AdaSG: A Lightweight Feature Point Matching Method Using Adaptive Descriptor with GNN for VSLAM
SENSORS(2022)
摘要
Feature point matching is a key component in visual simultaneous localization and mapping (VSLAM). Recently, the neural network has been employed in the feature point matching to improve matching performance. Among the state-of-the-art feature point matching methods, the SuperGlue is one of the top methods and ranked the first in the CVPR 2020 workshop on image matching. However, this method utilizes graph neural network (GNN), resulting in large computational complexity, which makes it unsuitable for resource-constrained devices, such as robots and mobile phones. In this work, we propose a lightweight feature point matching method based on the SuperGlue (named as AdaSG). Compared to the SuperGlue, the AdaSG adaptively adjusts its operating architecture according to the similarity of input image pair to reduce the computational complexity while achieving high matching performance. The proposed method has been evaluated through the commonly used datasets, including indoor and outdoor environments. Compared with several state-of-the-art feature point matching methods, the proposed method achieves significantly less runtime (up to 43x for indoor and up to 6x for outdoor) with similar or better matching performance. It is suitable for feature point matching in resource constrained devices.
更多查看译文
关键词
feature point matching, GNN, VSLAM
AI 理解论文
溯源树
样例
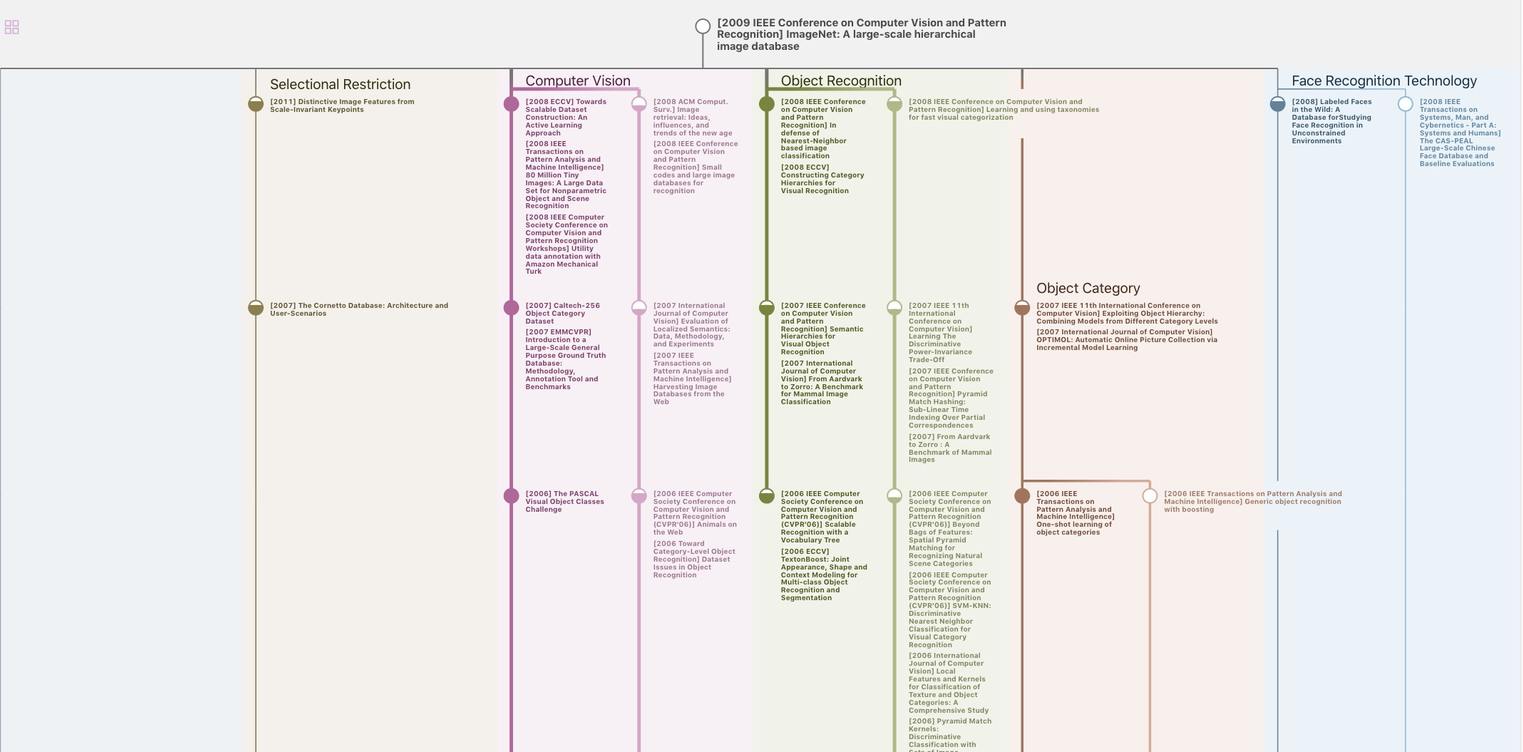
生成溯源树,研究论文发展脉络
Chat Paper
正在生成论文摘要