Sequential classification of customer behavior based on sequence-to-sequence learning with gated-attention neural networks
ADVANCES IN DATA ANALYSIS AND CLASSIFICATION(2022)
摘要
During the last decade, an increasing number of supermarkets have begun to use RFID technology to track consumers' in-store movements to collect data on their shopping behavioral. Marketers hope that such new types of RFID data will improve the accuracy of the existing customer segmentation, and provide effective marketing positioning from the customer’s perspective. Therefore, this paper presents an integrated work on combining RFID data with traditional point of sales (POS) data, and proposes a sequential classification-based model to classify and identify consumers’ purchasing behavior. We chose an island area of the supermarket to perform the tracking experiment and collected customer behavioral data for two months. RFID data are used to extract behavior explanatory variables, such as residence time and wandering direction. For these customers, we extracted their purchasing historical data for the past three months from the POS system to define customer background and segmentation. Finally, this paper proposes a novel classification model based on sequence-to-sequence (Seq2seq) learning architecture. The encoder–decoder of Seq2seq uses an attention mechanism to pursue sequential inputs, with gating units in the encoder and decoder adjusting the output weights based on the input variables. The experimental results showed that the proposed model has a higher accuracy and area under curve value for customer classification and recognition compared with other benchmark models. Furthermore, the validity of behavioral description variables among heterogeneous customers was verified by adjusting the attention mechanism.
更多查看译文
关键词
RFID data, Customer behavior, Seq2seq, Attention mechanism, Classification
AI 理解论文
溯源树
样例
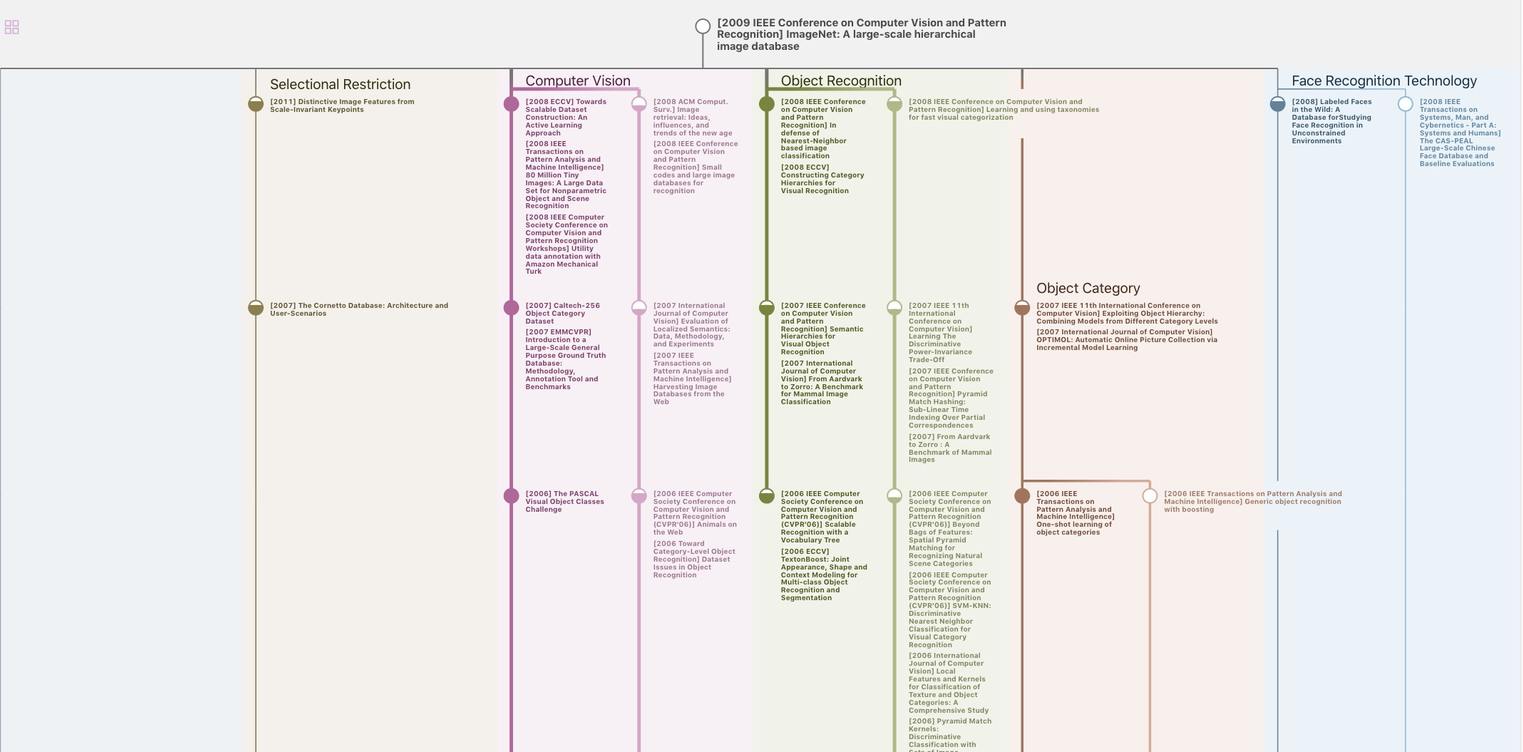
生成溯源树,研究论文发展脉络
Chat Paper
正在生成论文摘要