the clustering result depends on the audio transformation technique previously used to adjust the audio data. Moreover, it is difficult to verify the reliability of the clustering result. To analyze bioacoustic data using a clustering algorithm, it is, therefore, essential to select a reasonable algorithm from the many existing algorithms and prepare the recorded vocalizations so that the resulting values characterize a vocalization as accurately as possible. Frequency-modulated vocalizations, whose frequencies change over time, pose a particular problem. In this paper, we present the software CASE, which includes various clustering methods and provides an overview of their strengths and weaknesses concerning the classification of bioacoustic data. This software uses a multidimensional feature-extraction method to achieve better clustering results, especially for frequency-modulated vocalizations. Unsupervised clustering algorithms are widely used in ecology and conservation to classify animal sounds, but also offer several advantages in basic bioacoustics research. Consequently, it is important to overcome the existing challenges. A common practice is extracting the acoustic features of vocalizations one-dimensionally, only extracting an average value for a given feature for the entire vocalization. With frequency-modulated vocalizations, whose acoustic features can change over time, this can lead to insufficient characterization. Whether the necessary parameters have been set correctly and the obtained clustering result reliably classifies the vocalizations subsequently often remains unclear. The presented software, CASE, is intended to overcome these challenges. Established and new unsupervised clustering methods (community detection, affinity propagation, HDBSCAN, and fuzzy clustering) are tested in combination with various classifiers (k-nearest neighbor, dynamic time-warping, and cross-correlation) using differently transformed animal vocalizations. These methods are compared with predefined clusters to determine their strengths and weaknesses. In addition, a multidimensional data transformation procedure is presented that better represents the course of multiple acoustic features. The results suggest that, especially with frequency-modulated vocalizations, clustering is more applicable with multidimensional feature extraction compared with one-dimensional feature extraction. The characterization and clustering of vocalizations in multidimensional space offer great potential for future bioacoustic studies. The software CASE includes the developed method of multidimensional feature extraction, as well as all used clustering methods. It allows quickly applying several clustering algorithms to one data set to compare their results and to verify their reliability based on their consistency. Moreover, the software CASE determines the optimal values of most of the necessary parameters automatically. To take advantage of these benefits, the software CASE is provided for free download.
更多查看译文
关键词
bioacoustics, clustering methods, feature extraction, frequency-modulated vocalizations, multidimensional, vocalization classification, vocal repertoire
AI 理解论文
溯源树
样例
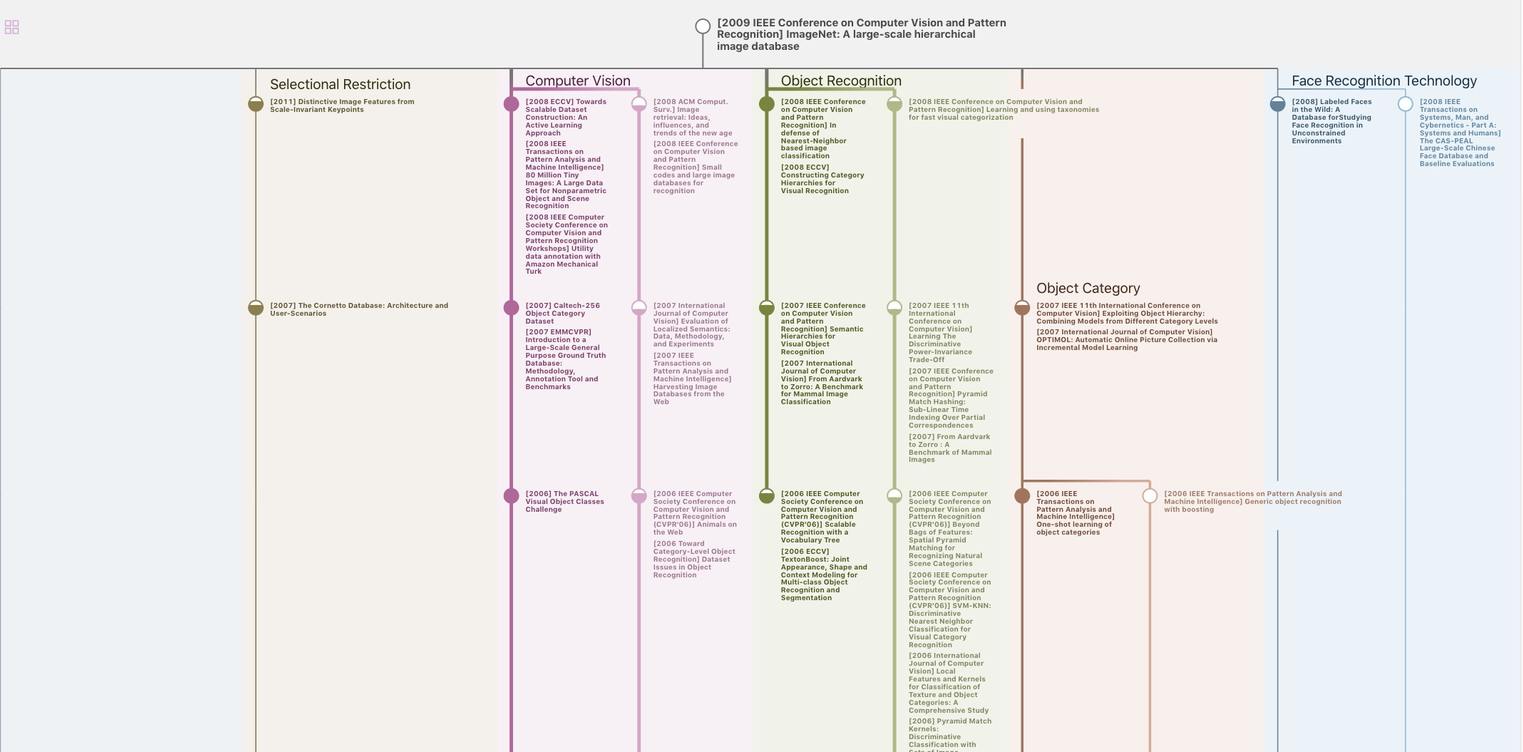
生成溯源树,研究论文发展脉络
Chat Paper
正在生成论文摘要