A Dimension Reduction in Neural Network Using Copula Matrix
International journal of general systems(2022)
摘要
In prediction analysis, there may exist some nonlinear relations between the exploratory variables, which are not captured by traditional correlation-based linear models such as multiple regression, principal component regression, and so on. In this work, we employ a copula matrix to extract principal components of a set of variables which are pair-wisely associated with a copula. By estimating the pairwise copula and its corresponding parameter(s), we suggest an optimization method to extract principal components from a matrix which contains some pairwise measures of association. We use these components as inputs of an artificial neural network to make a more accurate prediction. We test our proposed method using a simulation study and use it to carry out a more accurate prediction in an AIDS as well as a COVID-19 dataset. To increase the reliability of results, we employ a cross-validation technique.
更多查看译文
关键词
Principal component,copula,neural network,correlation,association measure
AI 理解论文
溯源树
样例
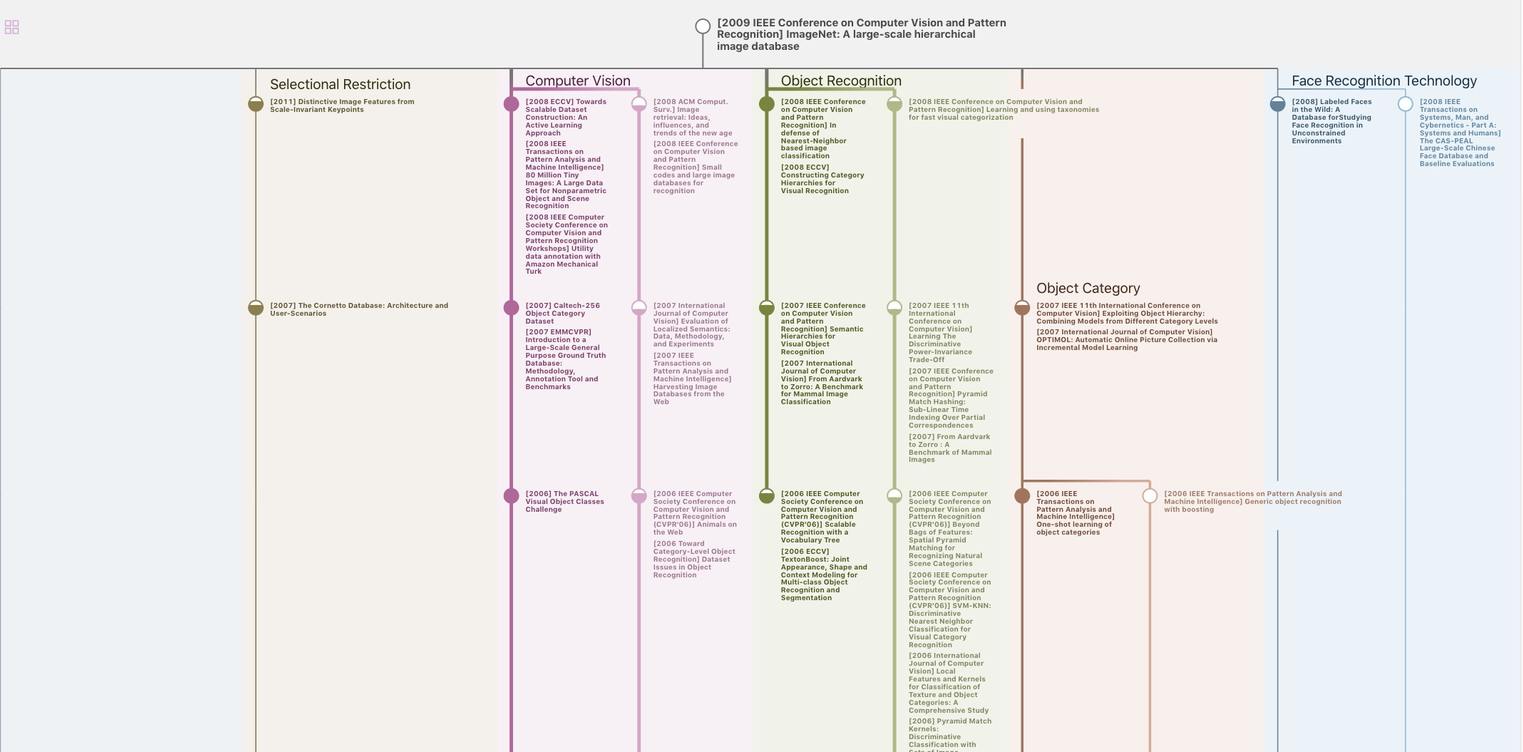
生成溯源树,研究论文发展脉络
Chat Paper
正在生成论文摘要