PDDNet: lightweight congested crowd counting via pyramid depth-wise dilated convolution
Applied Intelligence(2022)
摘要
The accuracy of crowd counting is susceptible to scale variations of crowd head in the congested scene. Some counting networks, such as crowd density pre-classification networks or multi-column counting networks, are proposed to model the different scales of crowd head. However, most of them own a complex network structure with many network parameters, making deploying a crowd counting network in practical application scenarios challenging. To this end, we propose a lightweight crowd counting network termed PDDNet. The front-end of the PDDNet chooses the first 13 layers of GhostNet to extract the crowd feature, and the back-end of the PDDNet is implemented with the proposed lightweight pyramidal convolution modules (LPC) to extract the multi-scale features. Finally, the extracted multi-scale features are fed to transposed convolution layers to regress the final crowd density map. We conduct extensive experiments on the commonly-used crowd counting datasets, i.e., ShanghaiTech, UCF_QNRF, and NWPU_Crowd. The experiment results show the superiority of our model compared with state-of-the-art methods.
更多查看译文
关键词
Crowd counting, Lightweight network, Multi-scale feature learning, Pyramidal convolution
AI 理解论文
溯源树
样例
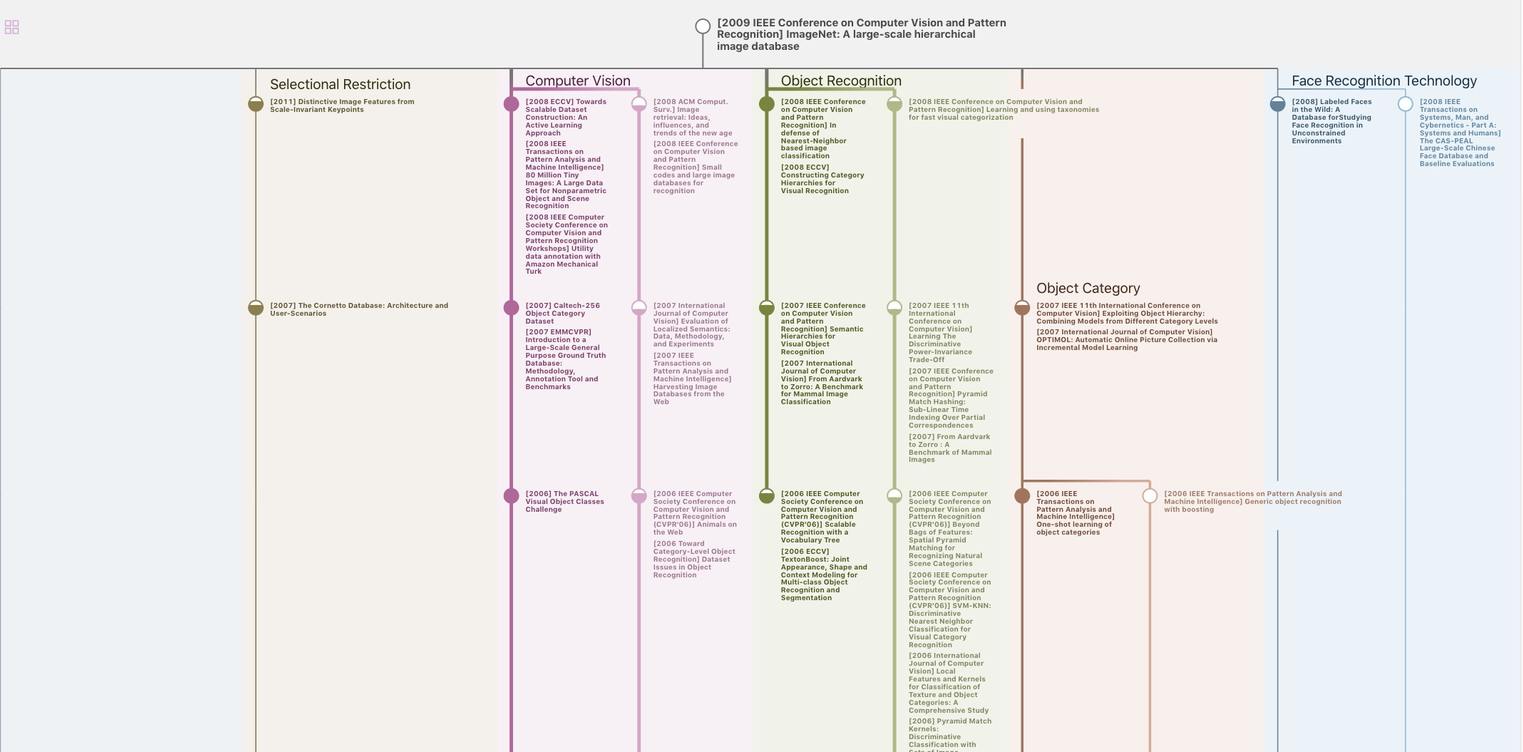
生成溯源树,研究论文发展脉络
Chat Paper
正在生成论文摘要