Weight grouping operators selection strategy for a multiobjective evolutionary algorithm based on decomposition
Applied Intelligence(2022)
摘要
Multiobjective evolutionary algorithm based on decomposition (MOEA/D) works by using a set of uniformly distributed weight vectors to decompose a multiobjective optimization problem (MOP) into multiple single objective optimization subproblems for simultaneous optimization. Selecting parents from the neighborhood when performing reproduction is highly probable, thus different operators and neighborhoods may produce different offspring. However, one omnipotent operator usually cannot handle all different complex MOPs very well, for this reason, we propose a weight grouping operators selection (WGOS) strategy. Firstly, we divide the weight vectors into several groups and assign different reproduction operators to each group. The subproblems in each group are optimized by using the reproduction operators assigned. Then, the size of each group is dynamically adjusted according to the quality of the generated offspring, and the group of the reproduction operator that generates the better offspring expands, and the group of the reproduction operator that generates the worse offspring shrinks correspondingly until all the groups are merged into one and all adopt the same reproduction operator. This process will eventually pick out one operator for subsequent evolution. Finally, we will verify the performance of the selected operator to decide whether to continue using it or switch to another operator. A large number of comparative experiments have proved this strategy has better performance.
更多查看译文
关键词
Multiobjective evolutionary algorithms, Decomposition, Weight vector group, Operator selection
AI 理解论文
溯源树
样例
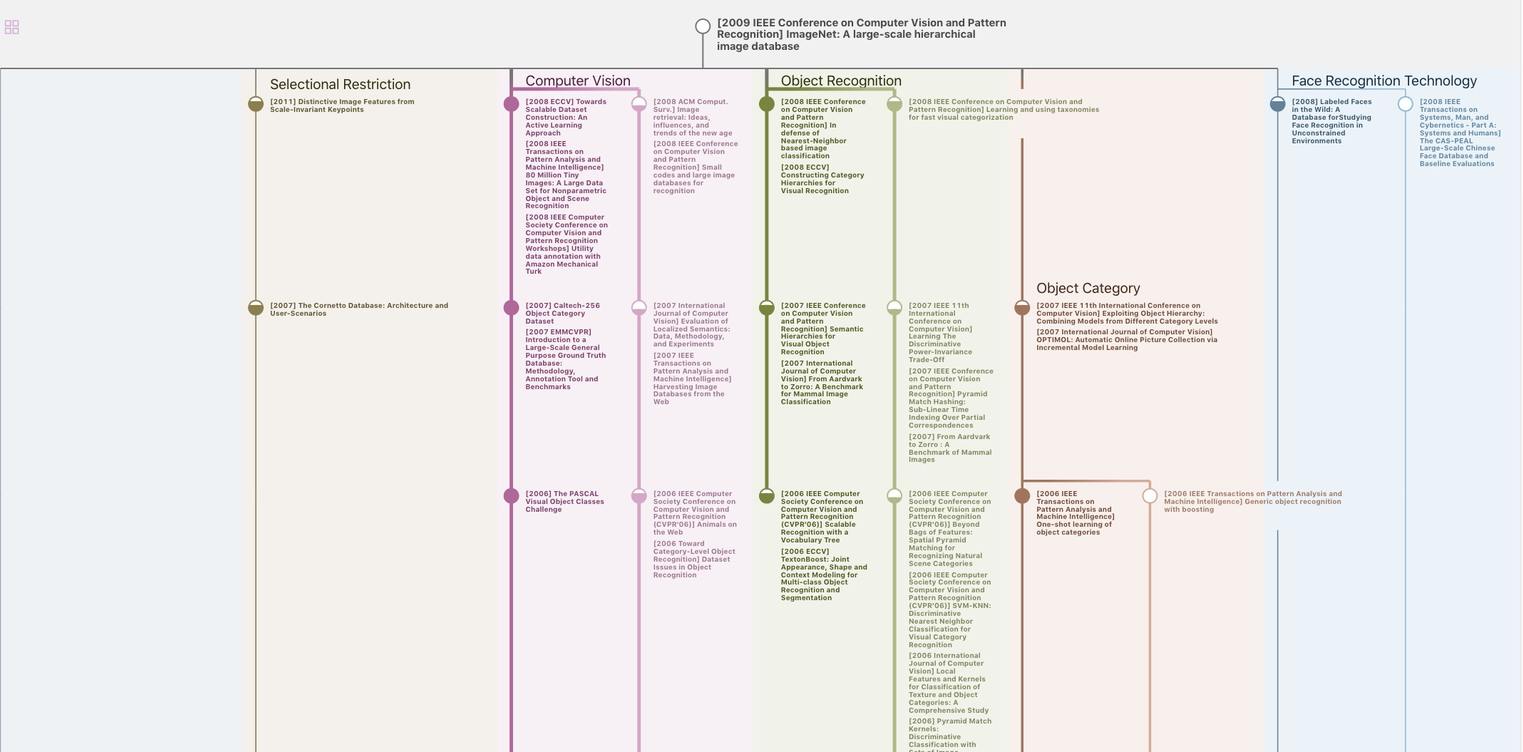
生成溯源树,研究论文发展脉络
Chat Paper
正在生成论文摘要