Investigating the effects of ensemble and weight optimization approaches on neural networks' performance to estimate the dynamic modulus of asphalt concrete
ROAD MATERIALS AND PAVEMENT DESIGN(2023)
摘要
This study hybridized the ensemble and weight optimization approaches with an artificial neural network (ANN) algorithm to forecast the dynamic modulus (E*) of asphalt concrete. For input selection, this study employed the random forest technique and tested various techniques, including evolutionary, backward, forward, and brute force, to be hybridized with random forest. The evolutionary-random forest technique was used as the best input-selection method. The rho 200, V-beff, binder G* (dynamic shear modulus), and binder phi (phase angle) were selected as the most important variables for developing the ANN models. The findings of this research indicated that the artificial neural network-particle swarm optimisation (ANNPSO) model performed better than the other models. Also, the weight optimization techniques were more efficient than the ensemble techniques to improve the predictive power of ANN to forecast the dynamic modulus of asphalt concrete.
更多查看译文
关键词
Dynamic modulus,neural network,ensemble approach,weight optimization approach,prediction
AI 理解论文
溯源树
样例
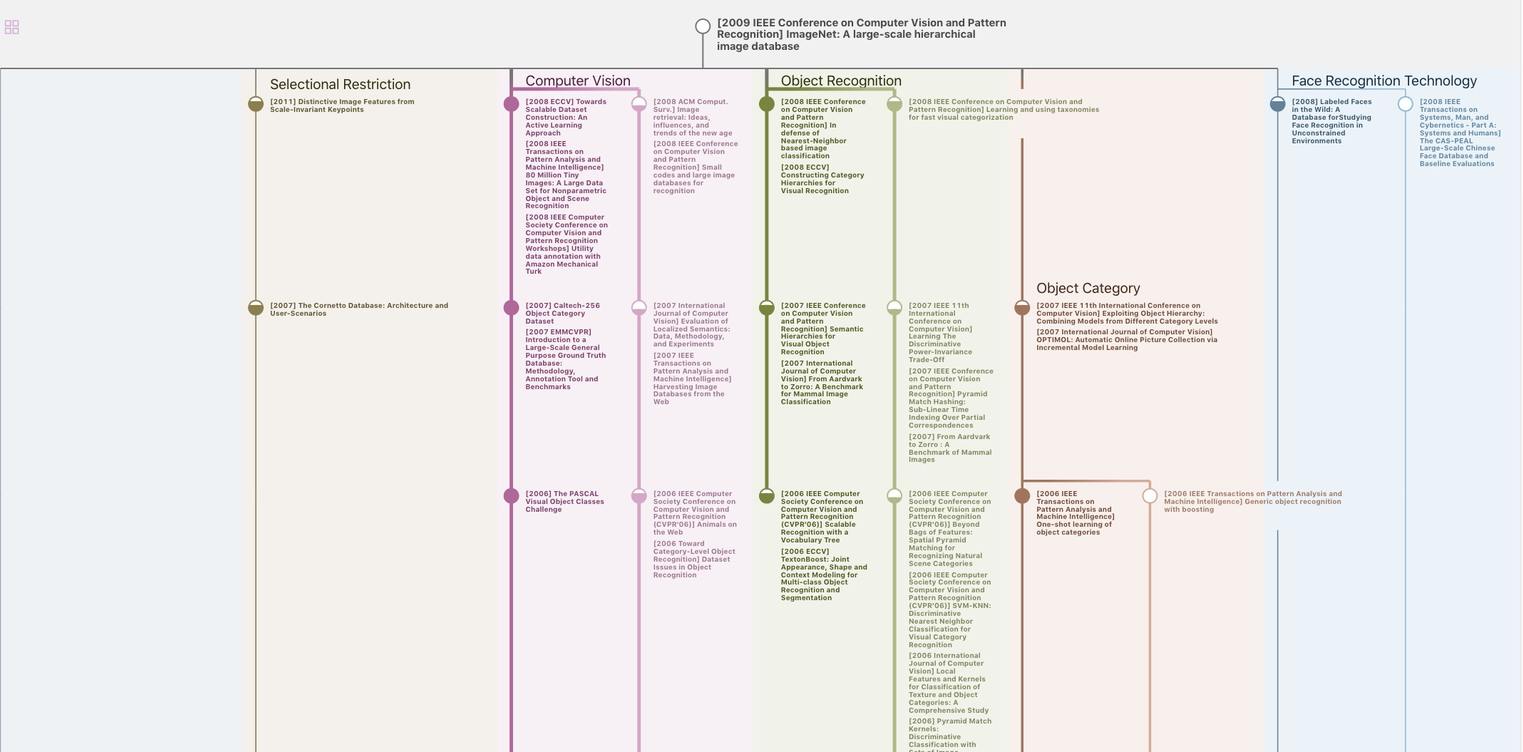
生成溯源树,研究论文发展脉络
Chat Paper
正在生成论文摘要