Multi-Agent Model-Based Reinforcement Learning for Trajectory Design and Power Control in UAV-Enabled Networks
2022 3rd Information Communication Technologies Conference (ICTC)(2022)
摘要
Unmanned aerial vehicles (UAVs) serving as aerial base stations is a promising technology for wireless communications. This paper formulates a joint optimization problem of UAV trajectory design and power control to minimize the power consumption when satisfying users’ QoS requirements in a downlink transmission. Firstly, a multi-agent deep deterministic policy gradient (MADDPG) scheme with centralized training and decentralized execution is proposed to improve the overall performance of the UAVs in cooperation. Secondly, model value expansion (MVE) is incorporated into the model-free MADDPG scheme. By imaging future transitions, the proposed multiagent model value expansion deep deterministic policy gradient (MA-MVE-DDPG) algorithm generates more experiences, and thus accelerates training. Simulation results have demonstrated that our proposed MA-MVE-DDPG algorithm achieves better performance and converges faster than benchmark schemes.
更多查看译文
关键词
Unmanned aerial vehicle (UAV),trajectory design,power control,reinforcement learning,model value expansion.
AI 理解论文
溯源树
样例
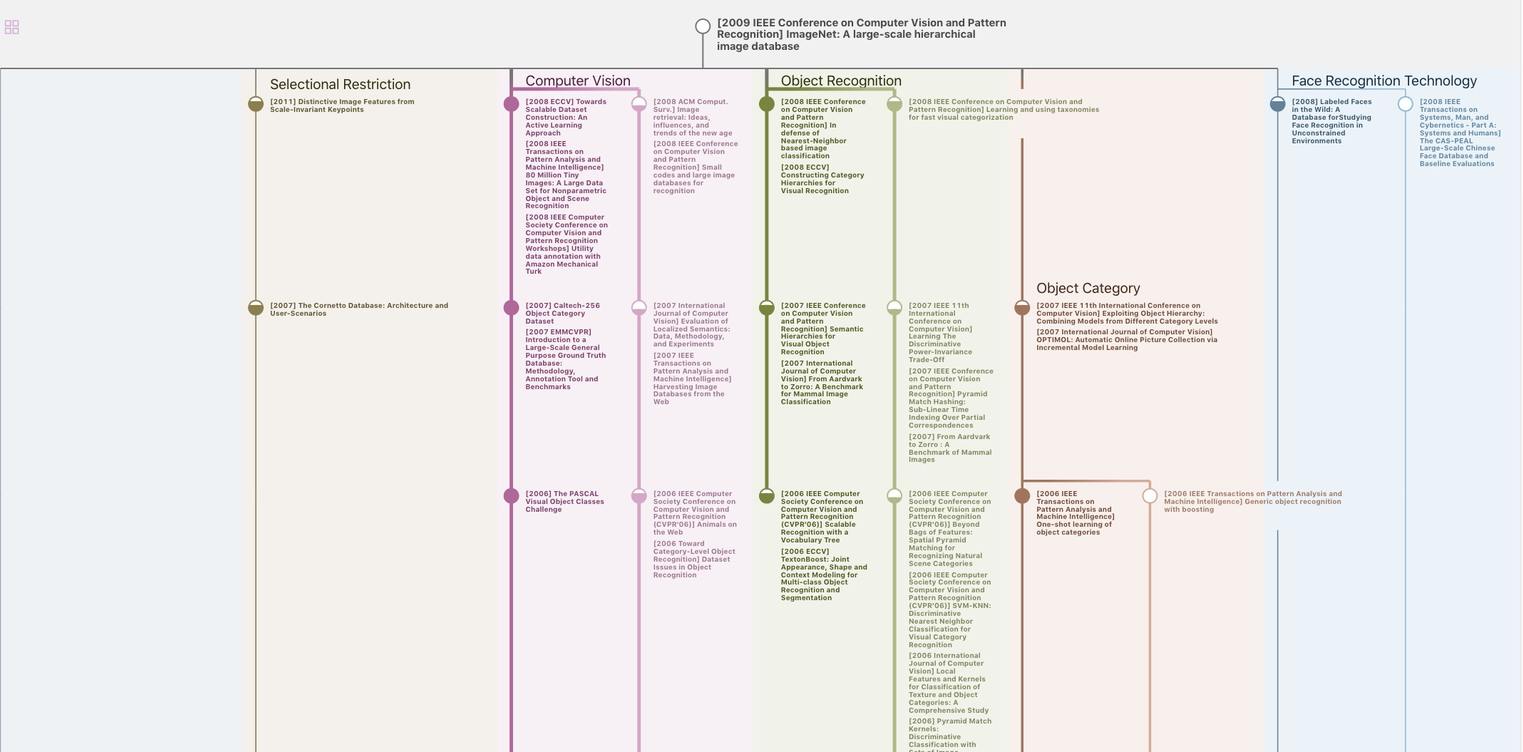
生成溯源树,研究论文发展脉络
Chat Paper
正在生成论文摘要