UNICON: Uncertainty-Conditioned Policy for Robust Behavior in Unfamiliar Scenarios
IEEE Robotics and Automation Letters(2022)
摘要
Deep reinforcement learning has been used to solve complex tasks in various fields, particularly in robotics control. However, agents trained using deep reinforcement learning have a problem of taking overconfident actions, even when the input state is far from the learned state distribution. This restricts deep reinforcement learning from being applied to real-world environments as overconfident actions in unlearned situations can result in catastrophic events; such as the collision of an autonomous vehicle. To address this, the agents should know “what they do not know” and choose an action by considering not only the state but also its uncertainty. In this study, we propose a novel uncertainty-conditioned policy (UNICON) inspired by the human behavior of changing policies according to uncertainty, e.g., slowing a car on a narrow road that has never been visited before. Our experimental results demonstrate that the proposed method is robust to unfamiliar scenarios that are not seen during training.
更多查看译文
关键词
Uncertainty-conditioned policy,robust behavior
AI 理解论文
溯源树
样例
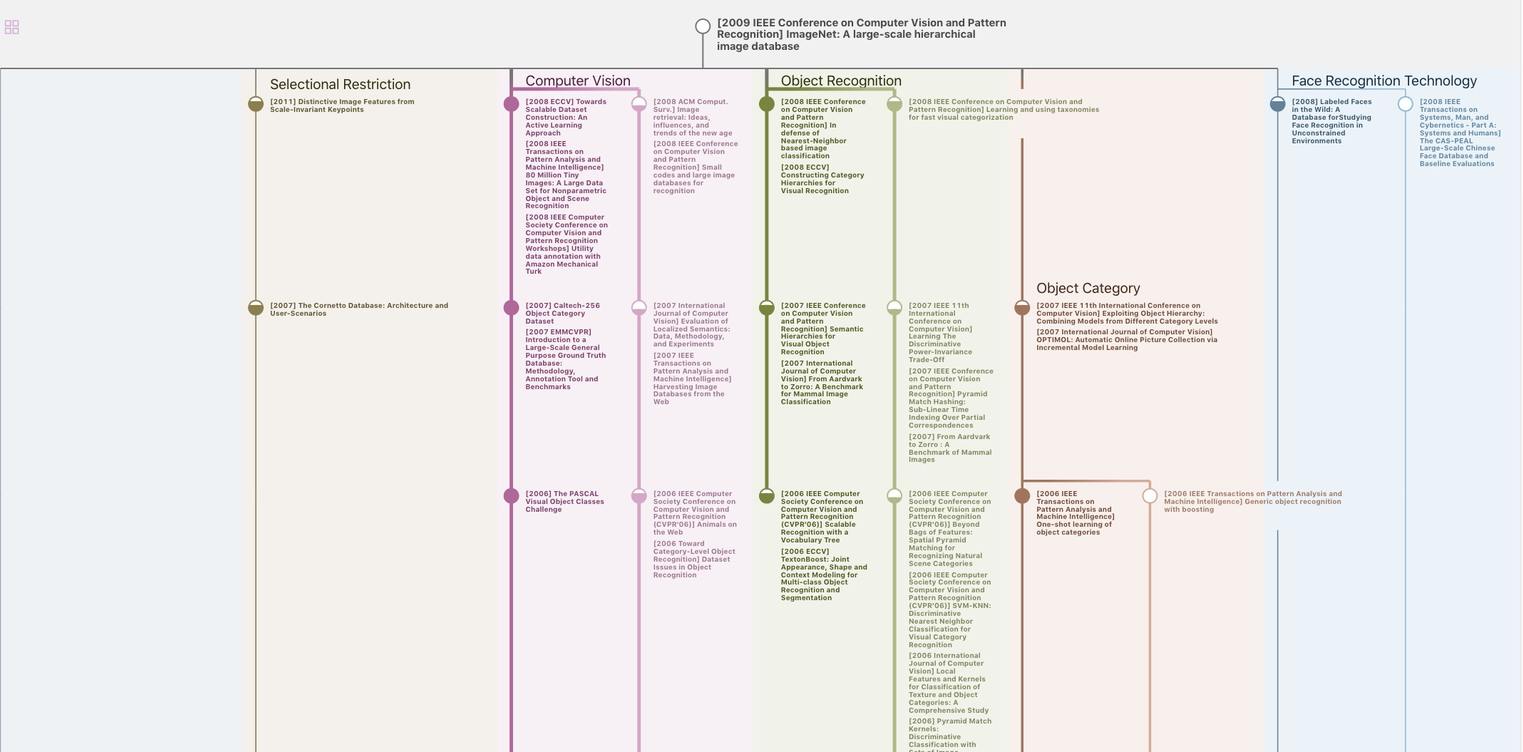
生成溯源树,研究论文发展脉络
Chat Paper
正在生成论文摘要