MACHINE LEARNING IDENTIFIES A BIOMARKER DERIVED FROM HUMAN COLONOIDS PREDICTING RESPONSE TO USTEKINUMAB IN IBD
GUT(2022)
摘要
Background
Cytokines mediating canonical immune responses in the epithelium regulate a large proportion of the transcriptional changes (~8,000 genes) captured by the transcriptomic profiling of the biopsied intestinal mucosa in active inflammatory bowel disease (IBD) and associate with response to therapy. In this study, we use a semi-supervised approach to discover and validate a gene signature based on cytokine regulated transcriptional programmes predicting response to ustekinumab in IBD.Methods
Cytokine regulated transcriptional programmes were generated by treating colonic organoids, originating from healthy individuals (n=4), with TNFα (10ng/mL), IFNγ(20ng/mL), IL13(10ng/mL), IL17A (50ng/mL) or IL22(10ng/mL) for 24hrs and then performing whole transcriptome sequencing on extracted RNA. An exploratory receiver operator characteristic curve (ROC) analysis was first performed to test the prognostic value of the enrichment of cytokine regulated gene sets of varying sizes in mucosal biopsies collected in the context of UNITI2 (phase III clinical trial testing efficacy of ustekinumab in Crohn’s disease). Gene set enrichment was performed with the gene set variation analysis (GSVA package implemented in R) and mucosal healing (defined as endoscopic healing and clinical remission) was used as the outcome of interest. Following on from the ROC analysis a filtered number of transcripts was used to identify the best combination of genes predicting outcome in UNITI2 (training set, n=197) using a generalised linear model with elastic net penalty (glmnet package implemented in R). The predictive value of the gene set defined in the training set was then validated in separate cohorts (validation set, UNIFI-n=356, UNITI1-n=101).Results
While there was a numerical difference in the area under the curve (AUC, IL22>IFNγ>TNFα>IL13>IL17A) most signatures appeared to predict with similar accuracy mucosal healing in UNITI2. In addition, the exploratory ROC analysis suggested that gene sets consisting of 50 genes for each cytokine had better predictive value than larger gene sets (100, 150, 250 upregulated genes) based on AUC. Hence, we decided to use the top 50 upregulated genes from each cytokine as the pool of potential candidate genes for the discovery of a predictive set (total n=293). The machine learning algorithm identified 14 genes to be predictive of mucosal healing (AUC: 90%, 95%CI[84,96] in colon and 87%, 95%CI[79,94] in ileum). Validation showed a similar performance in independent cohorts in Crohn’s disease (UNITI1, AUC: 92%, 95%CI[83,100] in colon and AUC:94%, 95%CI[87,100] in ileum) while the performance was lower in ulcerative colitis (UC) (UNIFI, AUC:72%, 95%CI[68,77]).Conclusion
A 14 gene signature derived from cytokine regulated programmes in human colonoids predicts with high accuracy mucosal healing in Crohn’s disease in response to ustekinumab paving the way for precision medicine therapeutic approaches in IBD.查看译文
关键词
human colonoids,ustekinumab,biomarker
AI 理解论文
溯源树
样例
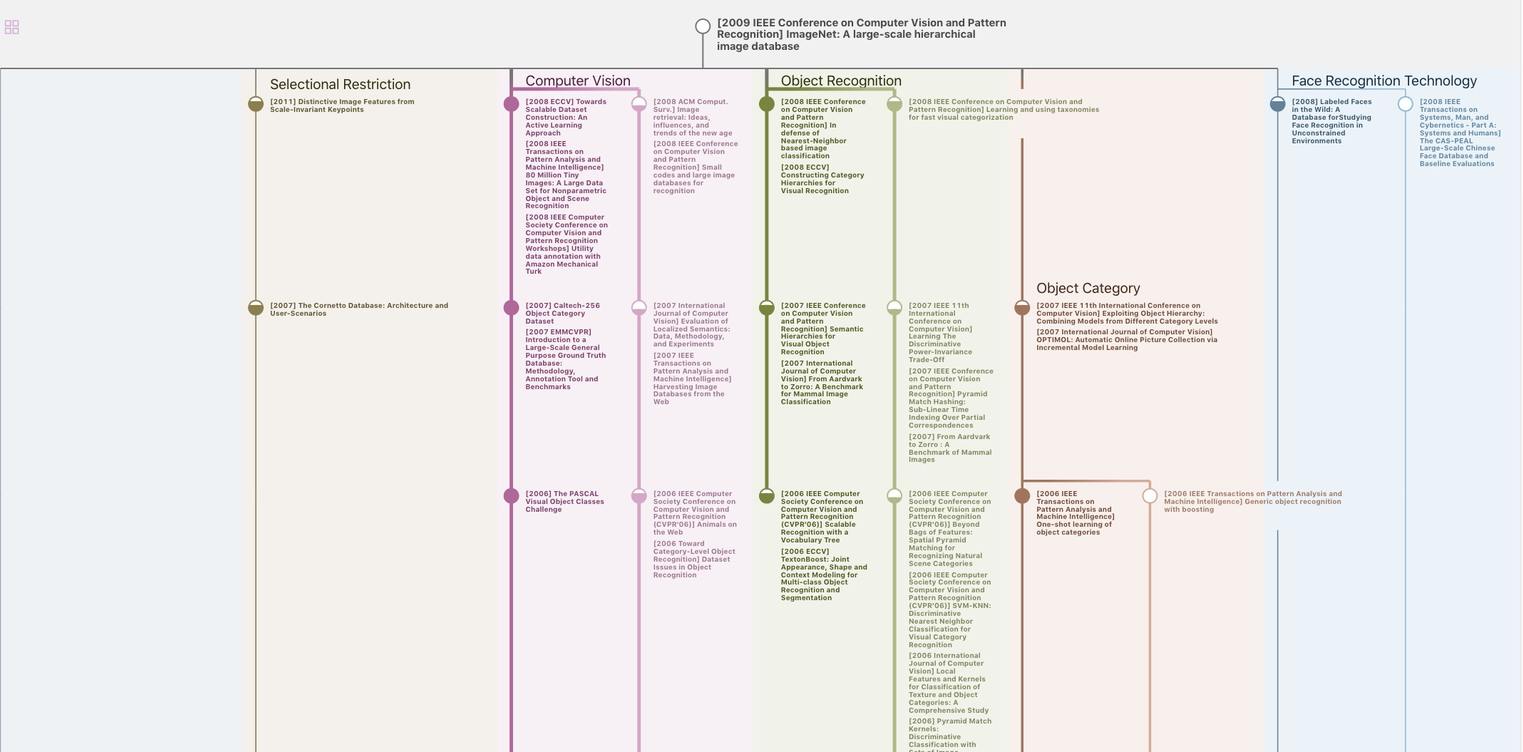
生成溯源树,研究论文发展脉络
Chat Paper
正在生成论文摘要