A Data-Driven Method for Metric Extraction to Detect Faults in Robot Swarms
IEEE ROBOTICS AND AUTOMATION LETTERS(2022)
Abstract
Robot swarms are increasingly deployed in real-world applications. Making swarms safe will be critical to improve adoption and trust. Fault detection is a useful component in systems which require a level of safety: a key element of which are metrics that allow us to differentiate between faulty and normal (non-faulty) robots - metrics which are measurable on-board the individual robots for self-detection of faults. In this paper, we develop a method for identifying and evaluating such metrics and discuss how these metrics may be used in building a model for fault detection. We demonstrate this method for real-time error detection in a realistic use-case: intralogistics using swarms. We show that we are able to identify metrics of large effect size for various faults, demonstrating the potency of metrics selected in this way with a simple fault detection model.
MoreTranslated text
Key words
Swarm robotics, probability and statistical methods, fault detection, metric extraction
AI Read Science
Must-Reading Tree
Example
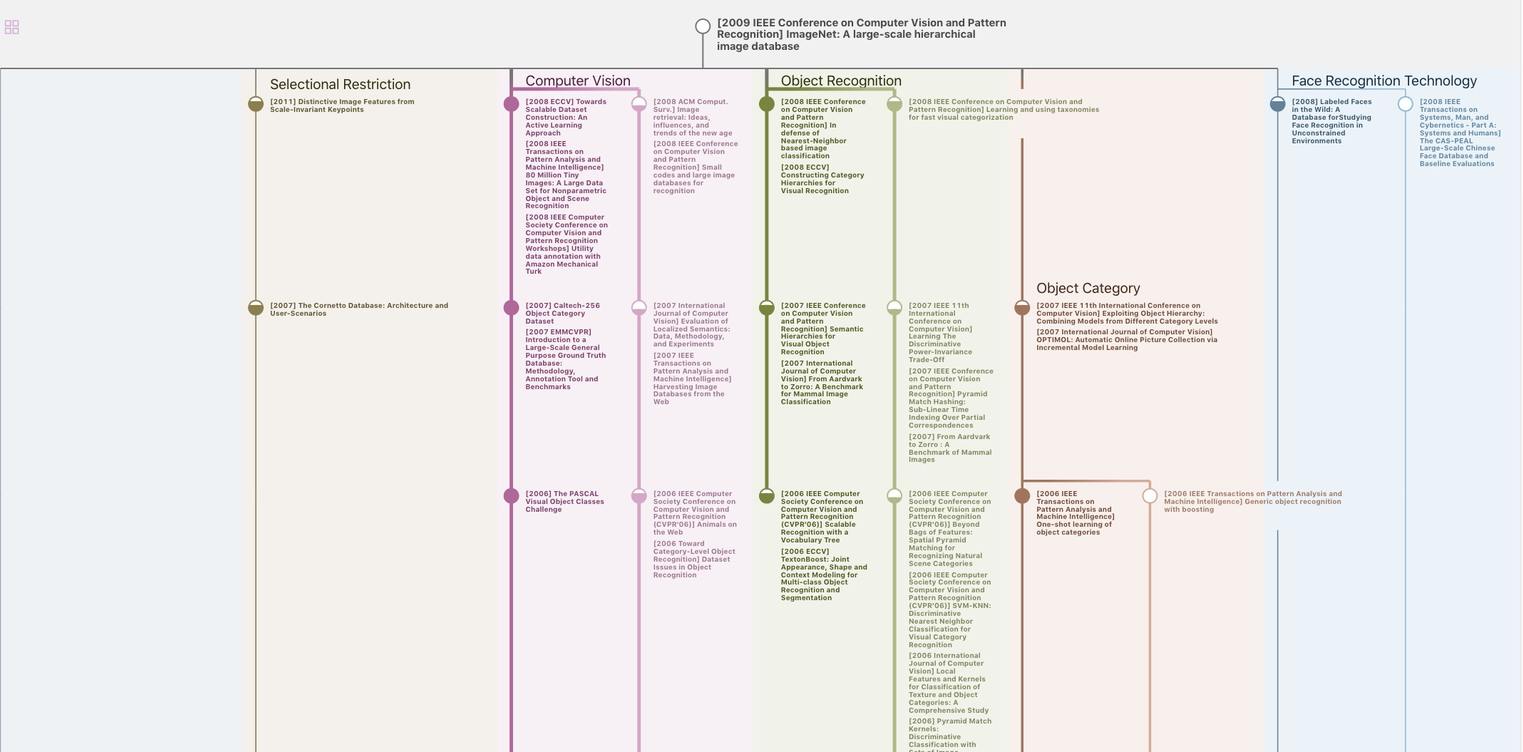
Generate MRT to find the research sequence of this paper
Chat Paper
Summary is being generated by the instructions you defined