COMPUTER AIDED DIAGNOSIS FOR DYSPLASIA CHARACTERISATION IN BARRETT'S ESOPHAGUS WITH MAGNIFICATION ENDOSCOPY ON I-SCAN IMAGING
GUT(2022)
摘要
Introduction
We aimed to develop a computer aided detection system that can support the diagnosis of BE dysplasia on magnification endoscopy.Methods
Videos were collected in high-definition magnification white light and virtual chromoendoscopy with i-scan (Pentax Hoya, Japan) imaging in patients with dysplastic/non-dysplastic BE (NDBE) from 4 centres. We trained a neural network with a Resnet101 architecture to classify frames. The network was tested – on high quality still images, all available video frames and on a selected sequence within each video.Results
57 different patients each with videos of magnification areas of BE (34 dysplasia, 23 NDBE) were included. Performance was evaluated using a leave-one-out cross-validation methodology. 60,174 (39,347 dysplasia, 29,827 NDBE) magnification video frames were used to train the network. The testing set included 49,726 iscan-3/optical enhancement magnification frames. On 350 high quality images the network achieved a sensitivity of 94%, specificity of 86% and Area under the ROC (AUROC) of 96%. On all 49,726 frames the network achieved a sensitivity of 92%, specificity of 82% and AUROC of 95%. On a selected sequence of frames per case (Total of 11,471 frames) we used an exponentially weighted moving average of consecutive frames to diagnose dysplasia. The network achieved a sensitivity of 90%, specificity of 82% and AUROC of 94% (figure 1). The mean assessment speed per frame was 0.0135 seconds (SD, ± 0.006).Conclusion
Our network can characterise BE dysplasia with high accuracy and speed on high-quality magnification images and sequence of video frames moving it towards real time automated diagnosis.查看译文
关键词
magnification endoscopy,esophagus,barretts,dysplasia characterisation,i-scan
AI 理解论文
溯源树
样例
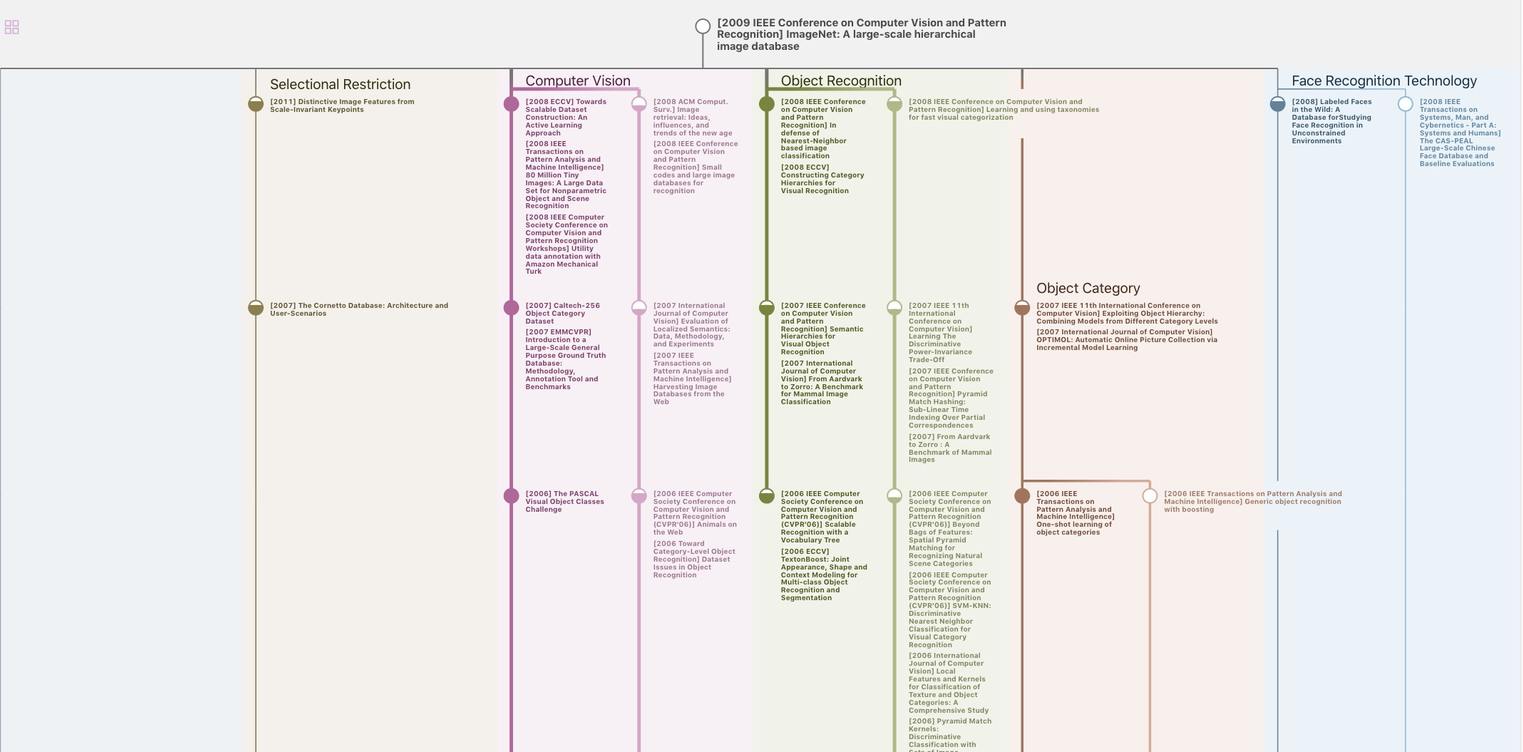
生成溯源树,研究论文发展脉络
Chat Paper
正在生成论文摘要