Normalizing flows for likelihood-free inference with fusion simulations
PLASMA PHYSICS AND CONTROLLED FUSION(2022)
摘要
Fluid-based scrape-off layer transport codes, such as UEDGE, are heavily utilized in tokamak analysis and design, but typically require user-specified anomalous transport coefficients to match experiments. Determining the uniqueness of these parameters and the uncertainties in them to match experiments can provide valuable insights to fusion scientists. We leverage recent work in the area of likelihood-free inference ('simulation-based inference') to train a neural network, which enables accurate statistical inference of the anomalous transport coefficients given experimental plasma profile input. UEDGE is treated as a black-box simulator and runs multiple times with anomalous transport coefficients sampled from priors, and the neural network is trained on these simulations to emulate the posterior. The neural network is trained as a normalizing flow model for density estimation, allowing it to accurately represent complicated, high-dimensional distribution functions. With a fixed simulation budget, we compare a single-round procedure to a multi-round approach that guides the training simulations toward a specific target observation. We discuss the future possibilities for use of amortized models, which train on a wide range of simulations and enable fast statistical inference for results during experiments.
更多查看译文
关键词
UEDGE, normalizing flows, likelihood-free inference, deep learning
AI 理解论文
溯源树
样例
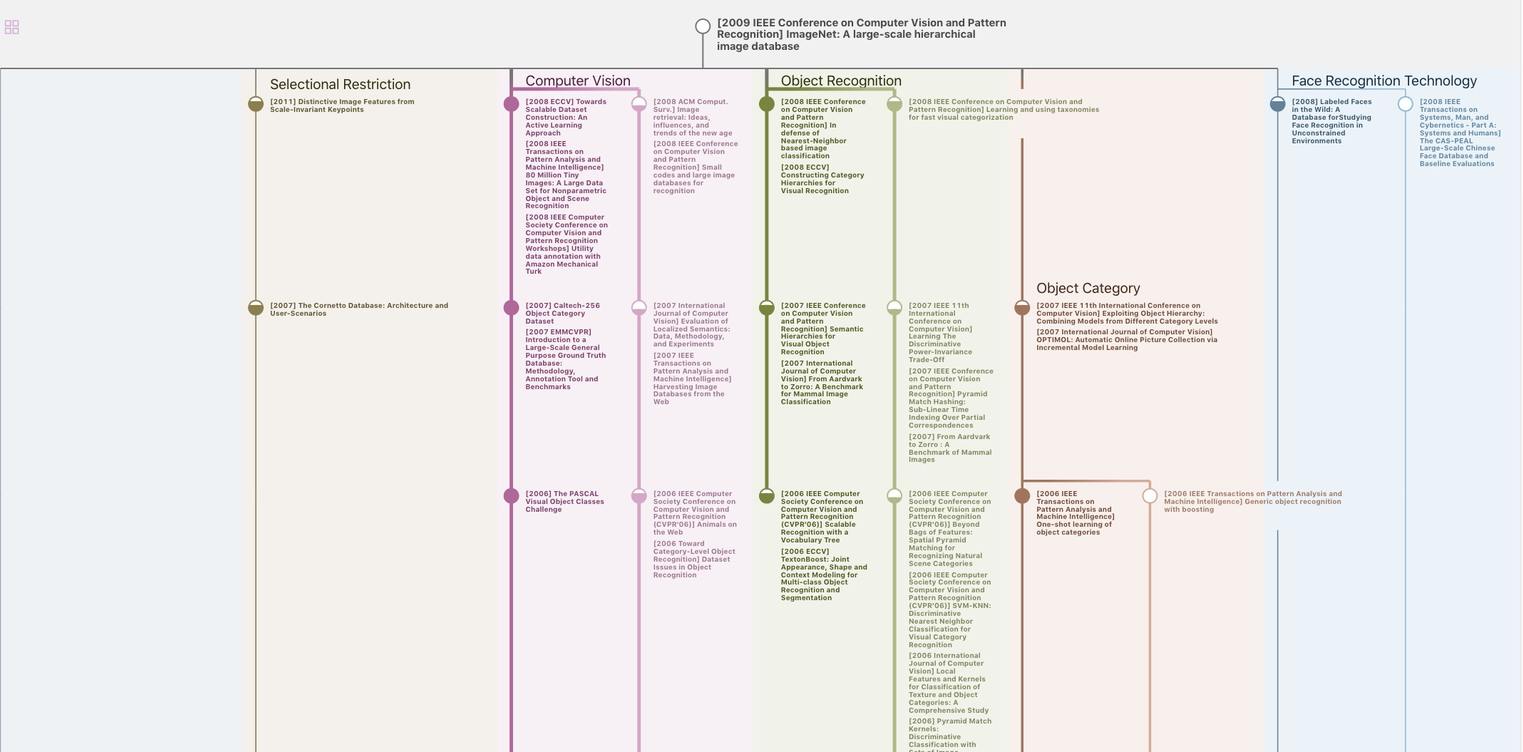
生成溯源树,研究论文发展脉络
Chat Paper
正在生成论文摘要