A Novel K-Means Clustering Method for Locating Urban Hotspots Based on Hybrid Heuristic Initialization
APPLIED SCIENCES-BASEL(2022)
摘要
With rapid economic and demographic growth, traffic conditions in medium and large cities are becoming extremely congested. Numerous metropolitan management organizations hope to promote the coordination of traffic and urban development by formulating and improving traffic development strategies. The effectiveness of these solutions depends largely on an accurate assessment of the distribution of urban hotspots (centers of traffic activity). In recent years, many scholars have employed the K-Means clustering technique to identify urban hotspots, believing it to be efficient. K-means clustering is a sort of iterative clustering analysis. When the data dimensionality is large and the sample size is enormous, the K-Means clustering algorithm is sensitive to the initial clustering centers. To mitigate the problem, a hybrid heuristic "fuzzy system-particle swarm-genetic" algorithm, named FPSO-GAK, is employed to obtain better initial clustering centers for the K-Means clustering algorithm. The clustering results are evaluated and analyzed using three-cluster evaluation indexes (SC, SP and SSE) and two-cluster similarity indexes (CI and CSI). A taxi GPS dataset and a multi-source dataset were employed to test and validate the effectiveness of the proposed algorithm in comparison to the Random Swap clustering algorithm (RS), Genetic K-means algorithm (GAK), Particle Swarm Optimization (PSO) based K-Means, PSO based constraint K-Means, PSO based Weighted K-Means, PSO-GA based K-Means and K-Means++ algorithms. The comparison findings demonstrate that the proposed algorithm can achieve better clustering results, as well as successfully acquire urban hotspots.
更多查看译文
关键词
urban hotspots,K-means clustering,genetic algorithm,fuzzy system,particle swarm optimization,taxi GPS data
AI 理解论文
溯源树
样例
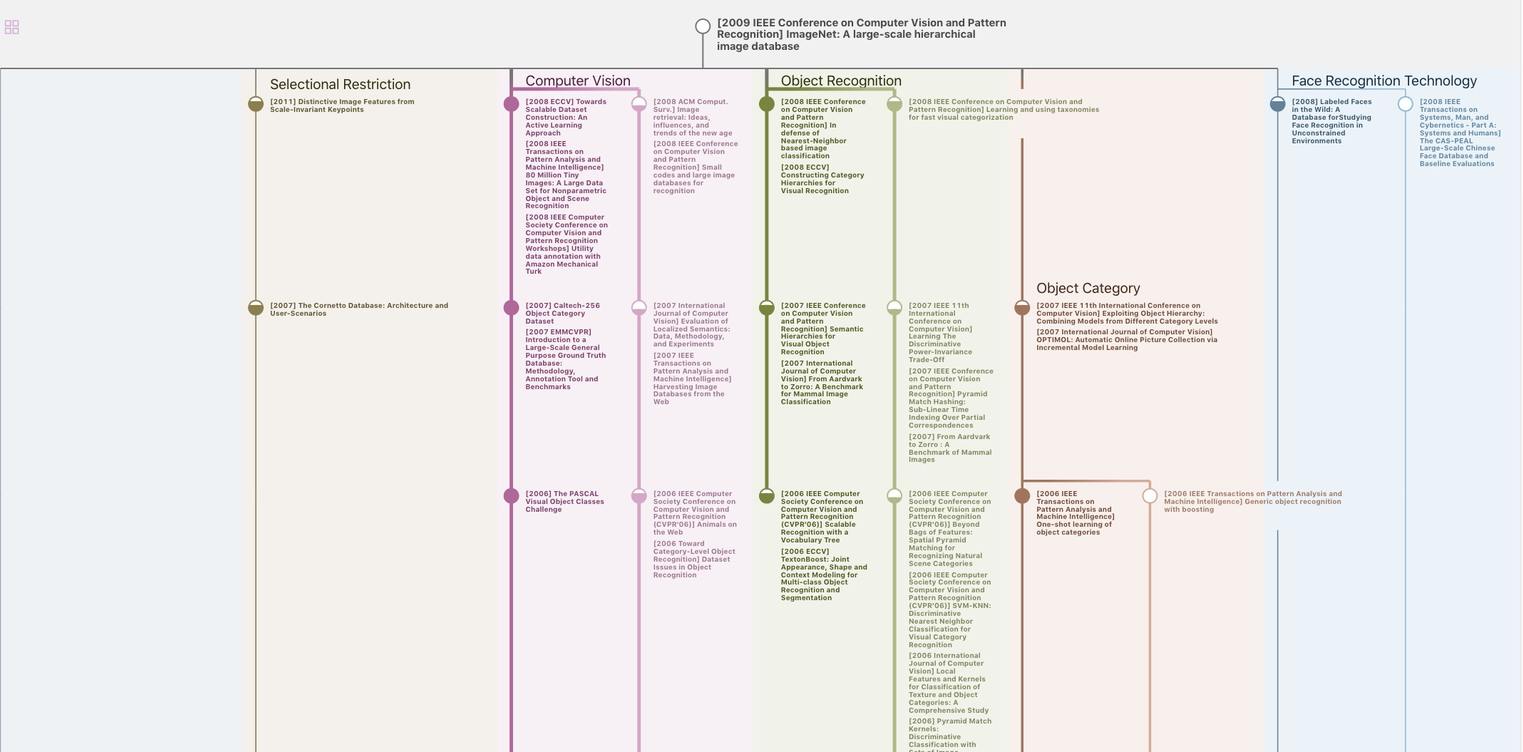
生成溯源树,研究论文发展脉络
Chat Paper
正在生成论文摘要