A preliminary implementation of convolutional neural network in embedded system for road pavement quality classification
REAL-TIME IMAGE PROCESSING AND DEEP LEARNING 2022(2022)
摘要
This paper proposes an embedded implementation able to evaluate the pavement quality of road infrastructure by using a low-cost microcontroller board, an analog microphone placed inside the tyre cavity and a Convolutional Neural Network for real-time classification. To train the neural network, tracks audio were collected employing a vehicle moving at different cruise speeds (30, 40, 50 km/h) in the area of Pisa. The raw audio signals were split, labelled and converted into images by calculating the MFCC spectrogram. Finally, the author designed a tiny CNN with a size of 18KB able to classify five different classes: good quality road, bad quality road, pothole-bad road, silence and unknown. The CNN model achieved an accuracy equal to 93.8 % on the original model and about 90 % on the quantized model. The finale embedded system is equipped with BLE communication for the transmission of information to a smartphone equipped with GPS and obtain real-time maps of road quality.
更多查看译文
关键词
road surface classification, convolutional neural network, audio processing, embedded system, image recognition
AI 理解论文
溯源树
样例
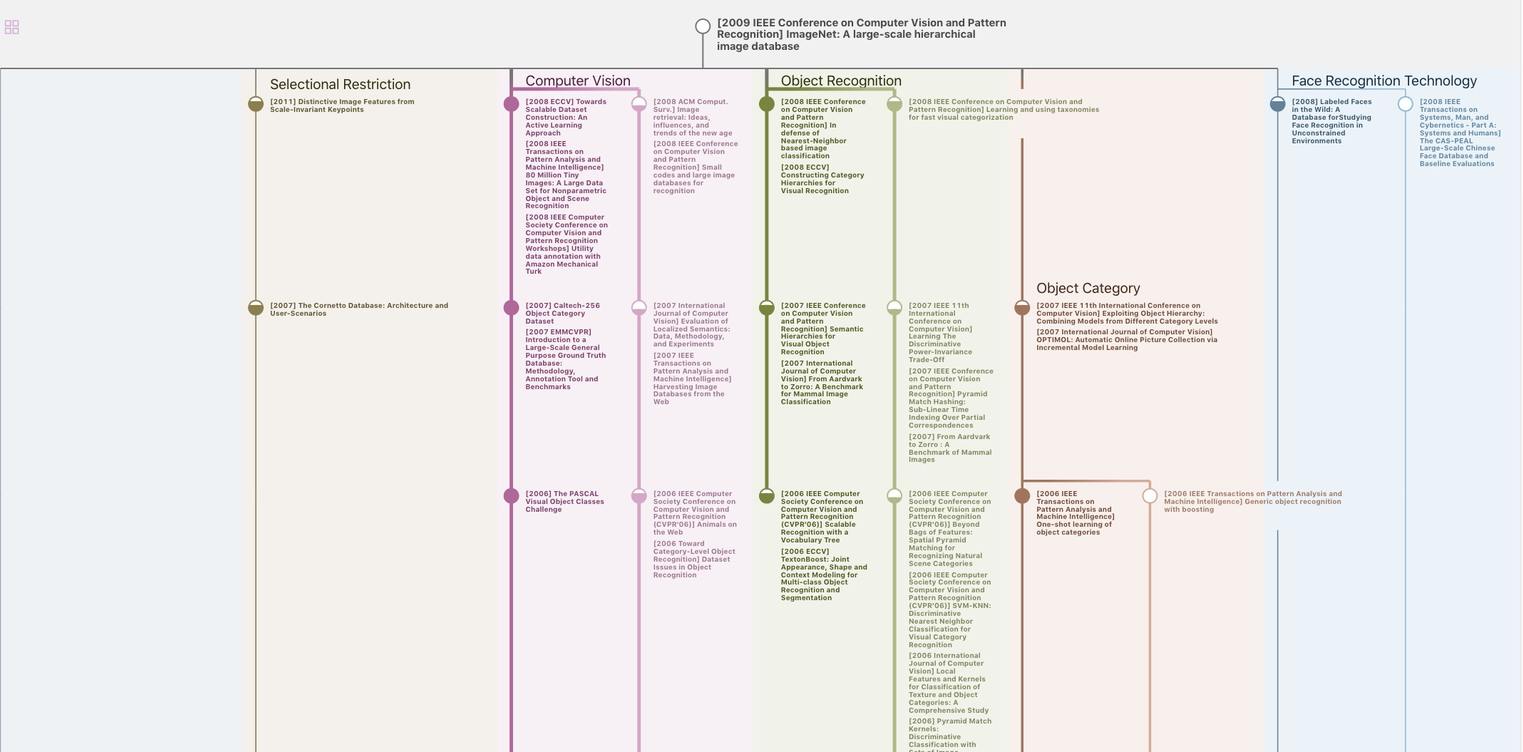
生成溯源树,研究论文发展脉络
Chat Paper
正在生成论文摘要